Sure-ergas: unsupervised deep learning multispectral and hyperspectral image fusion
IGARSS 2023 - 2023 IEEE INTERNATIONAL GEOSCIENCE AND REMOTE SENSING SYMPOSIUM(2023)
摘要
This paper proposes a new loss function to train a convolutional neural network (CNN) for multispectral and hyperspectral (MS-HS) image fusion. The loss function is based on the relative dimensionless global error synthesis (ERGAS), where we exchange the mean squared error (MSE) for its unbiased estimate using Stein's risk unbiased estimate (SURE). The loss function has a good balance between the spectral and spatial information implied by the weighted MSE, therefore it does not need a parameter to balance the spectral and spatial terms as in MSE loss function, and it also converges faster than the MSE one. Additionally, the loss function enables unsupervised training and avoids over-fitting, since it is derived by using SURE. Experimental results show that the proposed method yields good results and outperforms the competitive methods. Codes are available at https://github.com/hvn2/SURE-ERGAS
更多查看译文
关键词
Hyperspectral and multispectral image fusion,Stein's unbiased risk estimate (SURE),ERGAS,unsupervised CNN
AI 理解论文
溯源树
样例
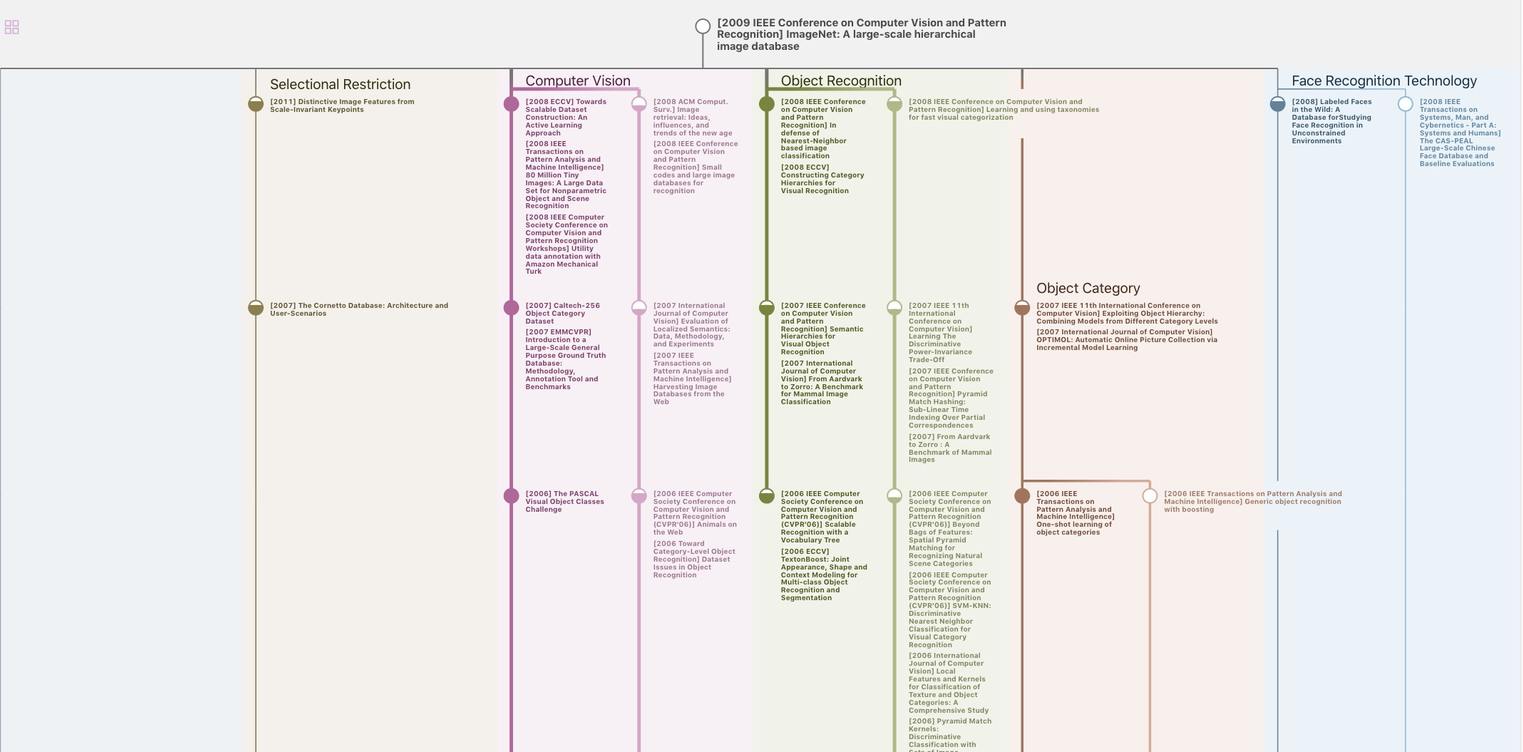
生成溯源树,研究论文发展脉络
Chat Paper
正在生成论文摘要