Attention Augmented ConvLSTM for Environment Prediction
2021 IEEE/RSJ International Conference on Intelligent Robots and Systems (IROS)(2021)
摘要
Safe and proactive planning in robotic systems generally requires accurate predictions of the environment. Prior work on environment prediction applied video frame prediction techniques to bird’s-eye view environment representations, such as occupancy grids. ConvLSTM-based frameworks used previously often result in significant blurring of the predictions, loss of static environment structure, and vanishing of moving objects, thus hindering their applicability for use in safety-critical applications. In this work, we propose two extensions to the ConvLSTM architecture to address these issues. We present the Temporal Attention Augmented ConvLSTM (TAAConvLSTM) and Self-Attention Augmented ConvLSTM (SAAConvLSTM) frameworks for spatiotemporal occupancy grid prediction, and demonstrate improved performance over baseline architectures on the real-world KITTI and Waymo datasets. We provide our implementation at https: //github.com/sisl/AttentionAugmentedConvLSTM.
更多查看译文
关键词
proactive planning,robotic systems,environment prediction,ConvLSTM architecture,spatiotemporal occupancy grid prediction,temporal attention augmented ConvLSTM,TAAConvLSTM,self-attention augmented ConvLSTM,SAAConvLSTM
AI 理解论文
溯源树
样例
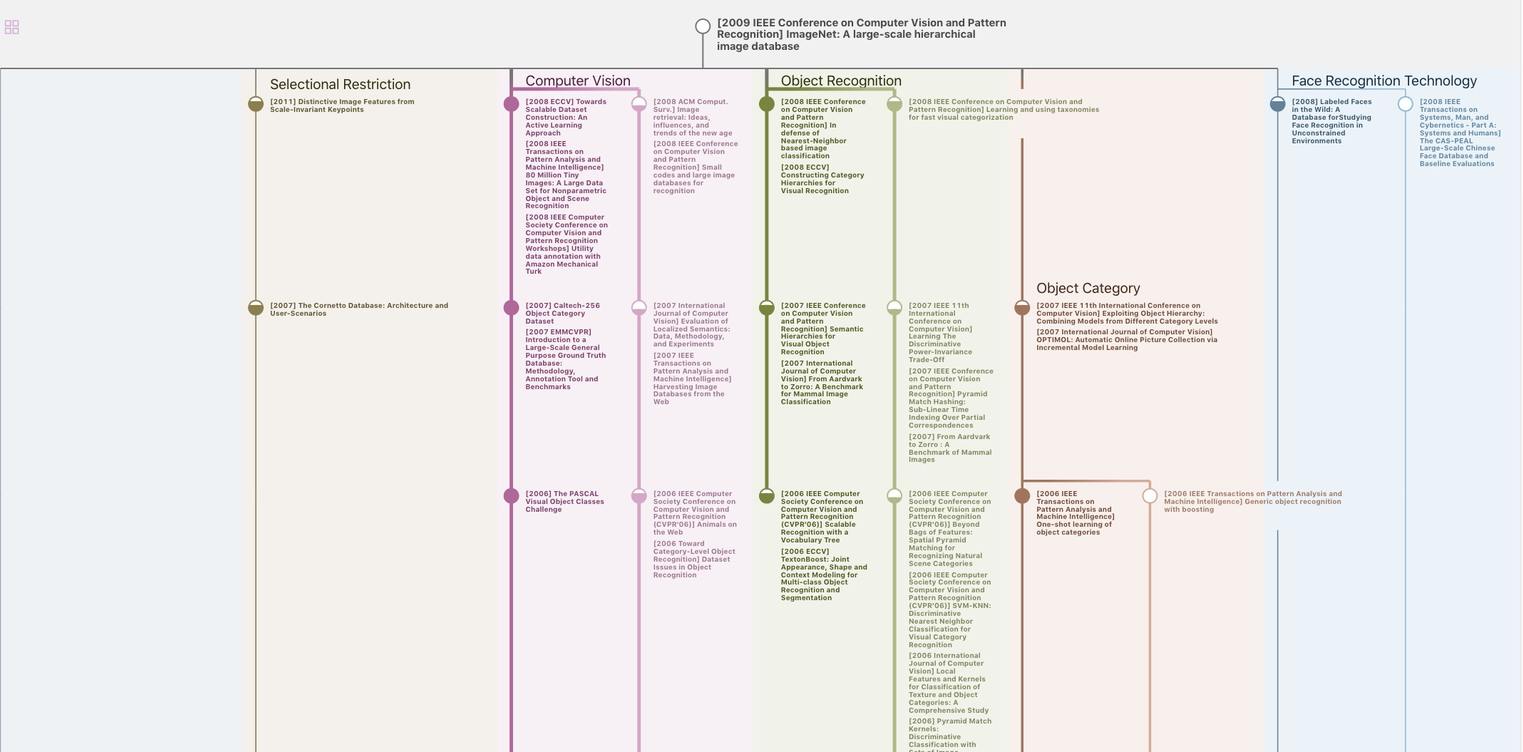
生成溯源树,研究论文发展脉络
Chat Paper
正在生成论文摘要