Early Identification of Battery End-of-Life Using Machine Learning
2023 13th International Conference on Power, Energy and Electrical Engineering (CPEEE)(2023)
摘要
This paper presents a methodology to predict near End-of-Life (EOL) of lithium-ion batteries. By predicting if a battery is expected to fail (unable to be discharged to 70% of its nominal capacity) within 30 cycles, timely replacement of battery can be carried out to minimize downtime. This methodology is validated using data from NASA Prognostics Center of Excellence. The battery charge cycling data is extracted and preprocessed as time series input features. The input features are based on mean and range of charge voltage, charge Ah capacity, charge time, and temperature. These features are used to train various machine learning techniques to perform multiclass classification on battery status. Battery status is categorized into three status, namely nonfailure status, near failure status (battery EOL is reached within 30 cycles), and failure status (battery EOL has already been reached). From various machine learning techniques studied, Fine kNN, ensemble bagged trees and ensemble boosted trees are three best techniques with more than 90% accuracy.
更多查看译文
关键词
battery PHM,prediction of battery EOL,machine learning
AI 理解论文
溯源树
样例
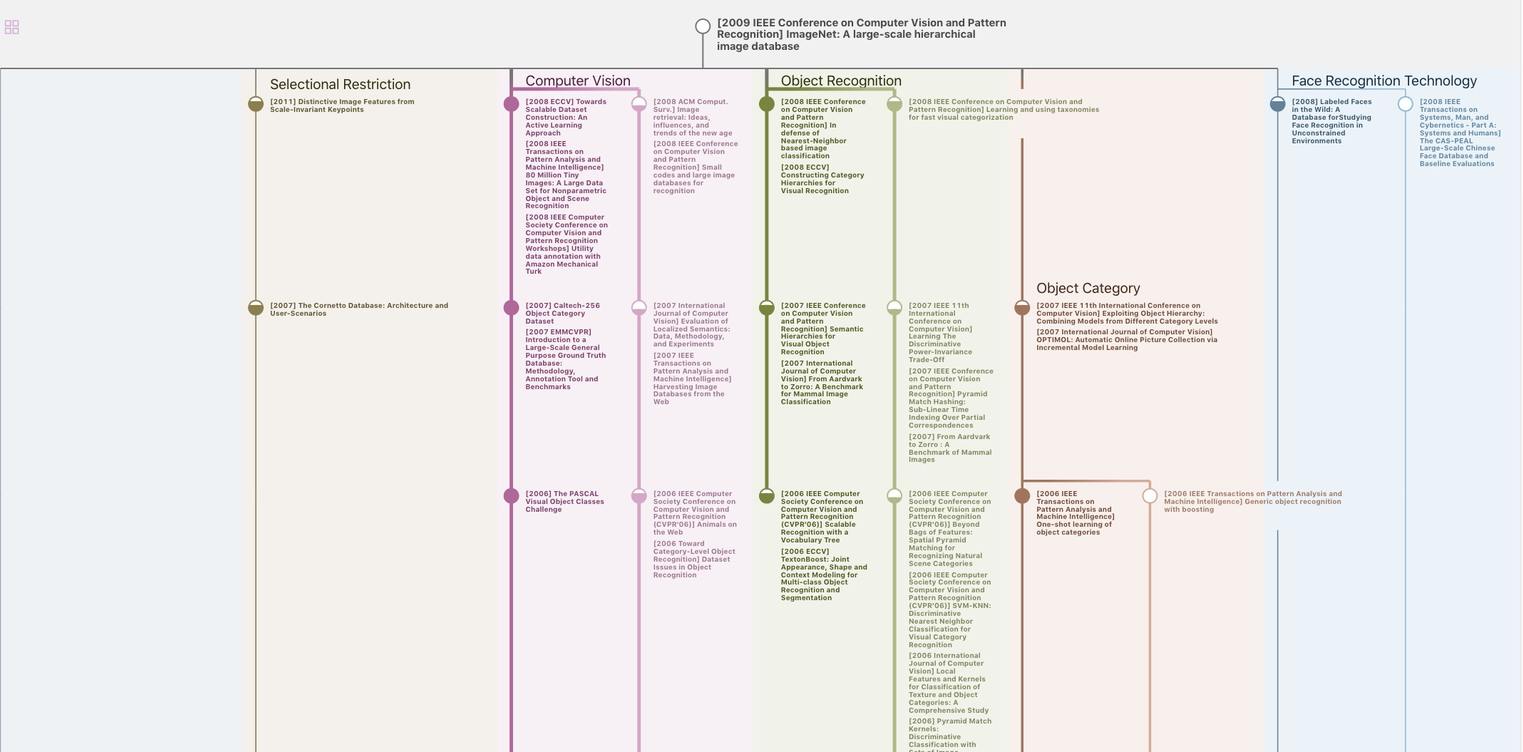
生成溯源树,研究论文发展脉络
Chat Paper
正在生成论文摘要