Semi-supervised Robust Dual-graph Concept Factorization via L2,1 Norm
2021 China Automation Congress (CAC)(2021)
摘要
Recently, as an effective paradigm for representation learning, Concept Factorization (CF) has attracted a great deal of interests in the areas of machine learning and data mining. However, it is difficult for existing CF related algorithms to effectively learn data manifold and feature manifold while utilizing the label information of data to learn more discriminative features. To overcome this problem, we propose a novel method called semi-supervised robust dual-graph CF (named RGCCF), which considers the manifold of data and features and combines the limited label information. Furthermore, an effective optimized method is proposed to solve the RGCCF problem. The experimential results on real-world datasets shows that RGCCF can achieve better clustering performance compared with the other CF-based methods.
更多查看译文
关键词
Semi-supervised,clustering,concept factorization,dual-graph regularized
AI 理解论文
溯源树
样例
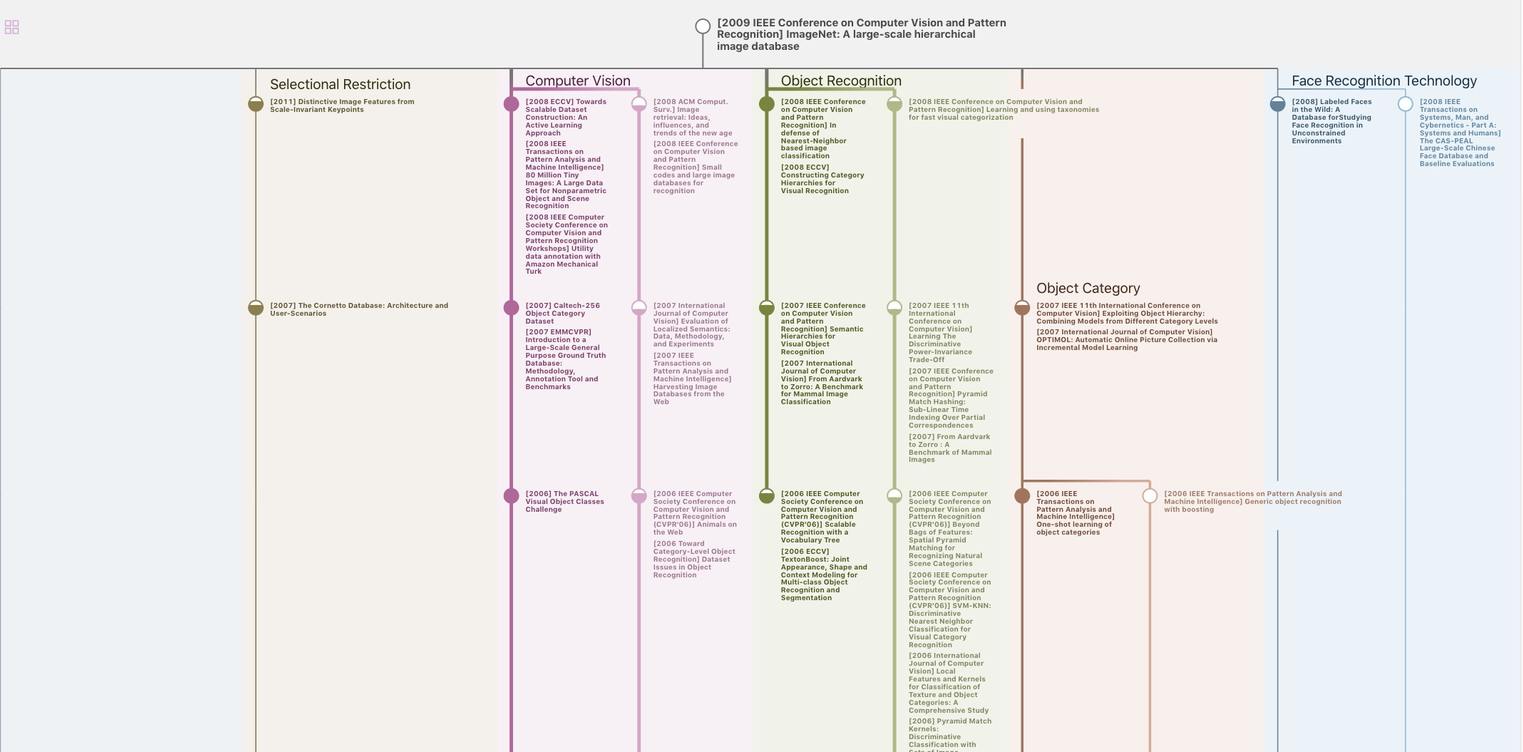
生成溯源树,研究论文发展脉络
Chat Paper
正在生成论文摘要