A Machine Learning-based Short-term Load Forecasting Method for Behind-the-meter DERs
2023 IEEE Power & Energy Society General Meeting (PESGM)(2023)
摘要
Due to recent advancements in the power industry, residential sites could provide part of or even their entire energy using on-site generation. However, because their equipment might consume or generate energy without going through a meter, the data recording and monitoring could be challenging for electric utilities. A more complex distribution system is the result of this issue, and utilities cannot detect the events between 15-minute intervals, resulting in an inaccurate forecasting process. This paper presents a technique based on a two-layer long short-term memory (LSTM) framework to forecast the load profile of the residents regarding the different combinations of distributed energy resources (DERs). The data for training the model is considered every minute instead of conventional 15-minute intervals, so it can make a more accurate forecasting process and preserve households’ privacy better.
更多查看译文
关键词
Behind-the-meter, Distributed energy resources, Energy consumption, Load forecasting, Long short-term memory
AI 理解论文
溯源树
样例
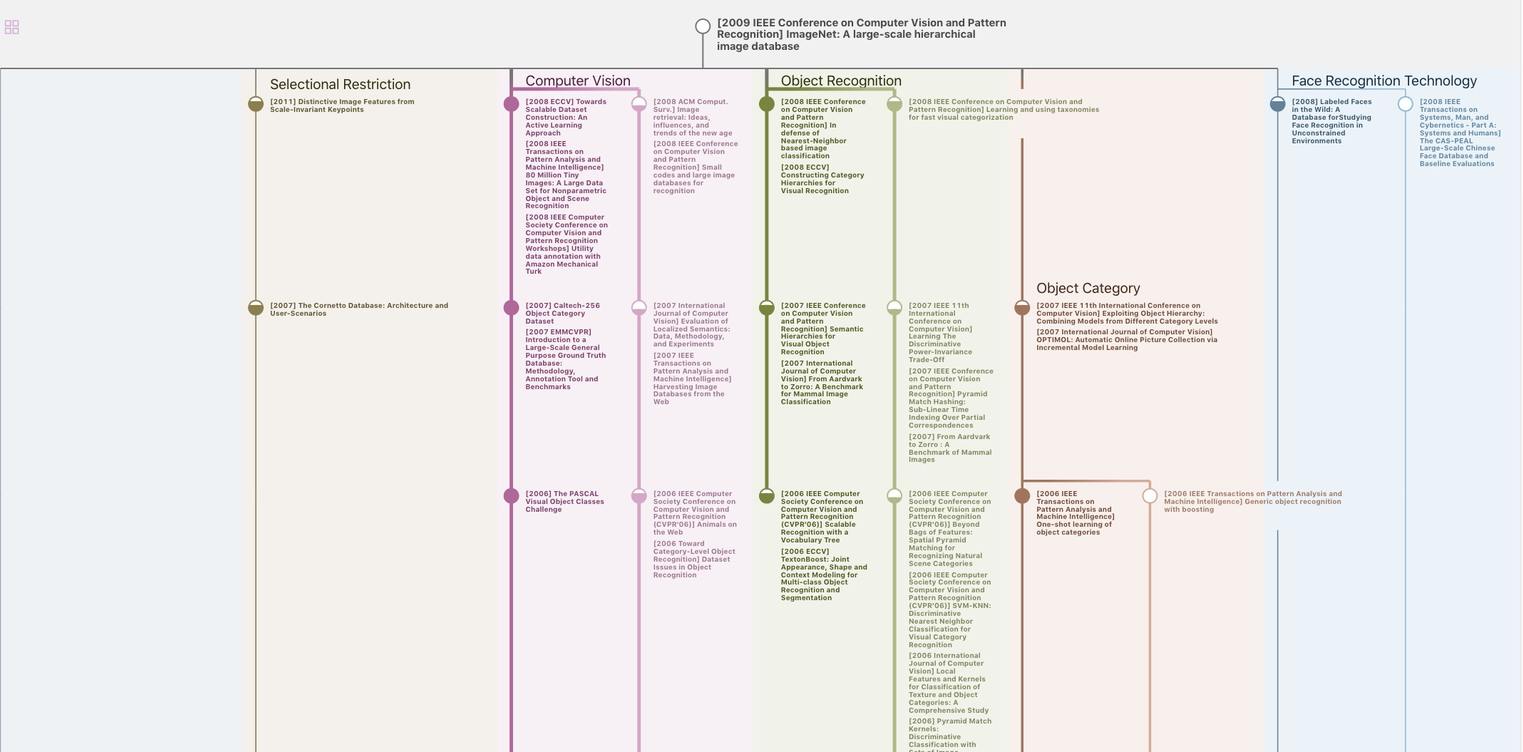
生成溯源树,研究论文发展脉络
Chat Paper
正在生成论文摘要