Electricity Anomaly Detection with Self-Supervised Time Convolutional Networks
2023 3rd International Conference on Electrical Engineering and Mechatronics Technology (ICEEMT)(2023)
摘要
Traditional detection methods for abnormal electricity usage behavior in power grids are inefficient, and machine learning models have limitations in generalization ability when dealing with large amounts of data. Considering the problem of imbalanced samples in the dataset of abnormal electricity usage, this paper proposes a self-supervised learning-based electricity abnormality detection model based on time convolutional networks. A self-supervised pretext task based on masked reconstruction is used to train an encoder-decoder network. The trained encoder network is used to extract the features of electricity usage data itself. A classifier trained on the extracted feature vectors with manually labeled is used to obtain the final abnormality detection result. Experiments on an electricity meter dataset collected by the State Grid Corporation of China show that the proposed method is more effective than existing Support Vector Machine, Recurrent Neural Network, and Convolutional Neural Networks.
更多查看译文
关键词
Electricity,Anomaly Detection,Self-Supervised Learning,Time Convolutional Networks
AI 理解论文
溯源树
样例
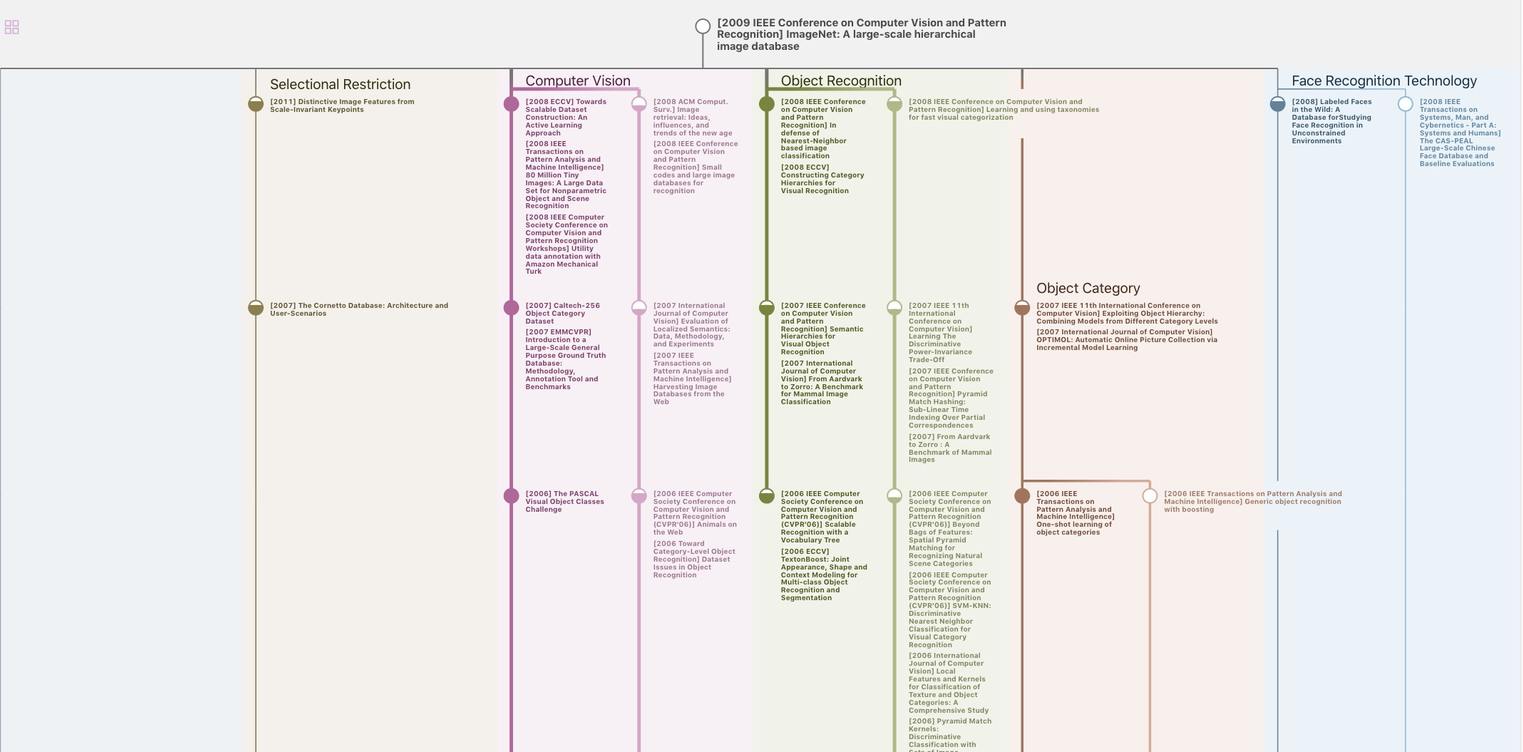
生成溯源树,研究论文发展脉络
Chat Paper
正在生成论文摘要