Transistor-based Modulator Modeling Technique Using Transfer Learning for Backscattered Communication Applications
2023 IEEE/MTT-S International Microwave Symposium - IMS 2023(2023)
摘要
A Load modulator using transistor is widely used in backscattering communications because of their low power consumption. Modeling of the load modulator is one of the most critical issue for design of backscattering tags. The conventional design approaches based on a simple parametric transistor model have inevitable errors due to the thermal or environmental noise sources. To achieve accurate higher-order modulation schemes, these errors should be corrected and modeled for better quality of service. This paper proposes active circuit modeling technique using ANN-based transfer learning. A pre-trained ANN model is built based on the gate bias and reflection coefficients through simulation. Then, the transfer learning technique is applied in order to build an accurate transistor model. The result of ANN-based transistor model through the transfer learning technique prevents overfitting for a small number of measurement datasets, and the modeled transistor provides 0.81% EVM in reflection coefficient estimation. An optimal algorithm for controlling the designed load modulator for QPSK is presented based on the newly modeled transistor.
更多查看译文
关键词
Internet of Things (IoT),machine learning,transfer learning,backscatter communications,Artificial Neural Network (ANN),K-Nearest Neighbor (KNN)
AI 理解论文
溯源树
样例
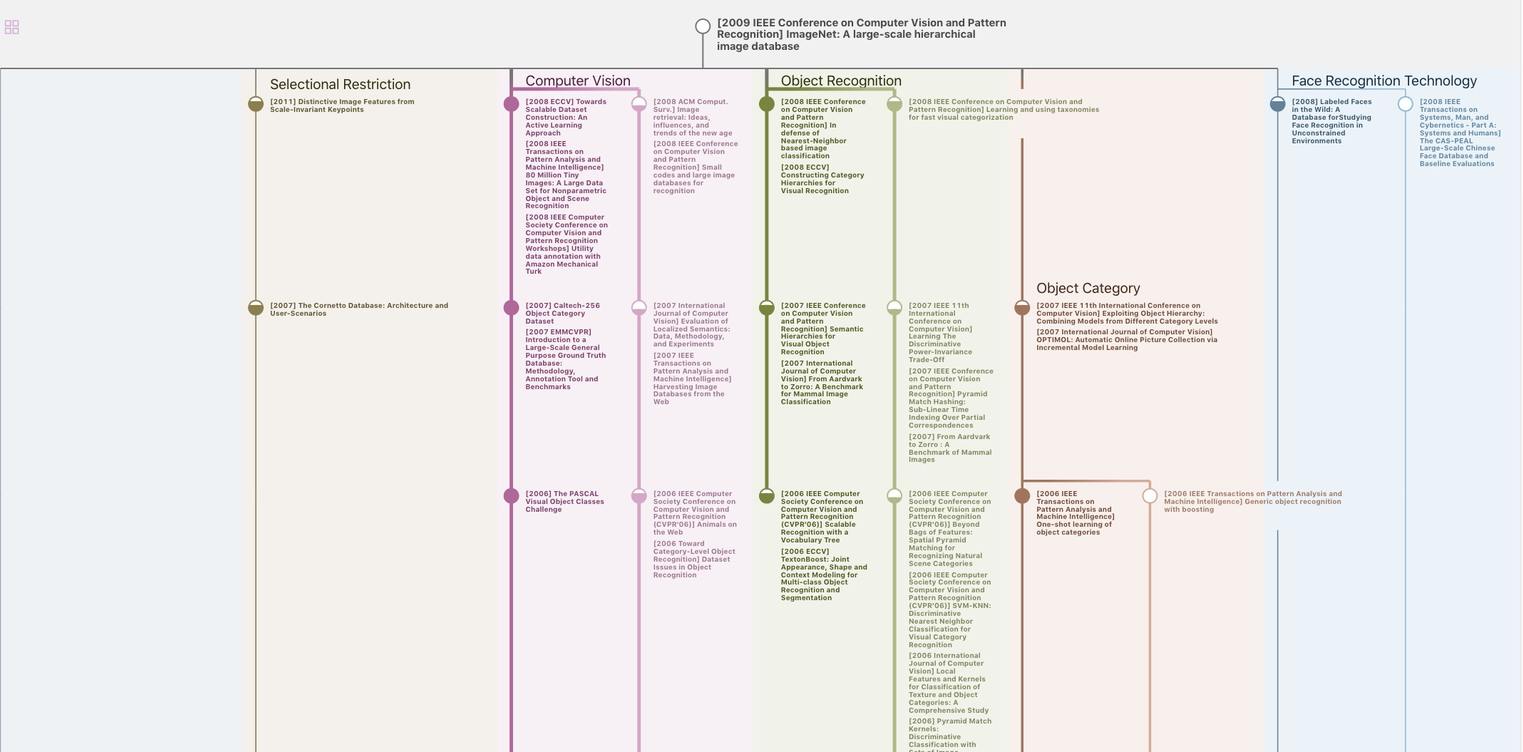
生成溯源树,研究论文发展脉络
Chat Paper
正在生成论文摘要