CrossBal: Data and Control Plane Cooperation for Efficient and Scalable Network Load Balancing
2023 19TH INTERNATIONAL CONFERENCE ON NETWORK AND SERVICE MANAGEMENT, CNSM(2023)
摘要
Load balancing network traffic through multiple shortest-paths has become common practice to efficiently utilize the network infrastructure. Despite widespread adoption, Equal-Cost Multi-Path (ECMP) delivers performance far from optimal. Several load balancing solutions utilizeWeighted-Cost Multi-Path (WCMP), splitting incoming traffic between links proportionally to link weights. However, implementing WCMP requires the controller to update match+action rules whenever the weights must be changed, introducing a delay before the appropriate traffic split can be applied. Additionally, weighted traffic splits are applied over network flows without regard to flow characteristics or needs. We propose CrossBal, a hybrid load balancing system based on Deep Reinforcement Learning (DRL) that focuses its efforts on high-impact elephant flows. The DRL agent is modeled to be able to efficiently utilize network links while minimizing the action space, allowing the agent to quickly learn how to load balance. Further, CrossBal can quickly react to network changes by monitoring and switching active routes directly in the data plane. Our evaluation shows that CrossBal can efficiently utilize network resources, using most available links, while also reducing link utilization imbalance. We also evaluate the elephant flow detection employed by CrossBal, showing how it can quickly identify elephant flows while efficiently utilizing switch resources.
更多查看译文
关键词
Load Balancing,Traffic Engineering,Deep Reinforcement Learning,Machine Learning,Programmable Data Planes,Elephant Flow
AI 理解论文
溯源树
样例
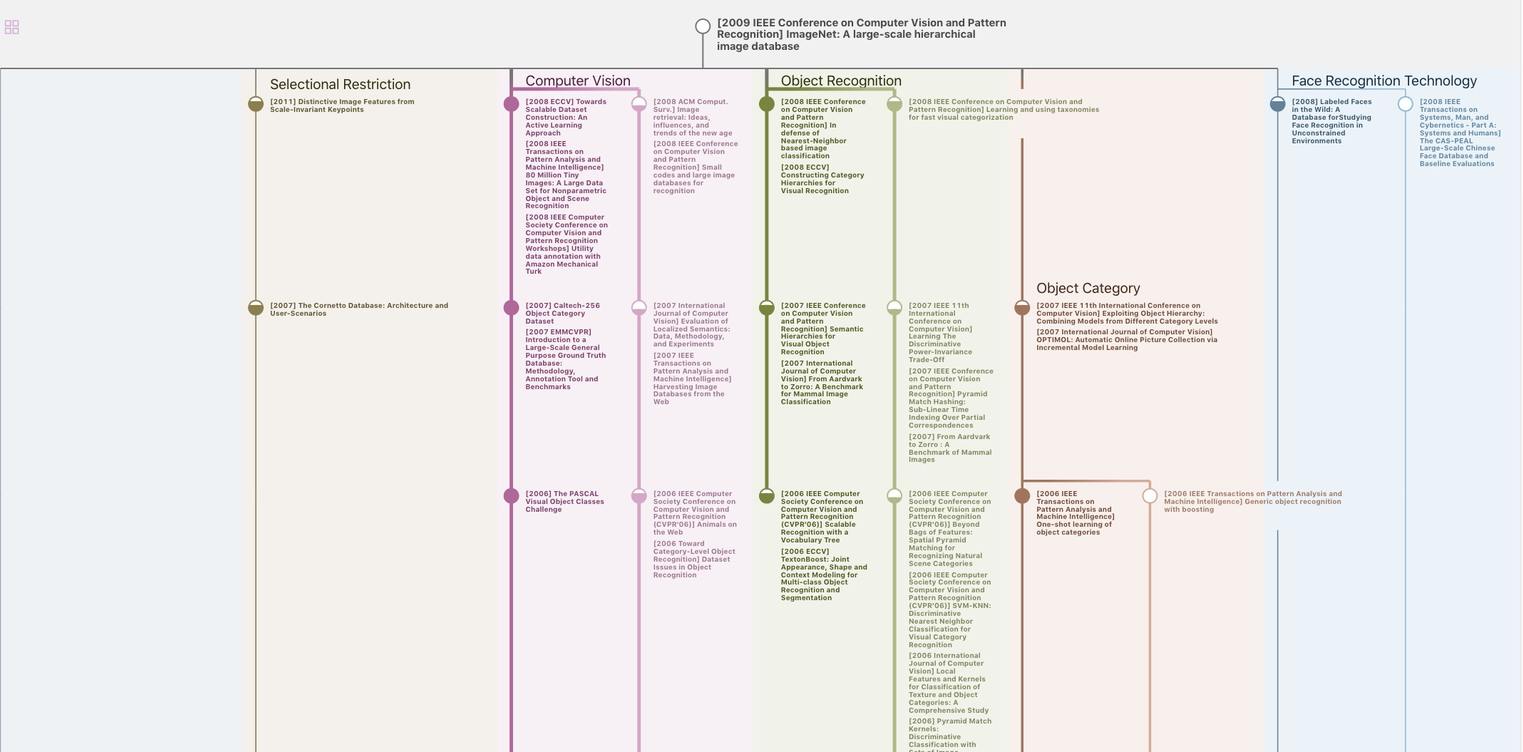
生成溯源树,研究论文发展脉络
Chat Paper
正在生成论文摘要