A Novel Framework for Online Knowledge Distillation
2022 IEEE 8th International Conference on Computer and Communications (ICCC)(2022)
摘要
Traditional knowledge distillation transfers the capabilities of a large network to a smaller network through a two-stage training. Recent Online knowledge distillation uses aggregated intermediate predictions of multiple peer models as the goal of the peer model. Although this approach can get rid of large teacher models, the use of simple aggregation functions makes the problem of inter-peer homogenization severely, which affects the effectiveness of distillation. In this paper, we propose a novel online knowledge distillation strategy, which resists the homogeneity problem by augmenting the inputs randomly. Specifically, we construct a multi-network model, and intervene in the training process by implementing a set pattern of image enhancements, allowing the network to benefit from different enhancements during the training process, as a way to enrich the diversity among peers. Experimental results on CIFAR-10/CIFAR-100 show the significant improvement on several structures.
更多查看译文
关键词
Deep Learning,Data Augmentation,Knowledge Distillation
AI 理解论文
溯源树
样例
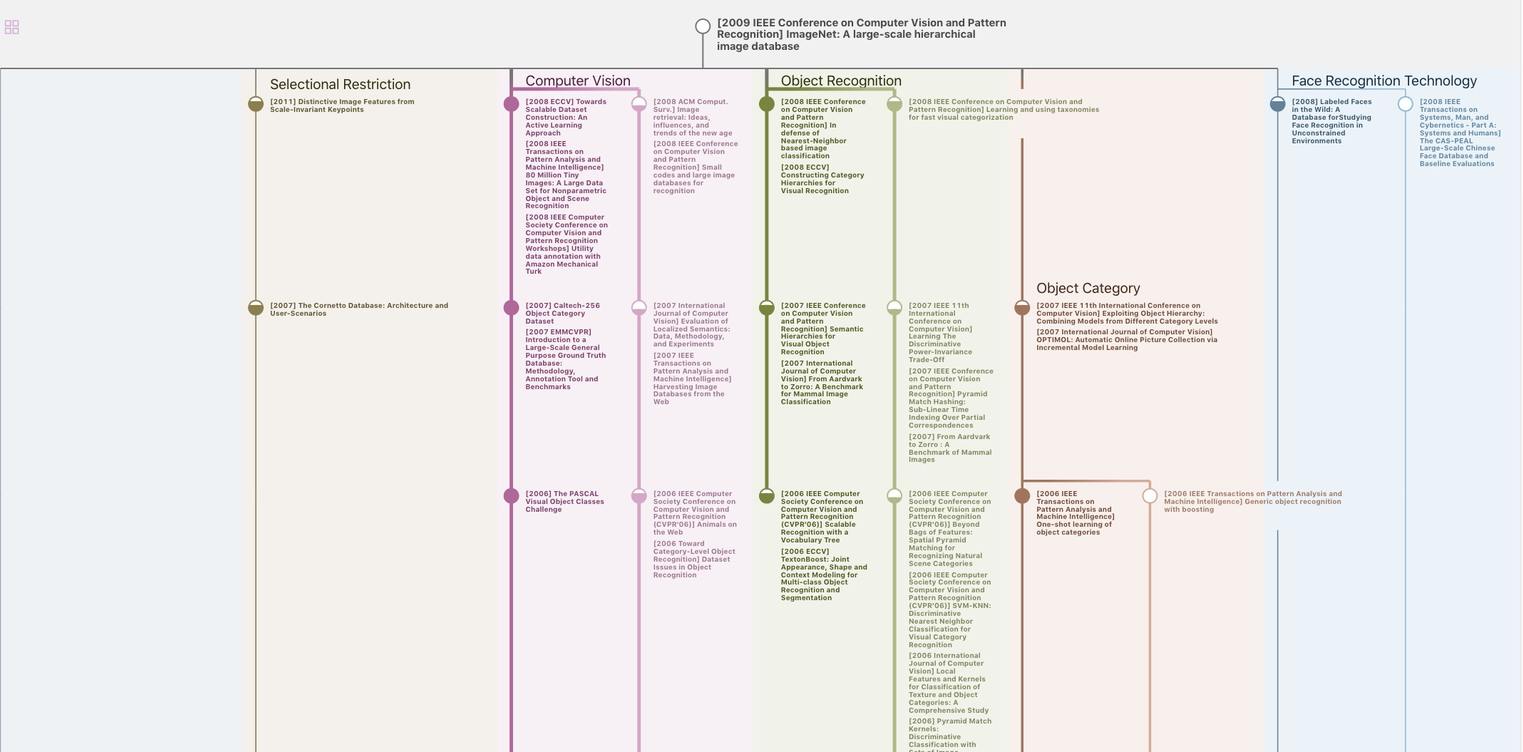
生成溯源树,研究论文发展脉络
Chat Paper
正在生成论文摘要