Analyzing the Quality of Synthetic Adversarial Cyberattacks
2023 19TH INTERNATIONAL CONFERENCE ON NETWORK AND SERVICE MANAGEMENT, CNSM(2023)
摘要
Today's networked systems face significant security challenges due to sophisticated attacks. Several Machine Learning (ML) and Deep Learning (DL) models are employed to combat these diverse attacks. Adversarial attacks, which can evade detection by AI-based intrusion detection systems (IDS) through small alterations to network attack traffic, pose a significant concern. These AI-synthesized adversarial attacks must adhere to network constraints to seem plausible. In this work, we explore the validation criteria for such adversarial attacks and propose a methodology for analyzing their quality. We evaluate adversarial attack samples synthesized by state-of-the-art generative DL models such as Variational autoencoder (VAE), Conditional Variational autoencoder (CVAE), Generative Adversarial Network (GAN) and compare the performance with our CVAE-Adversarial Network (CVAE-AN) model. Results indicate the effectiveness of CVAE-AN in synthesizing realistic adversarial attacks.
更多查看译文
关键词
Attack quality,Deep Learning,Generative models,Intrusion detection,Realistic adversarial attacks
AI 理解论文
溯源树
样例
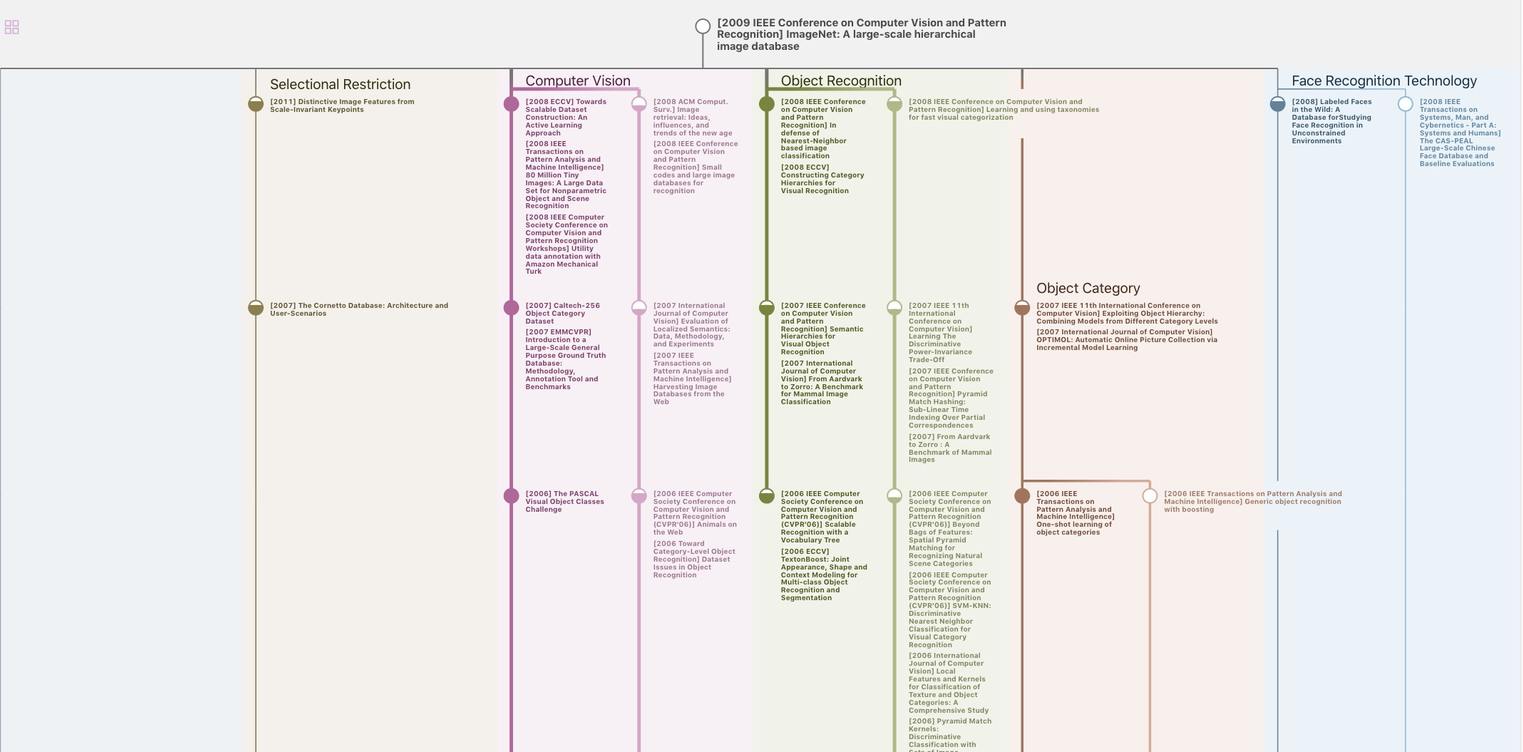
生成溯源树,研究论文发展脉络
Chat Paper
正在生成论文摘要