Improved water chlorophyll-a retrieval method based on mixture density networks using in-situ hyperspectral remote sensing data
IGARSS 2023 - 2023 IEEE INTERNATIONAL GEOSCIENCE AND REMOTE SENSING SYMPOSIUM(2023)
摘要
Continuous monitoring of chlorophyll-a (chl-a), whose shortage or excess leads to detrimental consequences on aquatic ecosystems, provides a better understanding of contamination sources to establish effective measures. Hyperspectral in-situ measurements at German inland waterbodies were used to estimate chl-a remotely. In this vein, Mixture Density Networks (MDNs) were employed, which outperformed other Machine Learning-based Regression (MLR) techniques due to their ability to handle ill-posed problems. Previous studies combined the predicted Gaussian functions of MDNs using maximum likelihood or simple averaging to estimate chl-a, which failed to effectively capture dependencies of multimodal distributions. To address this, Gaussian parameters of MDN were utilized to several Bayesian optimized MLRs, e.g., Support Vector Regressors (SVR), Random Forest (RF), and Gradient Boosting Regressors (GBR). The results revealed that integrating MDN with MLRs improved estimation of chl-a. The combination of MDN+RF showed the best performance with an improvement of 3.02 mu g/L, 4.22%, and 0.054 for RMSE, MAPE, and RMSLE, respectively.
更多查看译文
关键词
Water quality,chlorophyll-a,Hyperspectral,Mixture Density Networks,Remote Sensing
AI 理解论文
溯源树
样例
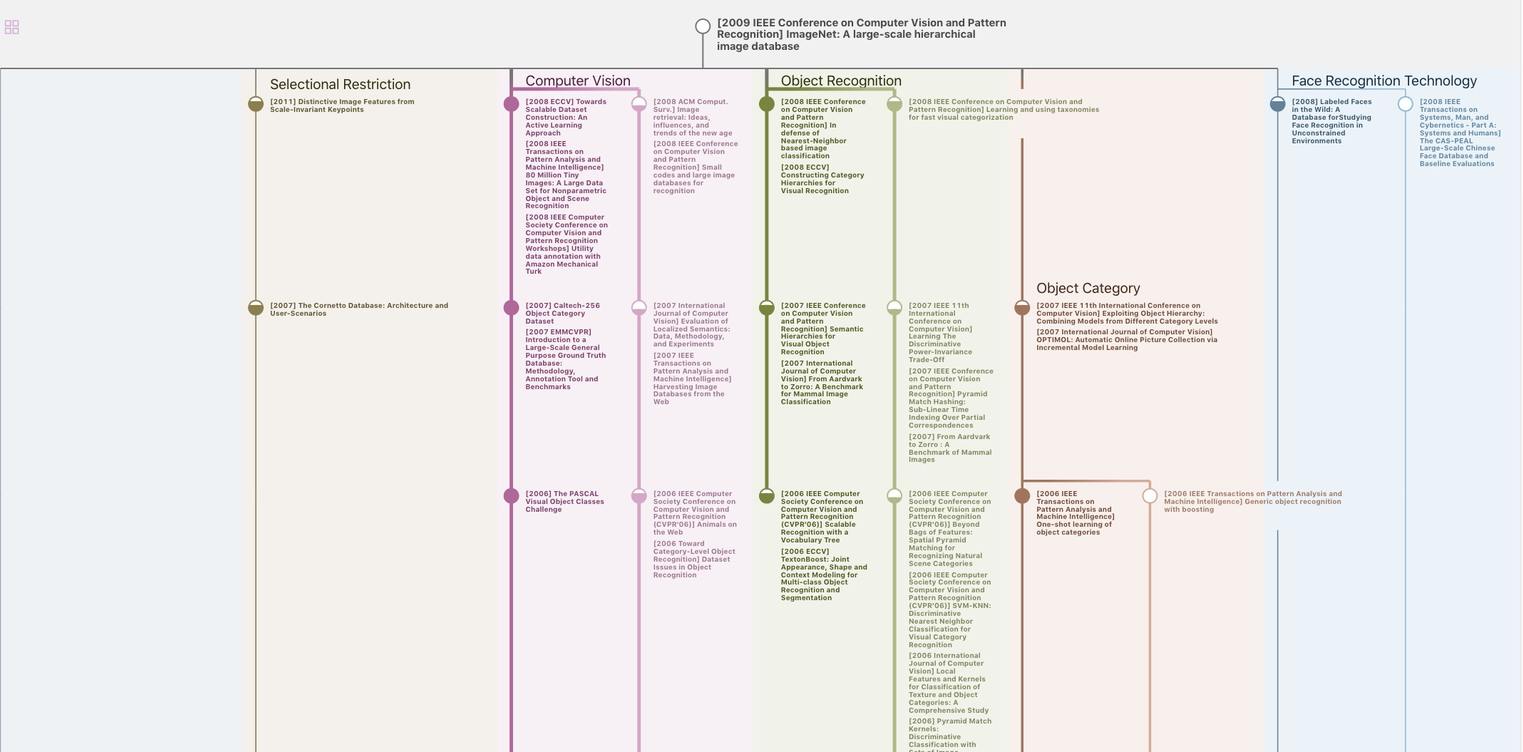
生成溯源树,研究论文发展脉络
Chat Paper
正在生成论文摘要