Application of Deep Learning and Transfer Learning in Continuous Missing Value Imputation of Water Quality Data
2022 IEEE 8th International Conference on Computer and Communications (ICCC)(2022)
摘要
The integrity of water quality data has an important impact on water quality prediction and analysis, so it is necessary to impute the missing values in the data. However, at present, most of the relevant studies on missing value imputation of water quality data focus on a small number of discontinuous missing values, but in many cases, there are a large number of continuous missing values. Therefore, this paper proposes a hybrid model to predict a large number of continuous missing values in the water quality data. The model integrates Bi-LSTM, Self-attention mechanism and transfer learning. The model first calculates the similarity between the sequences with missing values and other complete sequences, then trains the base model BLSA (Bi-LSTM+Self-attention) based on the most similar complete sequences, and finally applies the idea of transfer learning to migrate the model to obtain the model for predicting and filling the missing sequences. In order to verify the effectiveness and practicability of the model, this paper takes the dissolved oxygen concentration of five automatic monitoring stations in Liao River (Liaoning, China) as an example. The results show that the model can effectively predict a large number of continuous missing values in the water quality time series data.
更多查看译文
关键词
deep learning,transfer learning,missing value imputation,water quality data,time series
AI 理解论文
溯源树
样例
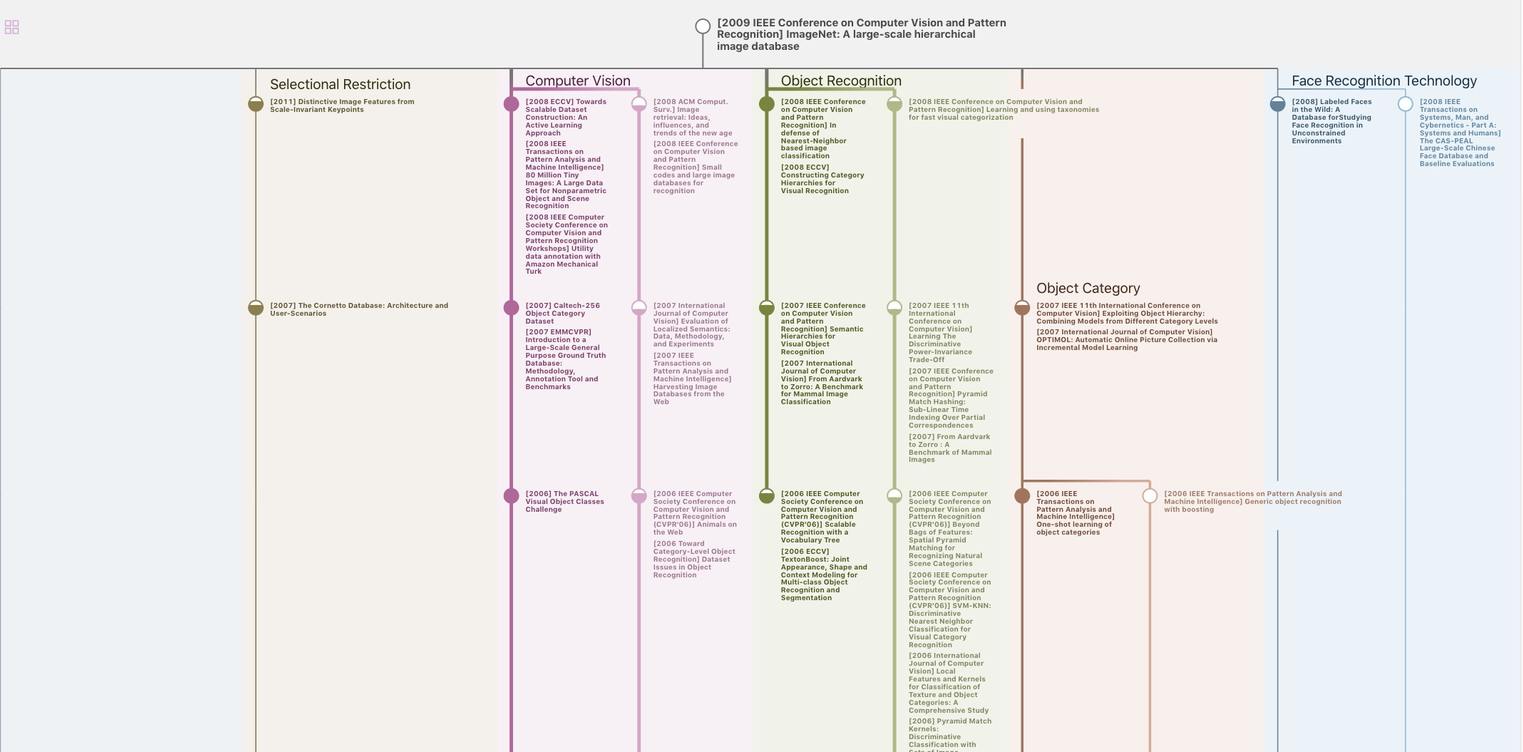
生成溯源树,研究论文发展脉络
Chat Paper
正在生成论文摘要