Bit-Level Quantization for Efficient Layout Hotspot Detection
2023 International Symposium of Electronics Design Automation (ISEDA)(2023)
摘要
Hotspot detection is an essential step in the physical verification flow to identify layout patterns that are sensitive to process variations. Recent advances in machine learning have enabled deep neural networks (DNNs) to achieve good performance for hotspot detection; however, these models require a high computational complexity and memory footprint. To reduce such costs, quantization provides a promising solution by compressing DNNs into low-bit inference schemes. In this paper, we propose several quantization algorithms specifically designed for a classic neural network based hotspot detector while taking into account the feature distribution of the dataset used. Our experiments show that our compressed model can achieve competitive results compared with full-precision models while significantly reducing inference runtime at the same time.
更多查看译文
关键词
Hotspot Detection,Quantization,Layout
AI 理解论文
溯源树
样例
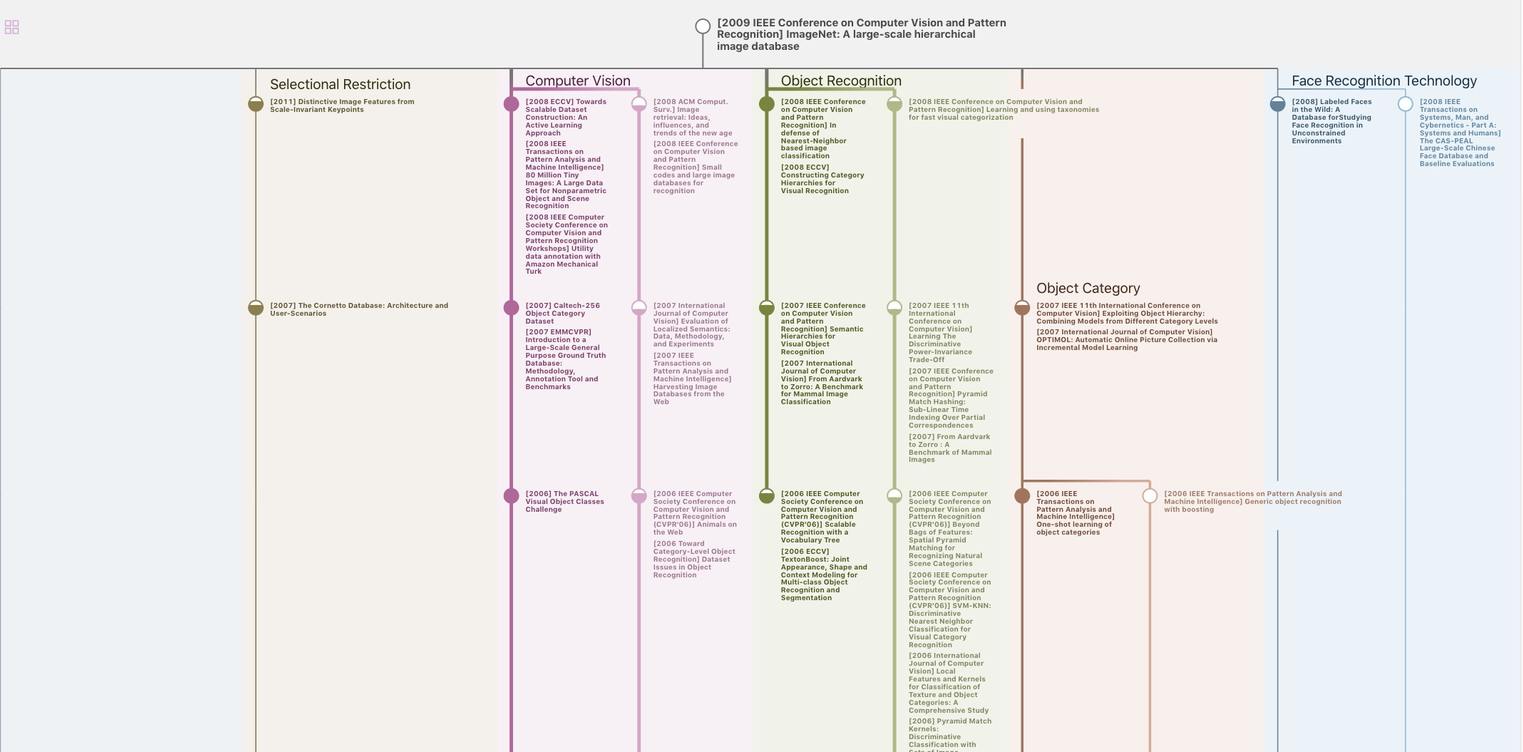
生成溯源树,研究论文发展脉络
Chat Paper
正在生成论文摘要