Lifting the Answer: Reranking Candidates on Data Augmented Text-to-SQL
2023 International Conference on Machine Learning and Cybernetics (ICMLC)(2023)
摘要
The text-to-SQL task is a realistic and challenging job, which aims to translate natural language questions into corresponding SQL queries. When attempting to generate SQL queries in the text-to-SQL task, prevailing semantic parsing models employ beam search to generate several candidates. Based on our pilot study, we observe that the gold-standard SQL answer may exist in the n-best candidate list produced by the decoder, rather than the first. Hence, we aim to lift the correct answer given several candidates generated by semantic parsing models for text-to-SQL. To this end, we propose a reranking module that reorders the n-best list of candidate SQL queries by pair-wise hinge loss. Meanwhile, a data augmentation module is leveraged to enrich the inadequate training instances for providing candidates with better qualities. These two modules can be easily grafted onto text-to-SQL backbone networks, and extensive experiments on the cross-domain text-to-SQL benchmark Spider demonstrate that our method achieves 73.8% in accuracy on the Spider dataset, surpassing the base model by up to 3.5%.
更多查看译文
关键词
Text-to-SQL,Reranking candidates,Data augmentation
AI 理解论文
溯源树
样例
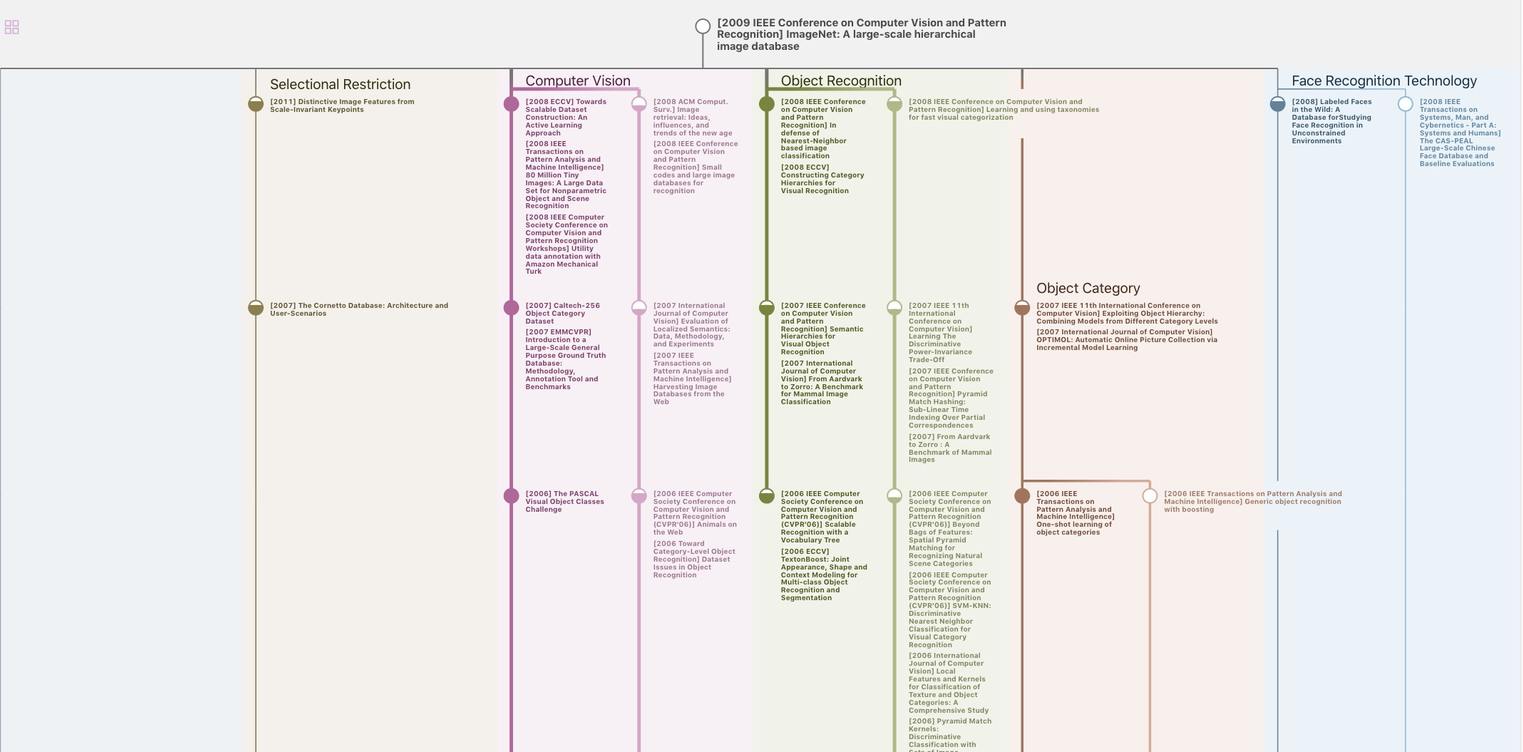
生成溯源树,研究论文发展脉络
Chat Paper
正在生成论文摘要