Towards Distributed Learning of PMU Data: A Federated Learning based Event Classification Approach
2023 IEEE Power & Energy Society General Meeting (PESGM)(2023)
摘要
This paper studies distributed learning of real-world phasor measurement unit (PMU) data to enable privacy-preserving data sharing and analytics among different entities (i.e., PMU data owners). As real-world PMU data are collected/owned by different entities, they may not share their data due to privacy/security concerns. To tackle this challenge, this paper develops a federated learning based event classification approach that enables multiple entities to collaboratively train a good event classifier while keeping the PMU data decentralized and private. As each entity may have different numbers of PMUs, the inputs from different entities to the event classification model can be different. To address this challenge, a federated XGBoost model is proposed, in which the same set of event features can be constructed by each entity with different numbers of PMUs. To enhance the privacy of the federated XGBoost model, the communication during the training process is encrypted using additive homomorphic encryption scheme, which eliminates the requirement of a trusted server. Numerical experiments using the real-world PMU dataset show that the proposed federated XGBoost model achieves almost the same performance as the centralized counterparts and is robust against different data distributions of entities.
更多查看译文
关键词
Phasor Measurement Units (PMUs),Event Classification,Federated Learning
AI 理解论文
溯源树
样例
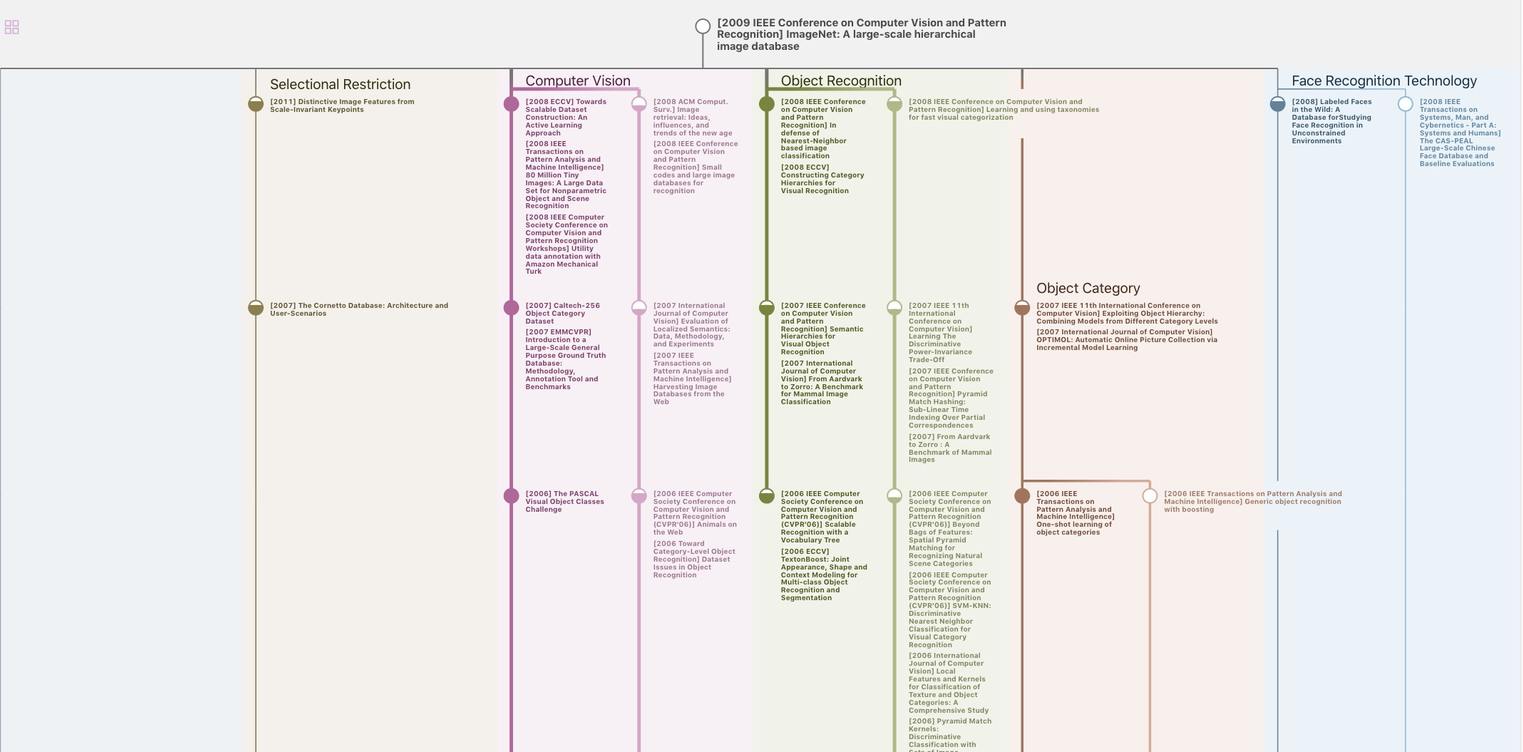
生成溯源树,研究论文发展脉络
Chat Paper
正在生成论文摘要