Unsupervised hyperspectral image domain adaptation through unmixing-based domain alignment
IGARSS 2023 - 2023 IEEE INTERNATIONAL GEOSCIENCE AND REMOTE SENSING SYMPOSIUM(2023)
摘要
Despite the great progress in hyperspectral image classification, it is still challenging due to the unique characteristic of satellite imagery where the training and test sets may come from different distributions because of the different acquisition conditions. Hence, directly deploying the trained model on the test data may lead to degradation in the performance. In this work, we propose an unsupervised domain adaptation approach that aligns distributions across the training and test domains. It projects the data to a shared embedding space, i.e., the abundance space, that is regularized by physical constraints. The shared abundance space, together with a metric-based distribution alignment approach applied on the abundance space, would largely reduce the domain discrepancy and provide a more representative feature set for classification purpose. Experimental results on hyperspectral benchmarks demonstrate superiority of the proposed method.
更多查看译文
关键词
Domain adaptation,spectral unmixing
AI 理解论文
溯源树
样例
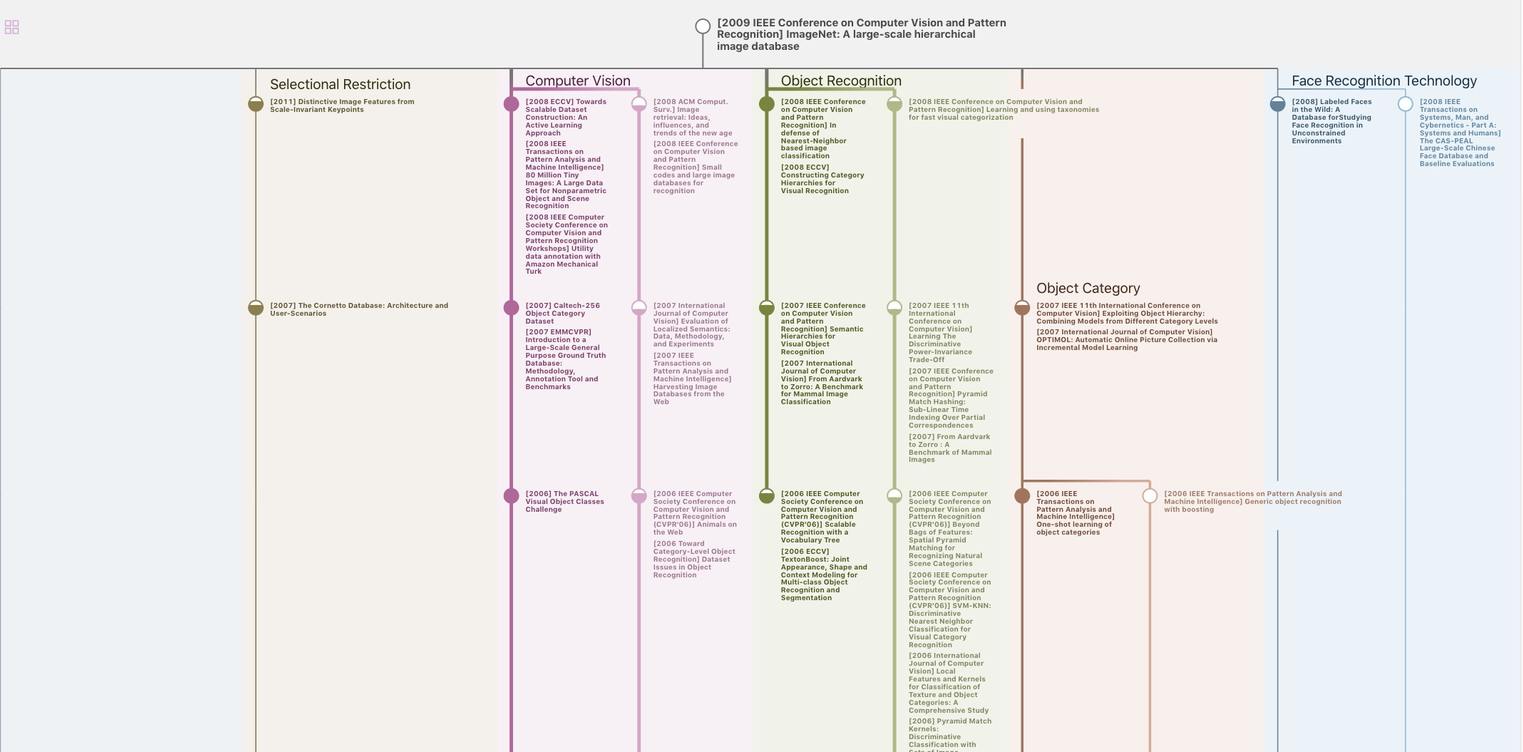
生成溯源树,研究论文发展脉络
Chat Paper
正在生成论文摘要