A Critical Study of Few-shot Learning for Encrypted Traffic Classification
2023 19TH INTERNATIONAL CONFERENCE ON NETWORK AND SERVICE MANAGEMENT, CNSM(2023)
摘要
Over the past twenty years, a plethora of methods have been proposed for encrypted traffic classification (ETC), while the Server name indication (SNI) is deemed to solve the problem of classification for TLS traffic. However, SNI-based classification has its pitfalls and the SNI will likely be pushed into the encrypted tunnel in the future. In this work, we envision a futuristic scenario in which encrypted SNI is the norm and labeled traffic flows are scarce. In such settings, we tackle the problem of traffic classification at ISP level using few-shot learning. By means of six real-world ISP-level datasets collected between 2019 and 2021 and two publicly available client-side datasets, we study the performance of a few-shot learner on TLS data, including its cross-dataset generalizability. We further investigate the effect of the number of required labeled samples on the learner's performance. Our experiments show that the dataset-specificity of deep learners carries over to few-shot meta-learning, and calls for addressing the problem of generalizability for deep learning architectures.
更多查看译文
关键词
Encrypted traffic classification,Meta-learning,Few-shot learning,Matching networks
AI 理解论文
溯源树
样例
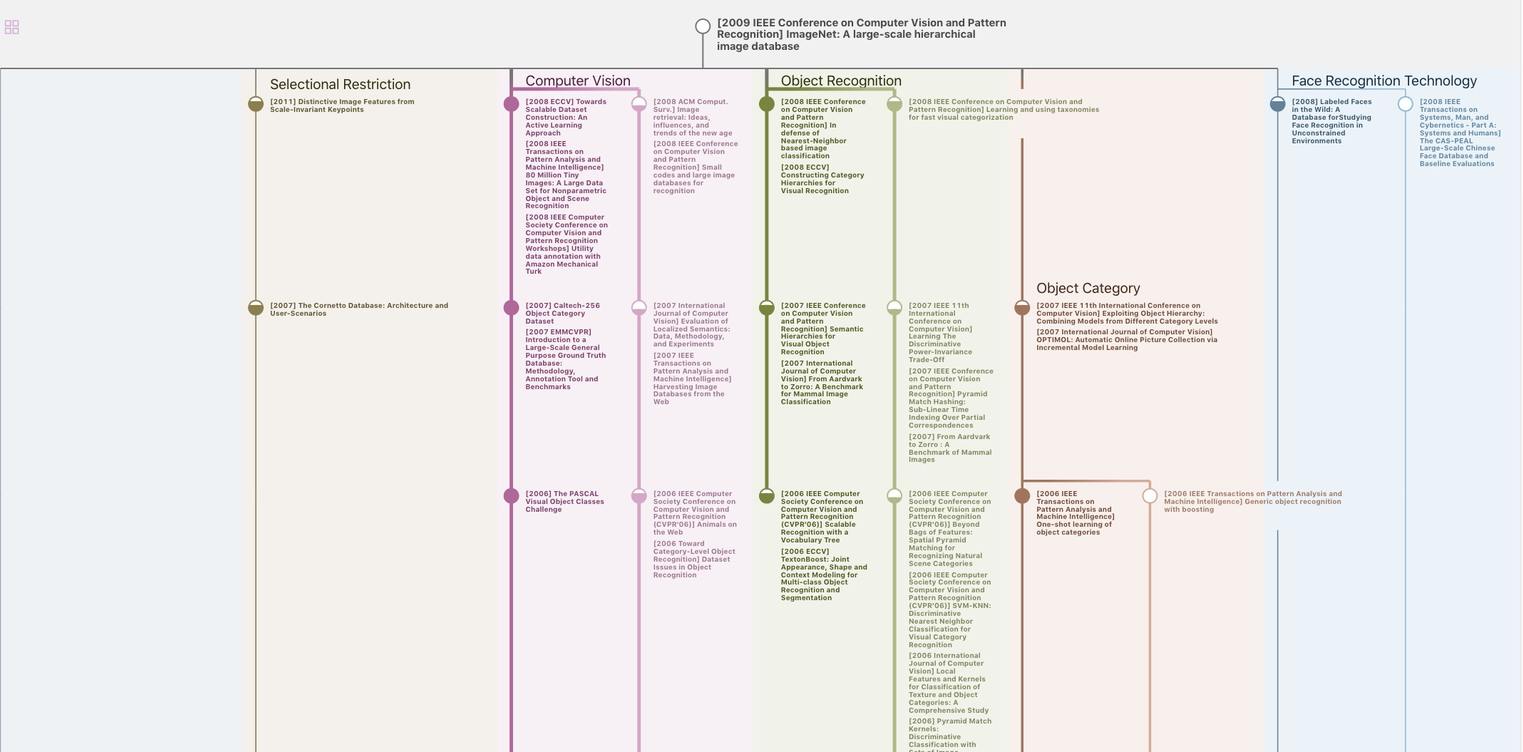
生成溯源树,研究论文发展脉络
Chat Paper
正在生成论文摘要