Long Short-Term Memory for Discharge Estimation in Coastal Neretva River
2023 8th International Conference on Smart and Sustainable Technologies (SpliTech)(2023)
摘要
Estimating discharge in tidal rivers presents a complex challenge, owing to their dynamic and ever-changing nature. These areas are influenced by multiple factors, including tidal range, wind, atmospheric pressure, and freshwater inflows, causing frequent fluctuations in water levels and discharges. As complex flow patterns and strong turbulence are common for tidal rivers, traditional methods of measuring discharge (such as current meters and stream gauges) are considered inefficient and result in unreliable data. Therefore, more adequate methods are required for estimating discharge. The machine learning approach is one of them. The current study employed a Long Short-Term Memory (LSTM) model that was optimized using grid search cross-validation and applied to simulated data of four water stages and a single discharge station in the Neretva River estuary located in Croatia. The study’s results demonstrated the model’s ability to estimate discharge in low and high-flow conditions accurately. A comparison between LSTM and a simple Multiple Linear Regression (MLR) model clearly shows the improvement in LSTM results for every range of discharge values. This study advances the ability to predict and obtain partial control over discharge in a tidal river by offering crucial insights for more effective environmental and flood management strategies.
更多查看译文
关键词
tidal river,machine learning,discharge,water level,long short-term memory,multiple linear regression
AI 理解论文
溯源树
样例
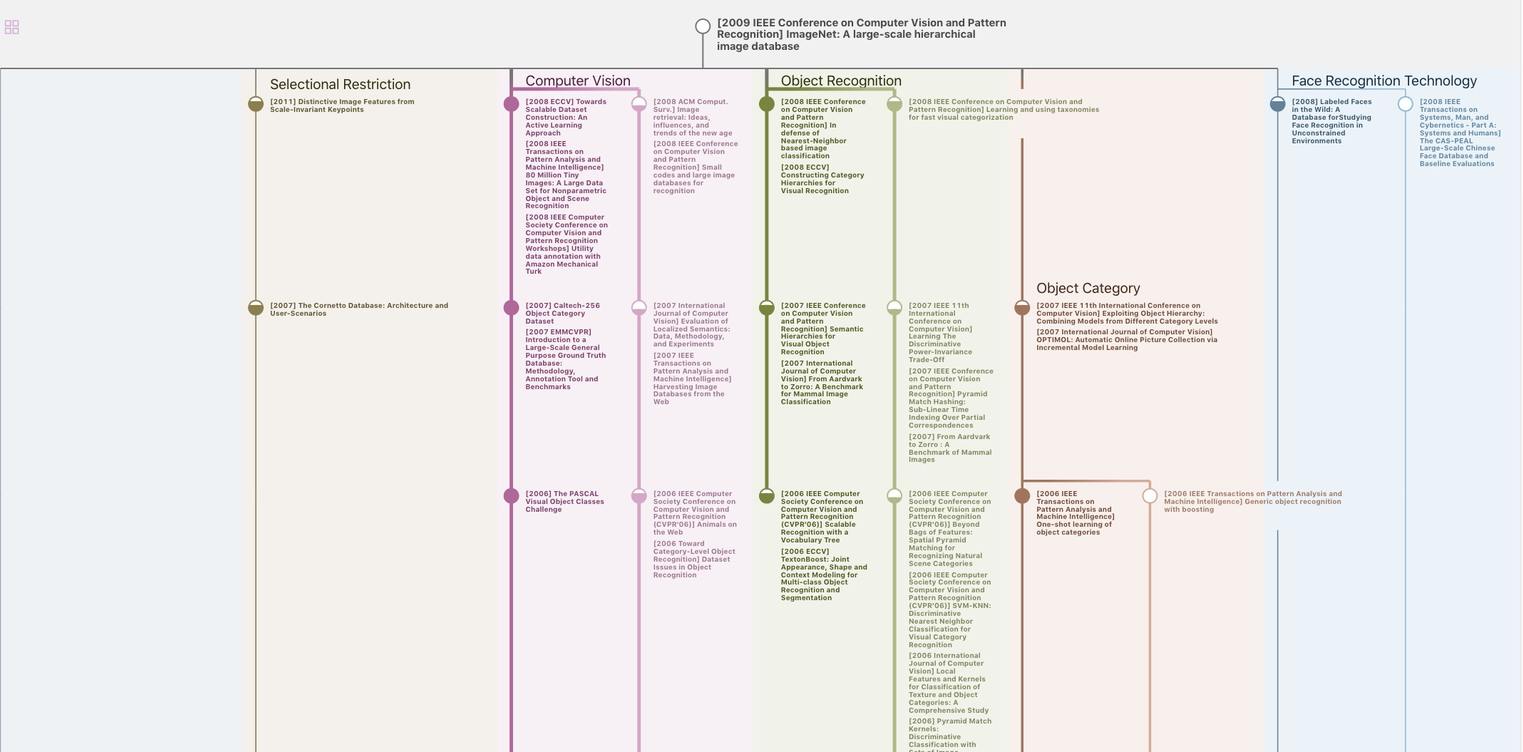
生成溯源树,研究论文发展脉络
Chat Paper
正在生成论文摘要