Feature-Based Machine Learning for Predicting Resistances in Printed Electronics
2023 IEEE International Conference on Flexible and Printable Sensors and Systems (FLEPS)(2023)
摘要
Making repeatable devices is a significant obstacle to the widespread adoption of printed electronics. Due to the complexity and sensitivity of inkjet or extrusion printing physics, it is difficult to use simple models to make predictions that match measured devices. To overcome this challenge, we developed an AI-based approach to predict experimental values. We employed two different printers to create a test pattern with various controllable parameters, and an automated prober was developed to measure wire resistances across a large printed area. Image processing was used on scanned PCB images to extract texture data from the printed structures. A machine learning model was developed to predict the test structures' resistance from only their geometric parameters and texture data. In our best single-board experiments, the root mean square error between the predicted and measured values was within
$0.01 \Omega$
. This model can be used to design structures that achieve desired resistances by actively controlling the print texture.
更多查看译文
关键词
Printed Electronics (PE),Virtual Metrology,Current-Voltage Measurement (IV),Machine Learning
AI 理解论文
溯源树
样例
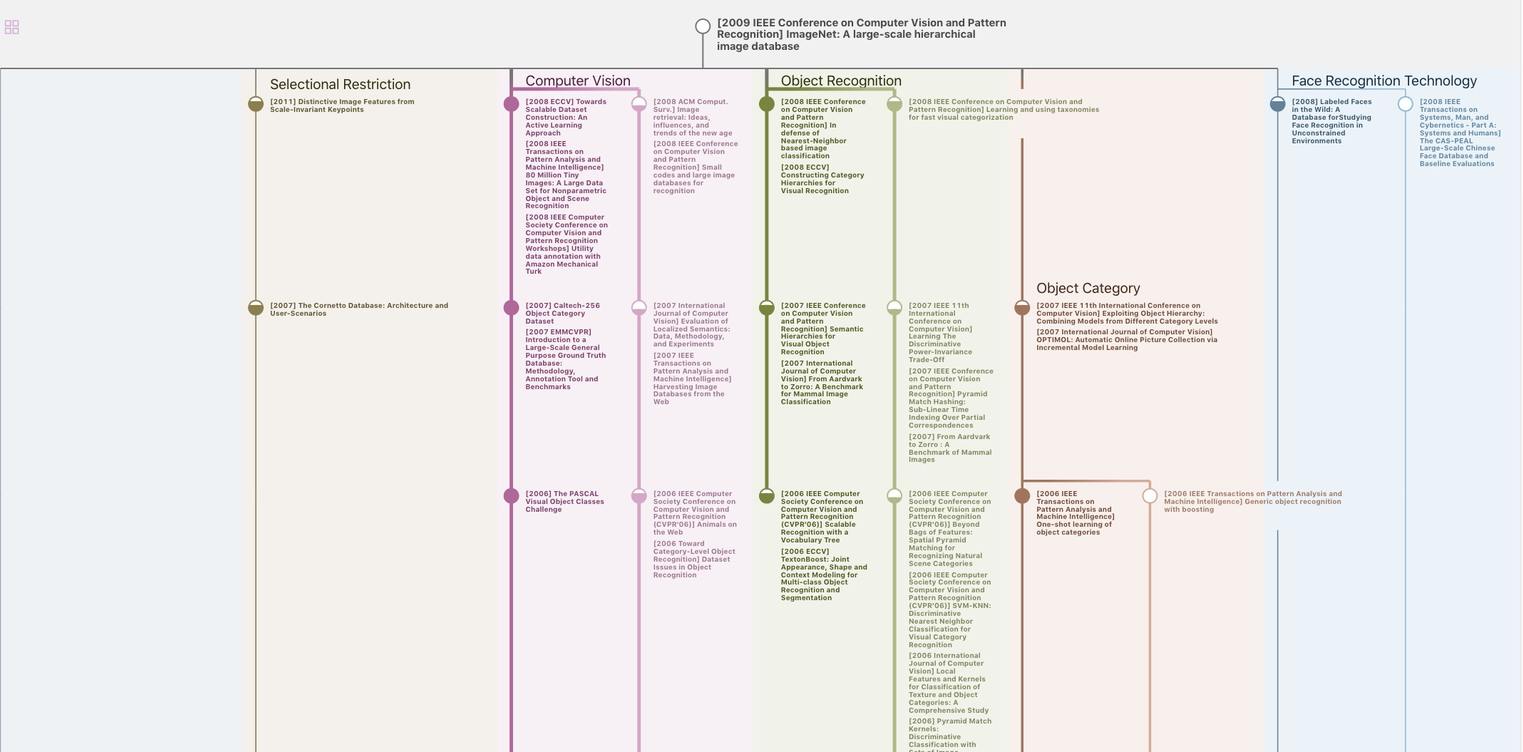
生成溯源树,研究论文发展脉络
Chat Paper
正在生成论文摘要