Impact of Pruning and Quantization: A Light Weight Multi-Sensor Pothole Detection System
2023 11th International Symposium on Electronic Systems Devices and Computing (ESDC)(2023)
摘要
Pothole Detection has been a part of Advanced Driver Assistant Systems (ADAS) for a long time. To detect potholes, many techniques have been used. Deep learning-based methods have been particularly successful in this regard. However, the localization of the potholes accurately may not be possible only by having one modality of information. This work explores the multi-sensor information fusion (from Accelerometer and Gyroscope) to detect the potholes. Notably, most of the existing works proposed to make use of models such as Convolution Neural Networks, and other Attention models like Long Short-Term Memory (LSTM)’s, Gated Recurrent Units (GRUs), and Transformers. Despite having such proven architectures for complex and non-linear learning representations with attention units, still, the challenge of real-time deployments with optimized computing devices remains unaddressed. With the proposed approach, efficient deployments are possible on edge devices embedded in the vehicle providing a reliable ADAS solution for improved driver safety. The investigations and ablation study from the proposal focus on two-fold addressing the trade-off between model size and test accuracy. Significantly, the proposed hybrid architecture, the INN-former with quantization, achieved a size reduction by 16.12%, not compromising much with the maximum test accuracy reported at 96.12%. Similarly, pruning achieves a 1.115% size reduction with a minimal difference in test accuracy of 85.43% for the INN-former, and a 3.76% decrease in size while only a 4.95% decrease in test accuracy reported as 95.43% with the Attention model making use of GRU/ LSTM. Importantly, the proposed work discusses the design parameters for lightweight architectures investigating the pruning and quantization techniques that are not compromising the generalization capability of the models, which is highly essential for real-time deployments and validation.
更多查看译文
关键词
ADAS,Multi-Sensor Fusion,Optimization,Pruning,Quantization,Transformers
AI 理解论文
溯源树
样例
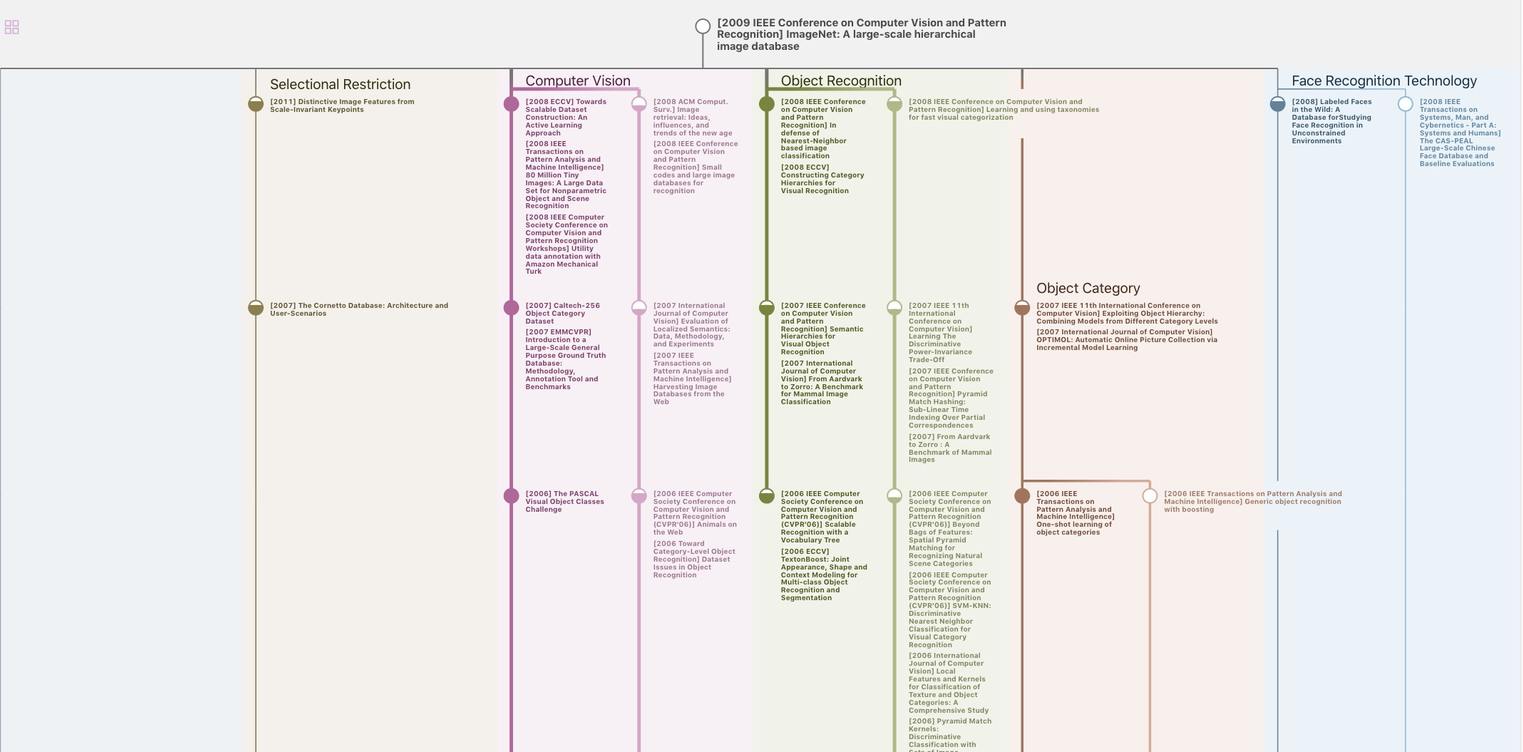
生成溯源树,研究论文发展脉络
Chat Paper
正在生成论文摘要