Iterative autoencoder coupling with constrained energy minimization for hyperspectral target detection
IGARSS 2023 - 2023 IEEE INTERNATIONAL GEOSCIENCE AND REMOTE SENSING SYMPOSIUM(2023)
摘要
The common purpose of HTD is to distinguish the target from the BKG in HSIs using either fully-known or partlyknown prior knowledge. Traditional HTD methods generally rely on distance or similarity measurement and statistical techniques to identify discriminating characteristics. Inspired by the simplicity and efficiency of AE in feature extraction and dimension reduction, and considering the susceptibility of limited prior knowledge to spectral variability, a novel HTD method based on hidden dimension-restricted iterative AE guided by posterior knowledge is proposed. The latent space mapping from the original data is constrained with the concept of VD by the algorithm of NWHFC, which allows the reconstruction more tendentious to target and purified BKG. The posterior knowledge, obtained by CEM on a subpixel level, is iteratively added to the input of AE to improve the diversity of the target and BKG collaboratively. The results show that the BKG is effectively suppressed and the target is prominently highlighted layer by layer. Comprehensive experiments and analysis conducted on the public dataset demonstrate that the proposed method is structurally simple yet efficient, outperforming five state-of-the-art algorithms rank by 3-D ROC. The key indicator, target detectability, exceeds the comparison methods by 51.1%.
更多查看译文
关键词
Hyperspectral target detection (HTD),iterative autoencoder (IAE),posterior knowledge,target detectability,background suppression
AI 理解论文
溯源树
样例
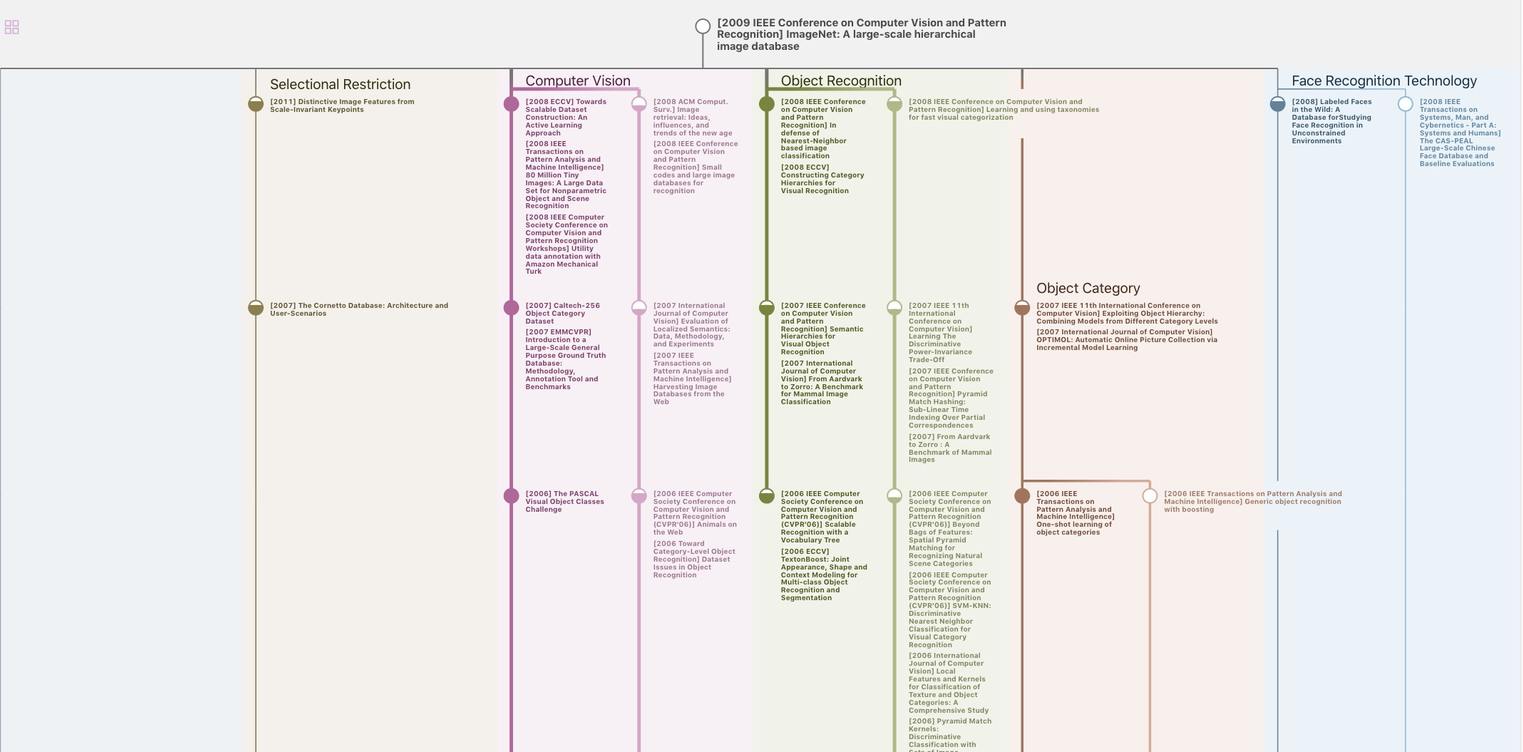
生成溯源树,研究论文发展脉络
Chat Paper
正在生成论文摘要