A meteorology-driven lstm for predicting time series of vegetation leaf area index in land surface model
IGARSS 2023 - 2023 IEEE INTERNATIONAL GEOSCIENCE AND REMOTE SENSING SYMPOSIUM(2023)
摘要
The leaf area index (LAI) is a basic vegetation structural variable in the ecosystem and climate model. Predicting vegetation LAI time series is essential for understanding the response of vegetation growth processes to climate change. Current predictive numerical phenology models are still subject to large uncertainties. Developing LAI time series prediction models is beneficial to improve the ability of land surface models to predict future vegetation growth. This study develops a deep learning model based on meteorological variables and attentional mechanisms to predict vegetation LAI time series. We tested our ALSTM for different biome types across North America and evaluated the model using satellite and flux tower data. The results demonstrated that the ALSTM outperforms other baseline models. The predicted ALSTM LAI shows high temporal and spatial consistency compared to satellite-based LAI. The ALSTM model can effectively predict downstream gross primary productivity (GPP) when coupled with the common land model ( CoLM). ALSTM performs high accuracy in predicting the LAI of terrestrial vegetation and provides a reference for developing biophysical methods to predict future vegetation ecological processes for land surface models.
更多查看译文
关键词
LSTM,LAI,land surface model,deep learning,terrestrial vegetation
AI 理解论文
溯源树
样例
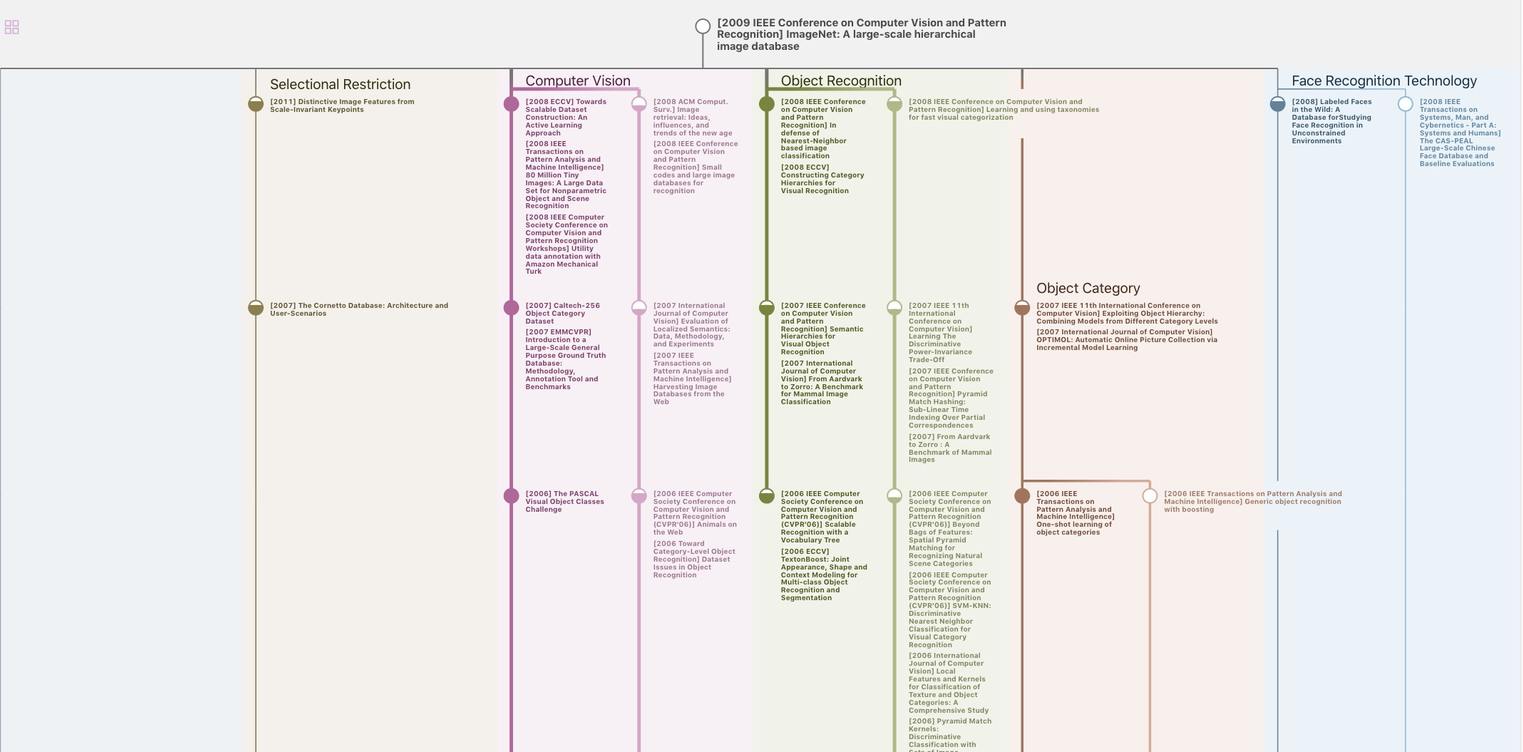
生成溯源树,研究论文发展脉络
Chat Paper
正在生成论文摘要