Hyperspectral anomaly detection based on background purification via deep autoencoding gaussian mixture model
IGARSS 2023 - 2023 IEEE INTERNATIONAL GEOSCIENCE AND REMOTE SENSING SYMPOSIUM(2023)
摘要
The primary task of hyperspectral anomaly detection (HAD) is to distinguish targets with noticeable spectral variances from their surroundings. Background purification for dictionary construction is vital for representation based HAD. In this letter, we present a novel HAD method based on background purification via deep autoencoding gaussian mixture Model. First, the low-rank representation (LRR) model is applied to separate the sparse anomalies from the low-rank background component. Secondly, we innovatively apply the deep autoencoding gaussian mixture model (DAGMM) for dimensionality reduction and background purification, which combines the feature mapping and Gaussian density estimation for joint training in the deep latent space. In addition, we propose a dictionary construction strategy based on the gaussian mixture model, achieving the exclusion of possible anomalies and inclusion of background features. Experiments on two real datasets illustrated the superior performance for HAD task of the proposed method.
更多查看译文
关键词
hyperspectral anomaly detection (HAD),low-rank representation (LRR),background purification,deep autoencoding gaussian mixture model (DAGMM)
AI 理解论文
溯源树
样例
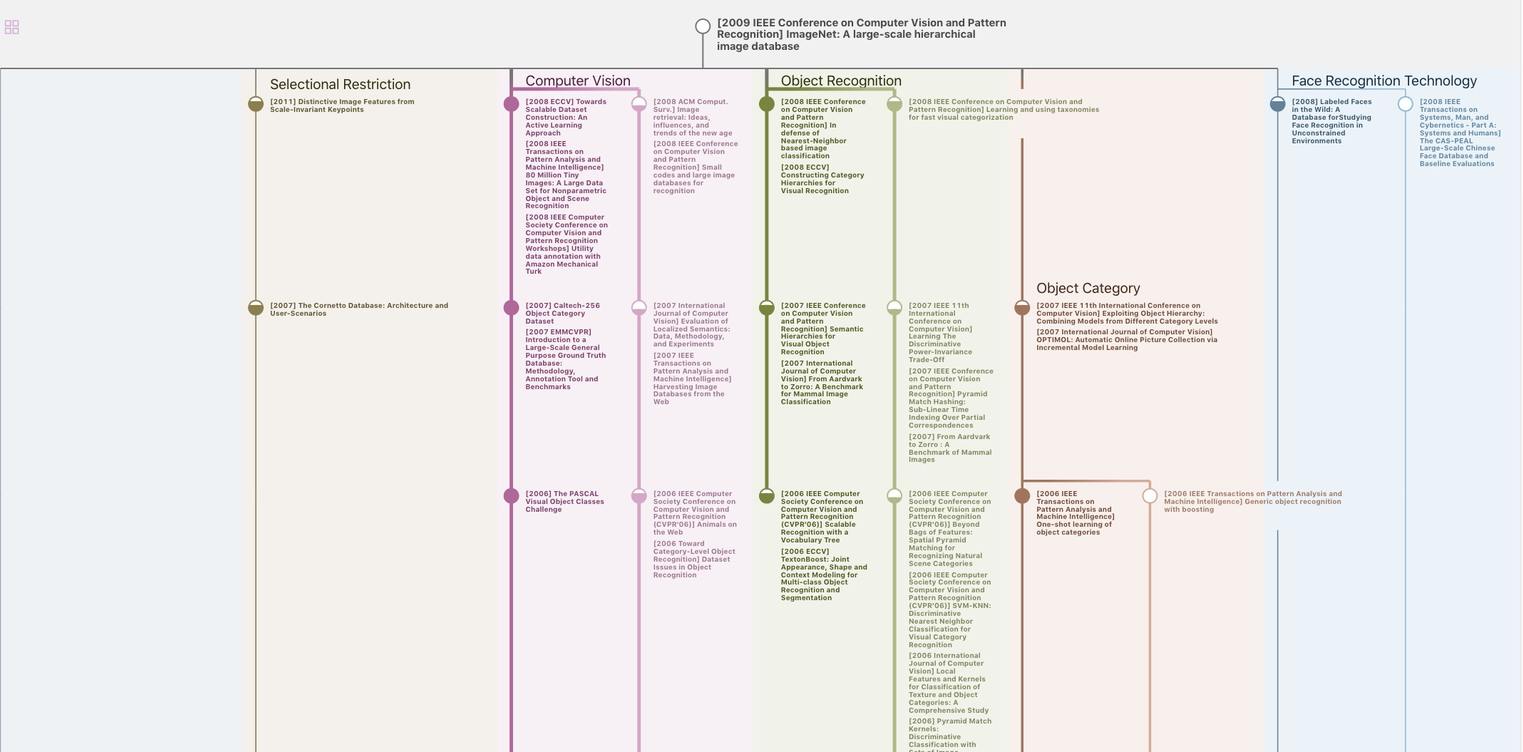
生成溯源树,研究论文发展脉络
Chat Paper
正在生成论文摘要