Learned masked robust principal component analysis model for infrared small target detection
IGARSS 2023 - 2023 IEEE INTERNATIONAL GEOSCIENCE AND REMOTE SENSING SYMPOSIUM(2023)
摘要
We proposed a learned masked robust principal component analysis (LMRPCA) algorithm for single-frame infrared small target detection. Firstly, the original images are constructed into patch images, which are separated into low-rank and sparse components corresponding to the backgrounds and foreground masks. The optimization function is solved by alternating directions of multipliers method (ADMM), which is mapped to trainable convolutional layers. We use elements of convolutional sparse coding to improve representation learning for foreground masks and side information in the auxiliary transform domain. By doing so, we assign learnable weights to different feature maps by using a reweighted-l(1) - l(1) minimization. Numerical experiments show that our proposed LMRPCA can segment and locate the targets precisely.
更多查看译文
关键词
Small infrared target,learned infrared patch-image model,deep network
AI 理解论文
溯源树
样例
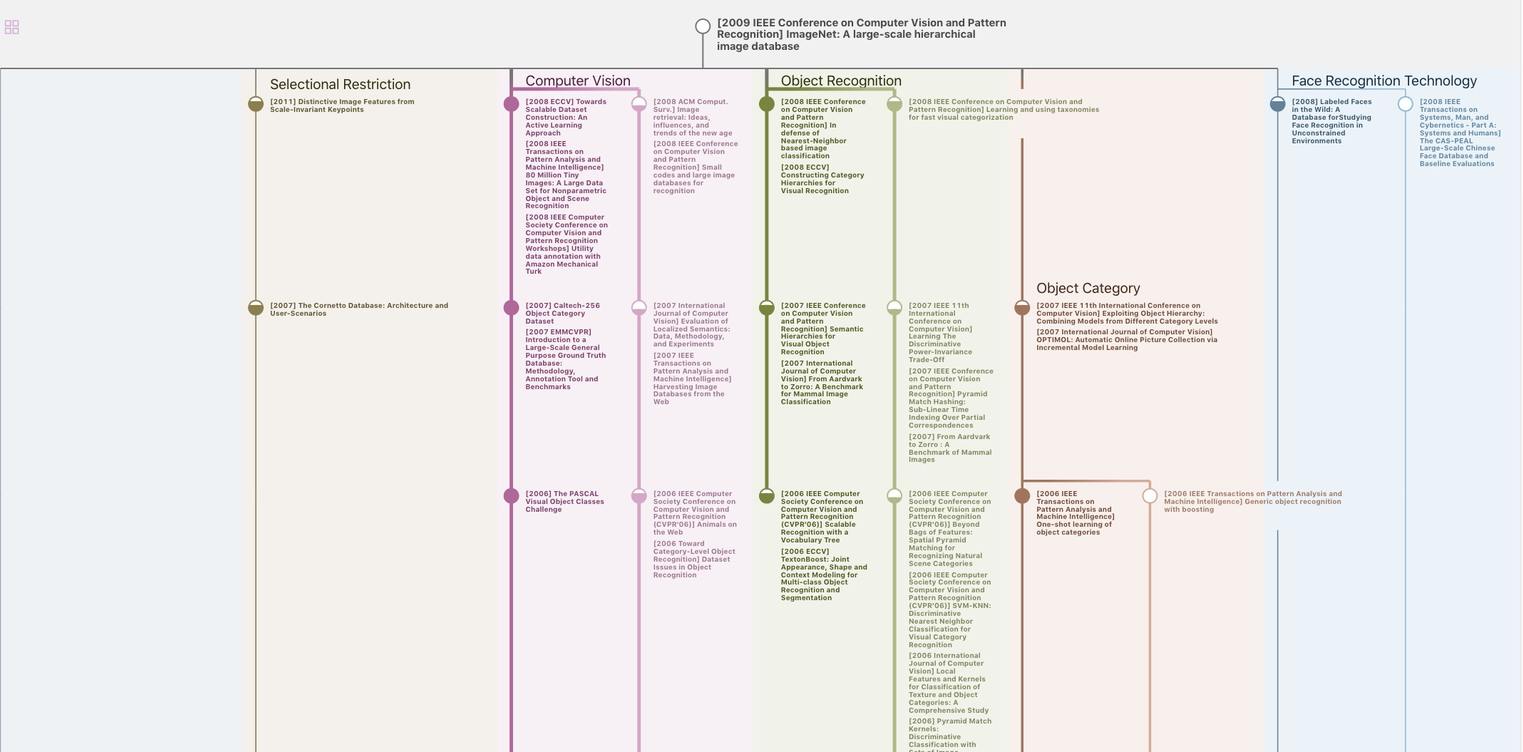
生成溯源树,研究论文发展脉络
Chat Paper
正在生成论文摘要