Improving Particle Filters with Adaptive Bayesian Resampling for Real-Time Filtering
2023 8th International Conference on Signal and Image Processing (ICSIP)(2023)
摘要
Particle filters (PFs) are a set of simulation-based methods, which recursively estimate the posterior densities by a set of weighted samples. Due to their sample-based representation, PFs are well suited to estimate the state of non-linear dynamic systems. The increased representational power of PFs, however, comes at the cost of higher computational complexity. Thus, it has been challenging to apply PFs in real-time applications such as target tracking. In this paper, we propose the design of PFs with two novel Bayesian resampling methods which are well suited for parallel execution. The resampling algorithms are further improved for speed consideration to allow for real-time filtering. We then propose the design of PFs with adaptive resampling performed during the filtering, in order to increase the estimation accuracy as well as the speed. The proposed method is evaluated with a well-known tracking problem. Experimental results confirm that PFs with the proposed resampling algorithms achieve similar localization accuracy compared to the traditional resampling methods, while improving the speed considerably. PFs with the adaptive resampling by the computation of effective sample size (ESS) can further improve the accuracy, up to 12% in comparison with traditional filtering.
更多查看译文
关键词
Particle Filter,Resampling,Real-time Filtering,Bayesian Resampling,Adaptive Resampling
AI 理解论文
溯源树
样例
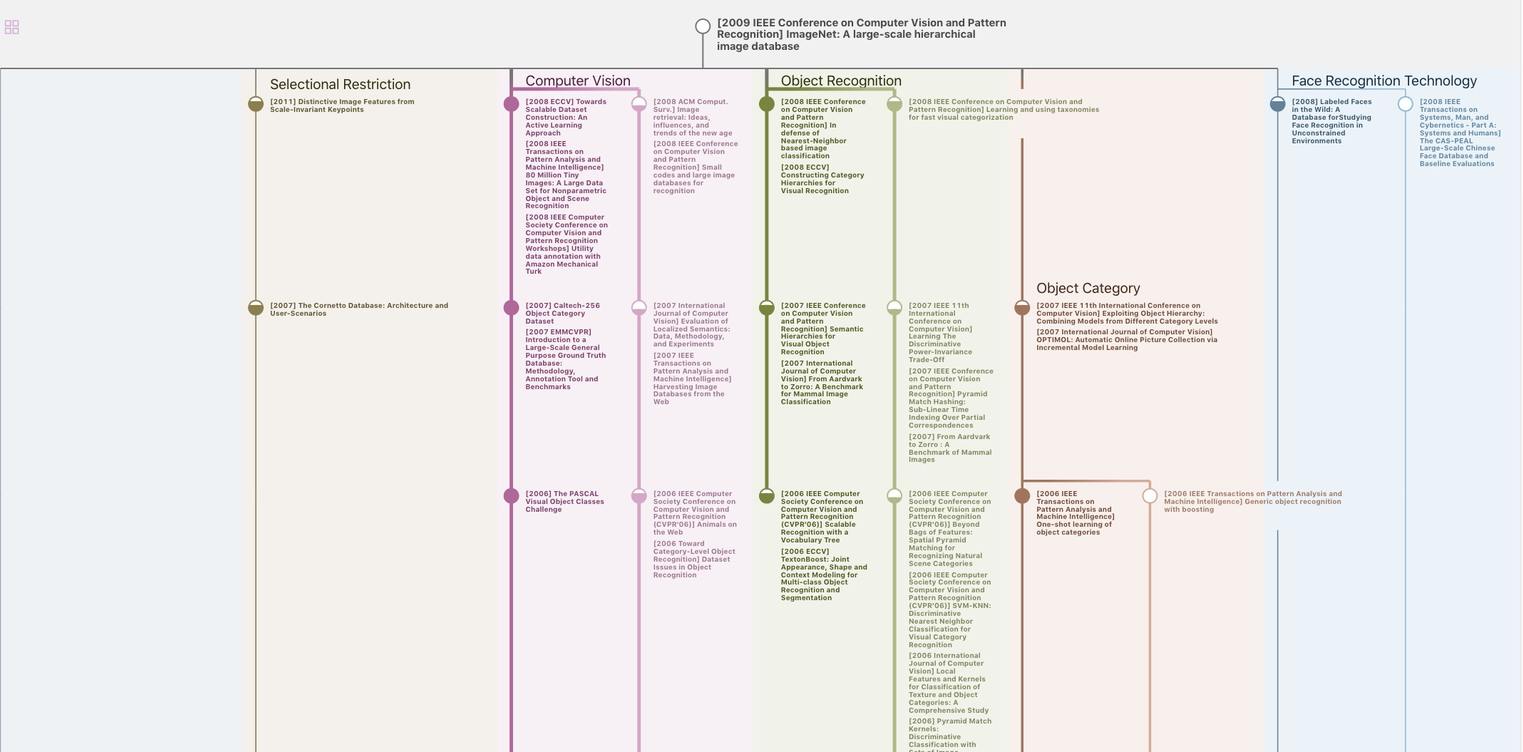
生成溯源树,研究论文发展脉络
Chat Paper
正在生成论文摘要