CloudLoc-NeRF: Point-cloud Assisted Volume Location for Neural Radiance Fields
IGARSS 2023 - 2023 IEEE INTERNATIONAL GEOSCIENCE AND REMOTE SENSING SYMPOSIUM(2023)
摘要
Realistic rendering results are available to be generated by volume-based neural rendering methods like NeRF. However, the existing schemes take the color vector as the unique supervision information, which leads to ambiguous prediction results of the volume density in the same spatial position through different rendering rays. This problem is common in large-scale scene reconstruction based on remote sensing images taken by UAVs and other equipment. To this end, we contrive to integrate spatial point cloud and multiview image information, making the sparse point cloud the calibration for key features and regions. Therefore, the CloudLoc-NeRF is proposed. For volume information extraction, the multi-resolution hash coding and voxel are adopted to estimate the district for ray marching and extract volume features efficiently. For the point cloud, annular sampling and plane coding are used to combine image features of the training views and the point cloud. The regions with high feature response in multiple modal data should correspond to the regions with high volume density. In addition, an optimization method based on point cloud density is proposed. The weight parameter of volume density confidence is constructed to symbolize the correlation between density distribution and point cloud density. We verified the performance of our method on NVSF and the wide-area scene reconstruction dataset. Experiments showed that CloudLoc-NeRF accurately expresses the details of the rendered scene and produces better view synthesis results.
更多查看译文
关键词
NeRF,point cloud,volume rendering,neural network
AI 理解论文
溯源树
样例
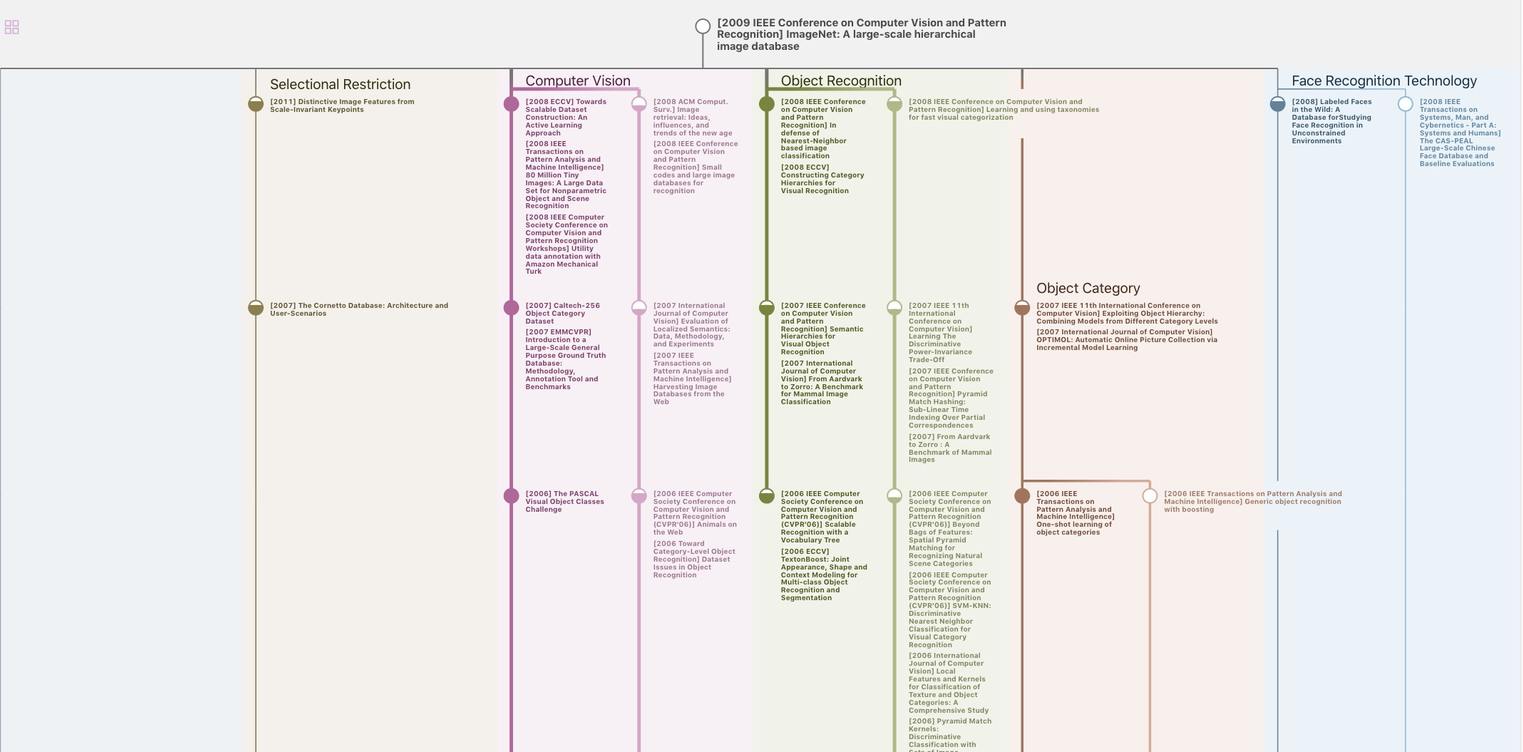
生成溯源树,研究论文发展脉络
Chat Paper
正在生成论文摘要