Research on the Transformer Fault Diagnosis Method Based on LSTM Artificial Neural Network and DGA
2022 International Conference on Intelligent Computing and Machine Learning (2ICML)(2023)
摘要
The concentration of dissolved gas in transformers is closely related to their operating status. Aiming at dissolved gas analysis (DGA) in transformer oil, this paper proposes a fault diagnosis method for transformer DGA based on long-term and short-term memory (LSTM) artificial neural networks. The method uses 240 sets of samples collected by China Southern Power Grid Corporation, with 180 sets as training data and the remaining 60 sets as test data. The input consists of five kinds of dissolved gases in oil, and the output is the corresponding fault type. The hyperparameters (H1=H2=50) of the network are determined through experimentation to establish a transformer DGA fault diagnosis model based on LSTM. The research results indicate that the LSTM diagnosis model has higher consistency with actual fault types compared to the traditional neural network diagnosis model. These findings demonstrate the promising application prospects of LSTM in the field of transformer DGA fault diagnosis.
更多查看译文
关键词
dissolved gases analysis,transformer oil,long-term and short-term memory artificial neural network,fault diagnosis
AI 理解论文
溯源树
样例
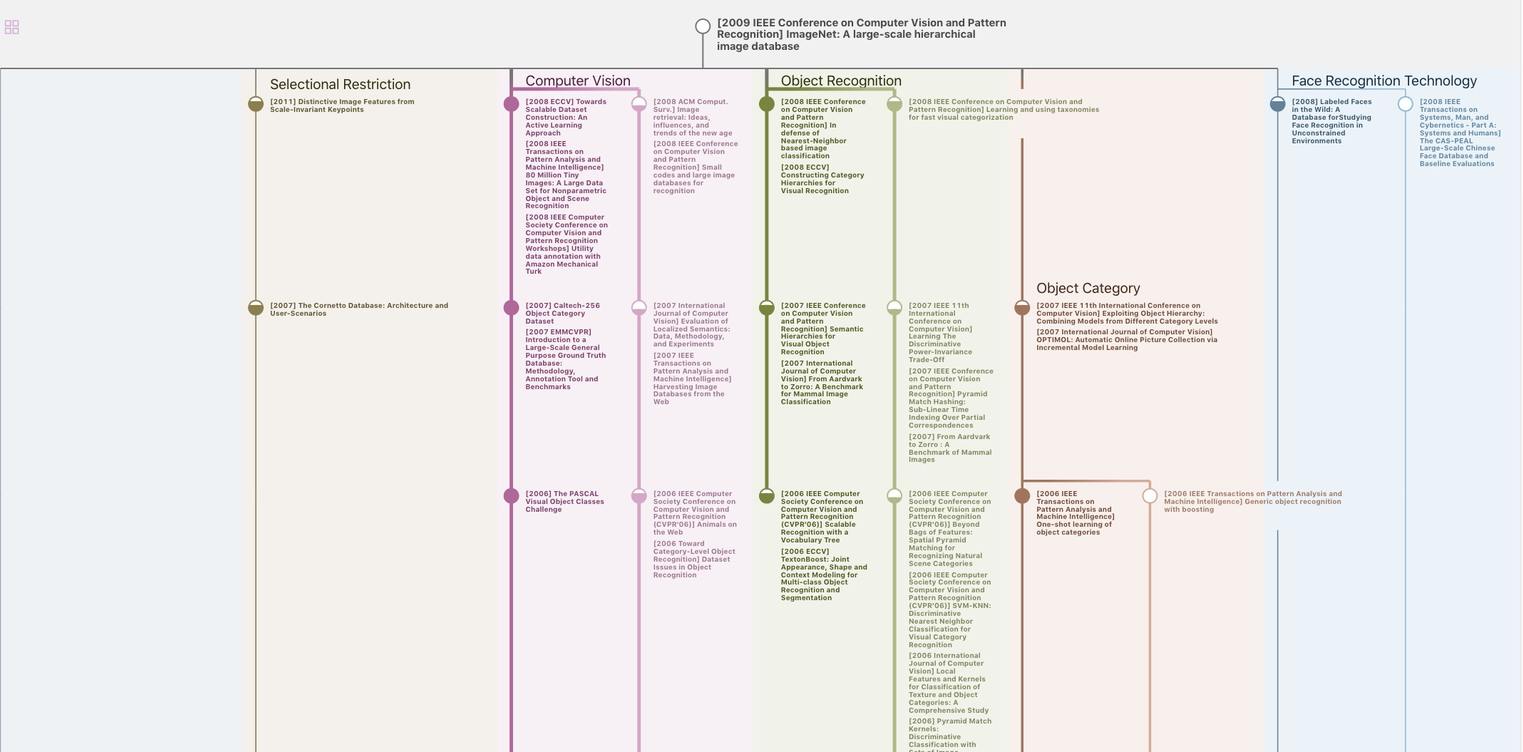
生成溯源树,研究论文发展脉络
Chat Paper
正在生成论文摘要