Resource Allocation of Multi-user Workloads in Cloud and Edge Data-Centers Using Reinforcement Learning
2023 19TH INTERNATIONAL CONFERENCE ON NETWORK AND SERVICE MANAGEMENT, CNSM(2023)
摘要
Cloud and edge Data-center (DC) are designed to allocate computing resources dynamically to users based on the agreed Service Level Agreement (SLA). However, the ever-increasing demand for beyond 5G services necessitates an efficient workload management. A key challenge in this regard is auto-scaling, a dynamic process that adjusts computing resources to meet fluctuating system demands, optimizing resource utilization and cost efficiency. Traditional auto-scaling algorithms, which rely on fixed thresholds or control-theory, may face limitations in modern DC which are characterized by diverse, dynamic, and multi-user workloads. In this paper, we propose a Reinforcement Learning (RL)-based controller that extends the capacity of the state-of-the-art RL-based auto-scalers to the multi-user workload scenario. We compare the proposed RL agent against the well-known Proportional-Integral (PI) controller and a Threshold (THD)-based controller in a multi-user workload scenario in terms of created Cloud-native Network Functions (CNFs) and peak latency performed in a discrete event simulator.
更多查看译文
关键词
Auto-scaling,Workload Management,Reinforcement Learning,Cloud-Native Network Function
AI 理解论文
溯源树
样例
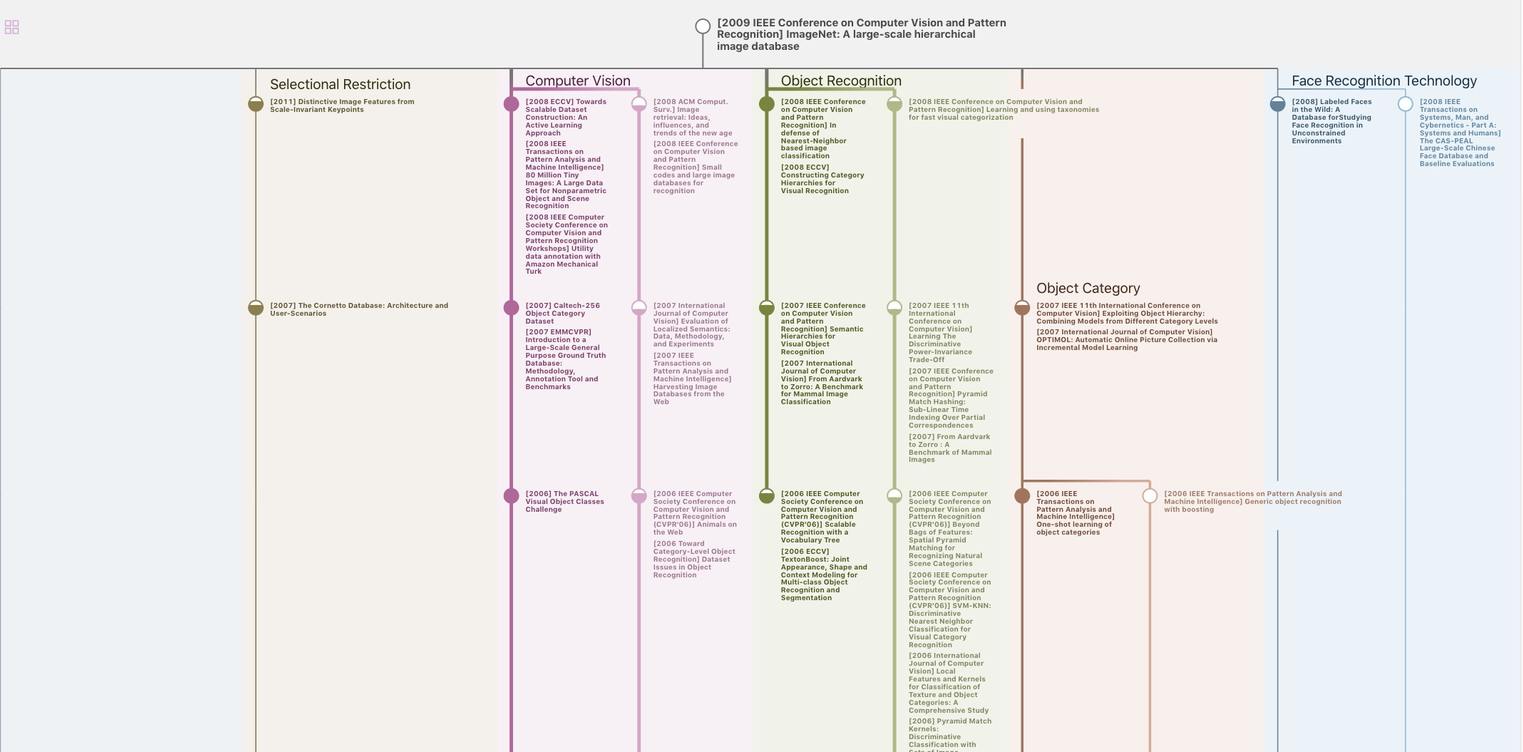
生成溯源树,研究论文发展脉络
Chat Paper
正在生成论文摘要