Fully automatic generation of training data for building detection and classification from remote sensing imagery
IGARSS 2023 - 2023 IEEE INTERNATIONAL GEOSCIENCE AND REMOTE SENSING SYMPOSIUM(2023)
摘要
Training data is an essential ingredient for the development of deep learning approaches. Yet, the preparation of training datasets for building detection and classification in remote sensing images implies substantial manual work and is, therefore, expensive concerning both labor charges and time. Since manual annotation also strongly depends on the experience and expertise of the annotators, quality control is an unavoidable issue. It is, thus, of great interest to explore means to reduce the manual part of dataset generation while keeping the quality of the annotation at an acceptable level. In this paper, we present a novel approach to creating training datasets for individual building detection and classification from remote sensing imagery consisting of a fully automatic pipeline. Using 3D city models and high-resolution imagery as input, annotations including building footprint and their attributes are automatically generated and combined with the corresponding image segments into a standard dataset complying with the COCO format. Experiments comprising also the comparison to manually labeled datasets demonstrate the potential of the proposed work.
更多查看译文
关键词
Buildings,Object Detection,Classification,Deep learning,Dataset Generation,CityGML
AI 理解论文
溯源树
样例
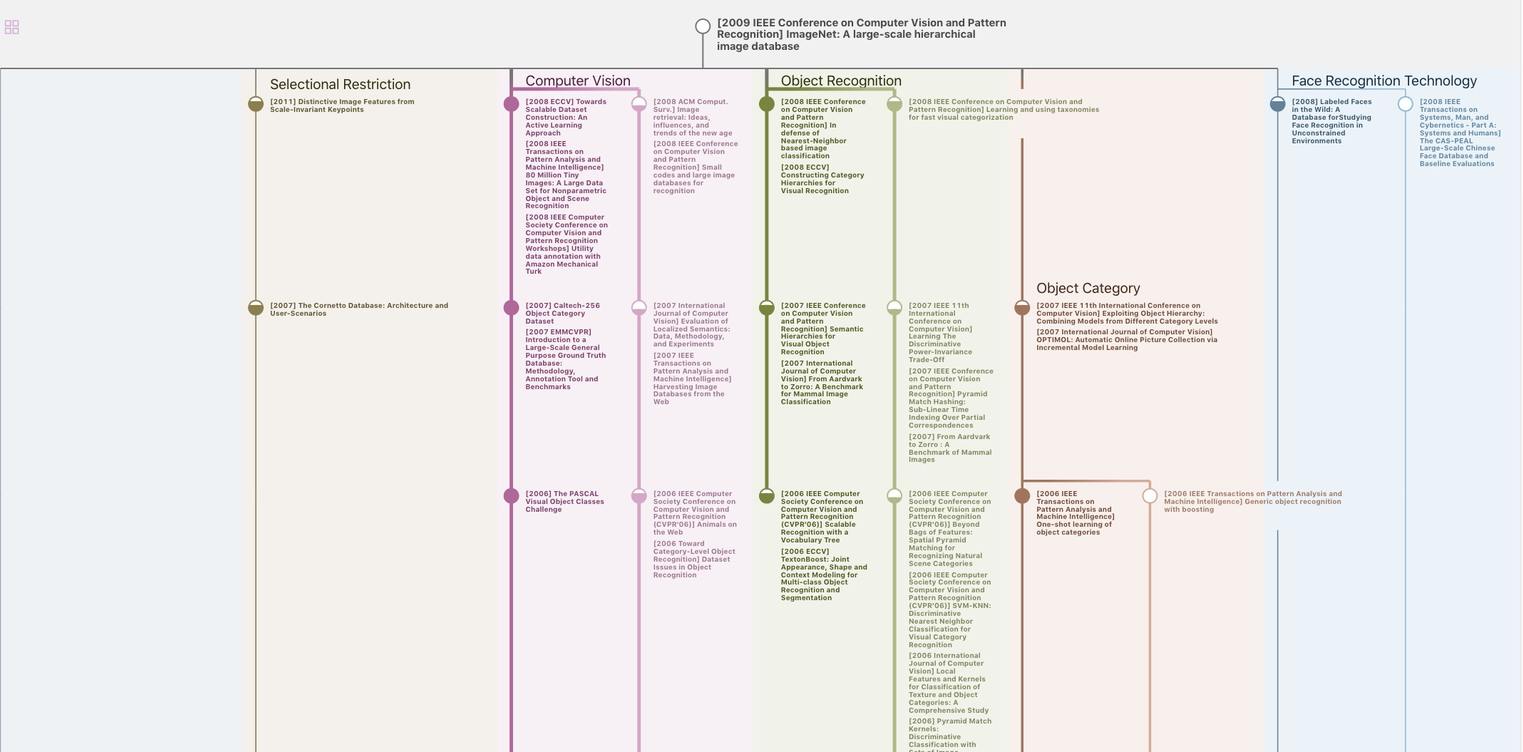
生成溯源树,研究论文发展脉络
Chat Paper
正在生成论文摘要