CML-IDS: Enhancing Intrusion Detection in SDN through Collaborative Machine Learning
2023 19TH INTERNATIONAL CONFERENCE ON NETWORK AND SERVICE MANAGEMENT, CNSM(2023)
摘要
The centralized control plane in Software-Defined Networking (SDN) offers significant advancements in network management capabilities. However, SDN is also susceptible to cybersecurity risks and vulnerabilities. Deploying the Machine Learning (ML) approach in an Intrusion Detection System (IDS) can facilitate early detection of potential vulnerabilities. However, deploying an ML-based IDS solely in either the SDN control plane or the data plane has its benefits and drawbacks. For instance, a high-capacity ML model deployed in the control plane can enhance the detection performance but may increase network latency and the risk of overwhelming the control plane. In contrast, lightweight ML models deployed in the data plane could accelerate intrusion detection with lower detection performance. However, a functional IDS should provide a good detection performance at a line rate. To accomplish these objectives, we introduce a novel method called Collaborative ML-based IDS (CML-IDS), which involves deploying ML models in both the control and data planes to detect network attacks collaboratively. To facilitate this collaboration, we assess the confidence of the classification model, which is flexibly deployed within the programmable data plane. Our evaluation results demonstrate that the CML-IDS enhances the average intrusion detection performance to 93.46% and reduces the misclassification rate by 54.66% when compared to an IDS that solely relies on the ML model deployed in the data plane. Furthermore, CML-IDS effectively reduces network latency caused by forwarding flows to the control plane.
更多查看译文
关键词
Intrusion Detection System,Machine Learning Models,P4 Switches,Software Defined Networking
AI 理解论文
溯源树
样例
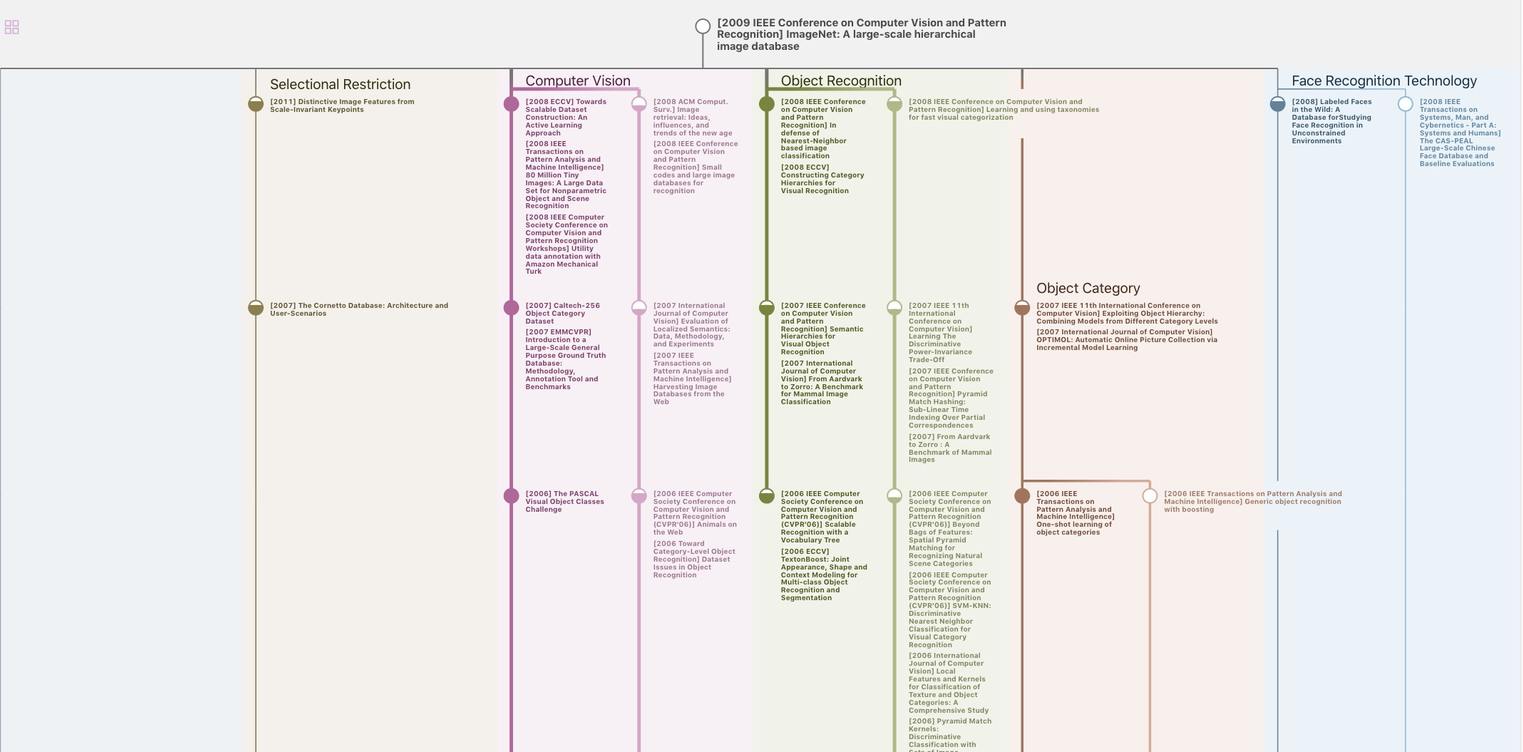
生成溯源树,研究论文发展脉络
Chat Paper
正在生成论文摘要