Primal-Dual Differentiable Programming for Distribution System Critical Load Restoration
2023 IEEE Power & Energy Society General Meeting (PESGM)(2023)
摘要
Swift and reliable critical load restoration (CLR) can help make a distribution system resilient towards extreme events. To optimally achieve that, alongside practical concerns such as limiting online computational burden, some studies leverage model-free reinforcement learning (RL) to train control policies. Despite the advantages provided by RL algorithms, these approaches suffer from two issues: 1) the lack of a proper mechanism for constraint enforcement, and 2) poor sample efficiency. Therefore, in this paper, a primal-dual differentiable programming (PDDP) method is developed for guiding the training leading to a constraint-satisfying policy. Additionally, the model-based nature of the proposed method aims at improving sample efficiency. The experiment on a CLR problem demonstrates that PDDP can effectively train a control policy that both achieves desirable performance and satisfies required constraints.
更多查看译文
关键词
grid resilience, load restoration, differentiable programming, primal-dual method, reinforcement learning
AI 理解论文
溯源树
样例
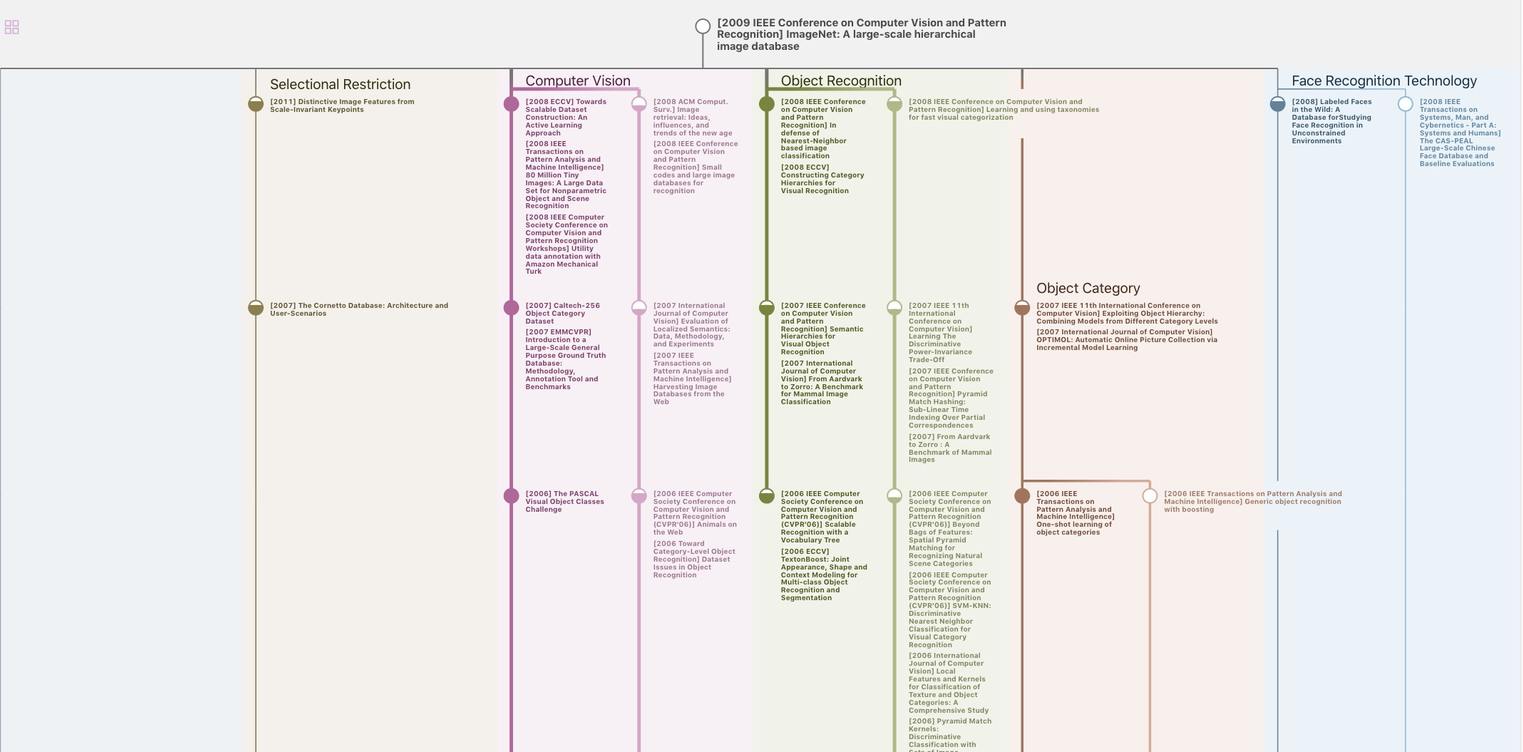
生成溯源树,研究论文发展脉络
Chat Paper
正在生成论文摘要