Comparison of biome-specific ai models to estimate biomass
IGARSS 2023 - 2023 IEEE INTERNATIONAL GEOSCIENCE AND REMOTE SENSING SYMPOSIUM(2023)
摘要
Maintaining and, ultimately, increasing vegetation coverage is likely the most impactful approach to globally capture carbon. Biomass is a crucial parameter for quantifying carbon stored in vegetation, and estimating it poses challenges as statistical models need to be customized to specific biomes. This study compares the prediction of aboveground biomass using various regression methods that were locally fitted in three distinct study sites located in Texas and Louisiana, USA. These sites (biomes) had average aboveground biomass densities of 4.1, 17.3, and 94.6 Mg/ha. The predictions obtained from these localized models were then compared to those derived from a general model that pooled data from all three sites together. Optical and radar imagery acquired from Sentinel satellites were used as predictors, while biomass density from GEDI served as the reference. In most experiments, Random Forest scored best, and the results indicate that the biome-specific models exhibited slightly higher accuracy. Specifically, the root mean square error (RMSE) values for the biome-specific models were 8.8, 16.8, and 54.8 Mg/ha, respectively. In comparison, the general model exhibited approximately 1 Mg/ha higher RMSE. The results indicate that the locally fitted models tailored to specific biomes generally outperformed the general model tested.
更多查看译文
关键词
aboveground biomass,biomass model comparison,machine learning
AI 理解论文
溯源树
样例
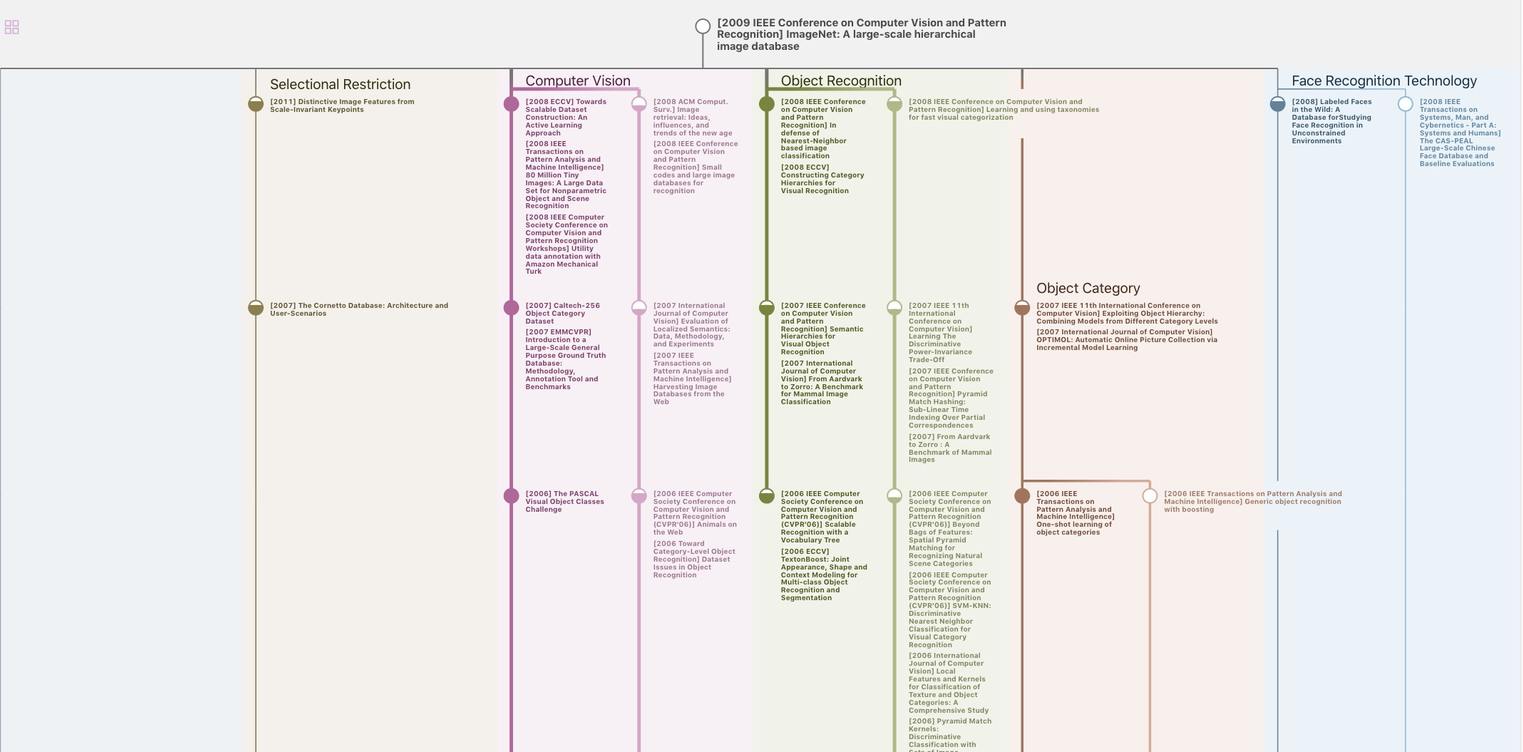
生成溯源树,研究论文发展脉络
Chat Paper
正在生成论文摘要