Leveraging Spatiotemporal Relations for Predicting Potential Link Failures
2023 19TH INTERNATIONAL CONFERENCE ON NETWORK AND SERVICE MANAGEMENT, CNSM(2023)
摘要
Being able to predict link failures in advance would be of great benefit to network operators. We use Machine Learning (ML) techniques to extract temporal and spatial relations from real network data and use them to predict link failures. We use Interior Gateway Protocol (IGP) configuration changes as a guide to achieve this. We predict link failures in the next five days based on data collected from the previous five days. We propose a modified Variational Auto Encoder (VAE) model to compress the higher dimensional dataset into a latent space that captures time-based relations in the data. We demonstrate that five days is the smallest look-back window of time required to get satisfactory prediction results. Using feature importance plots, we learned that the VAE model was able to capture intricate time-based dependencies in the error counter features to achieve good performance. In addition, using a Graph Convolutional Network (GCN), we were able to aggregate data from neighboring links to improve the model's performance. Neighbors up to two hops away carried relevant information in IGP metric settings and in traffic metric counter features. The relevance of the correlation of the features in time and space is confirmed using standard feature importance wrapper methods. Finally, by combining the VAE and GCN components, we were able to extract spatial and temporal features in conjunction, leading to further improvements. These ML approaches significantly improve existing manual methods of tracking metrics in time and space currently followed by the operator.
更多查看译文
关键词
IGP,Transformers,VAE,GCN,Machine Learning,Feature Importance,Time Series,Graphs
AI 理解论文
溯源树
样例
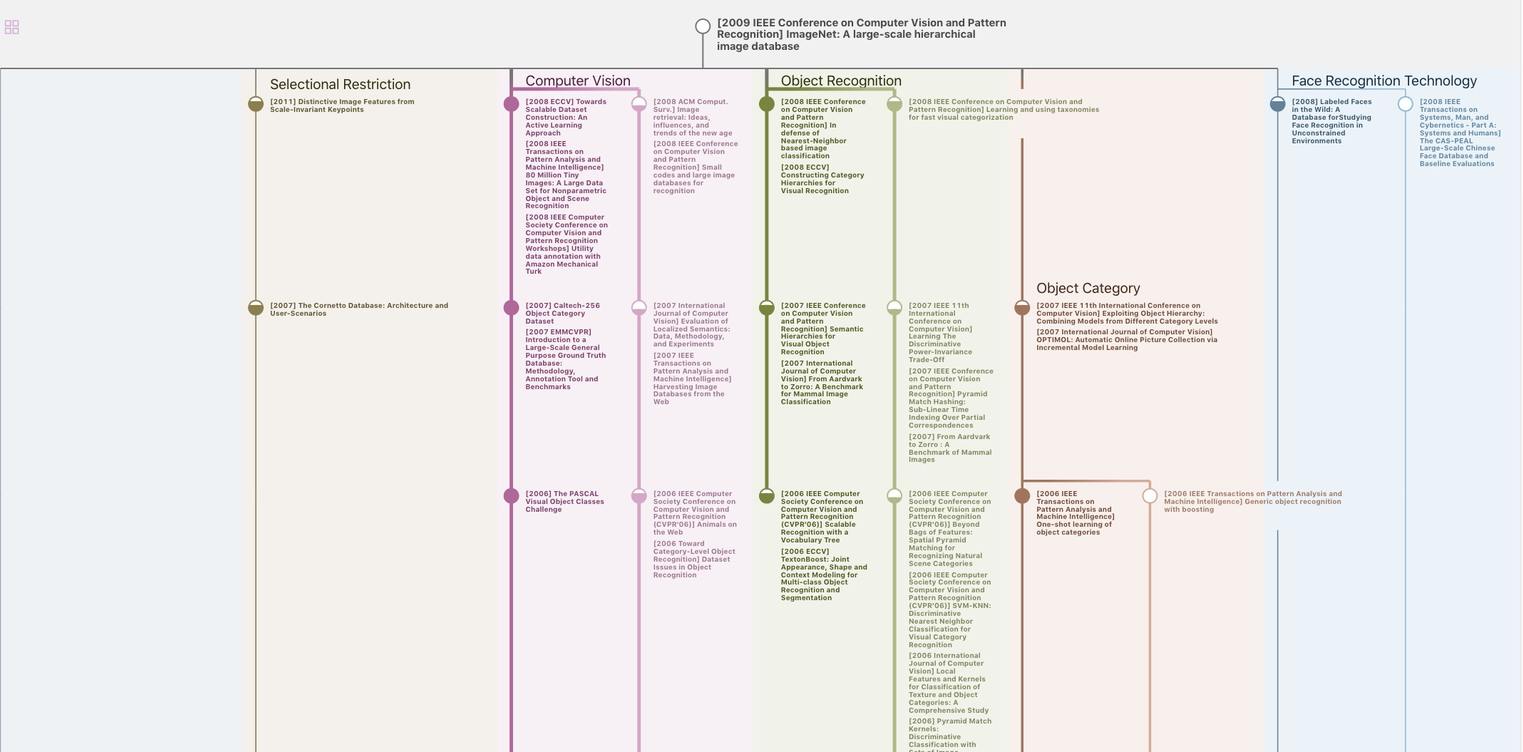
生成溯源树,研究论文发展脉络
Chat Paper
正在生成论文摘要