Gpu-based linear programming: an application to seismic sparse layer inversion
IGARSS 2023 - 2023 IEEE INTERNATIONAL GEOSCIENCE AND REMOTE SENSING SYMPOSIUM(2023)
摘要
Seismic sparse layer inversion (SLI) plays a crucial role in improving the resolution of seismic data for accurate subsurface characterization. However, achieving high accuracy and efficient runtime in SLI is of paramount importance for reservoir characterization. In this paper, we address the SLI problem by constructing a dictionary using odd and even reflection coefficients. The conventional Linear Programming (LP) approach suffers from the equal penalization of all model parameters, resulting in ghost layers and high computational costs on Central Processing Units(CPUs). To overcome these limitations, we propose an enhanced formulation by incorporating Tikhonov regularization, dynamically penalizing the model parameters based on the target seismic trace. Moreover, we optimize the computational runtime by leveraging the power of a Graphical Processing Unit (GPU)-based LP solver. Our algorithm is implemented on an NVIDIA RTX 4000 GPU with 8GB dedicated memory and tested on the Indian WADU dataset. Results demonstrate the efficacy of our proposed method in accurately delineating thin seismic layers compared to state-of-the-art techniques. Additionally, we highlight the superior runtime performance of the GPU-based LP formulation compared to its CPU implementation, further enhancing the efficiency of the SLI process.
更多查看译文
关键词
Sparse layer inversion,Linear programming,Parallel computing,GPU
AI 理解论文
溯源树
样例
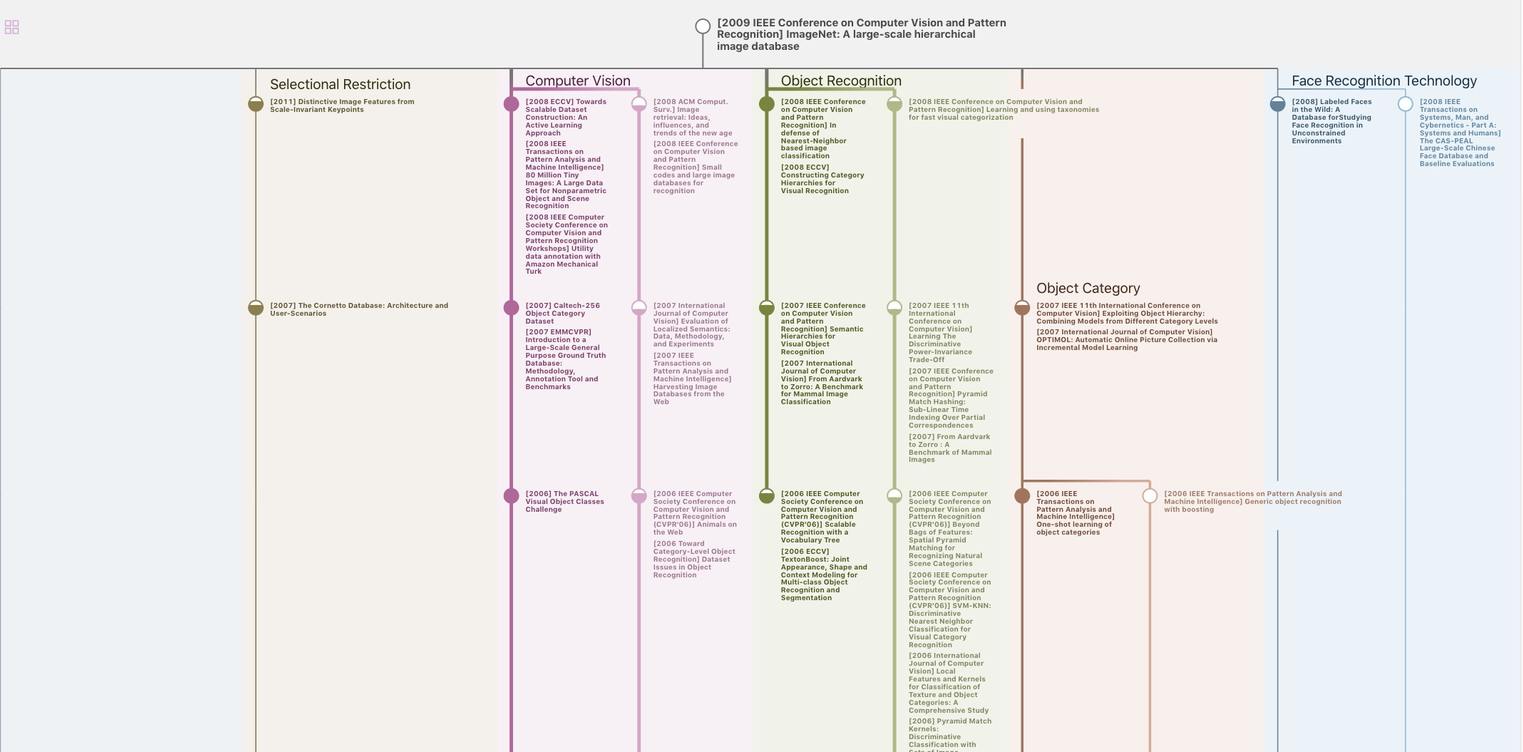
生成溯源树,研究论文发展脉络
Chat Paper
正在生成论文摘要