Above ground carbon biomass estimate with physics-informed deep network
IGARSS 2023 - 2023 IEEE INTERNATIONAL GEOSCIENCE AND REMOTE SENSING SYMPOSIUM(2023)
摘要
Nature-based carbon sequestration solution have the potential to capture carbon dioxide from the atmosphere and store it in vegetation biomass or soil. Forests are covering around 30% of Earth's land surface and combined with forest longevity, trees/soil have the potential to store carbon from decades to centuries. One key challenge is to develop methodologies for high-resolution measurements of carbon sequestered and assess year to year change. Here, we use deep neural network to generate a wall-to-wall map of AGB within the Continental USA (CONUS) with 30-meter spatial resolution for the year 2021. We combine radar and optical multispectral imagery, with a physical climate parameter of Solar Induced Fluorescence (SIF)-based Growth Primary Production (GPP). Validation results show that a masked variation of UNet has the lowest validation RMSE of 37.93 +/- 1.36 Mg C/ha, as compared to 81.95 +/- 0.01 Mg C/ha (linear regressor), 53.37 +/- 0.05 Mg C/ha (gradient boosting), and 52.30 +/- 0.03 Mg C/ha for random forest algorithm. Furthermore, models that learn from SIF-based GPP in addition to radar and optical imagery reduce validation RMSE by almost 10% and the standard deviation by 40%.
更多查看译文
关键词
environmental monitoring,laser radar,geospatial analysis,big data applications,weak supervision
AI 理解论文
溯源树
样例
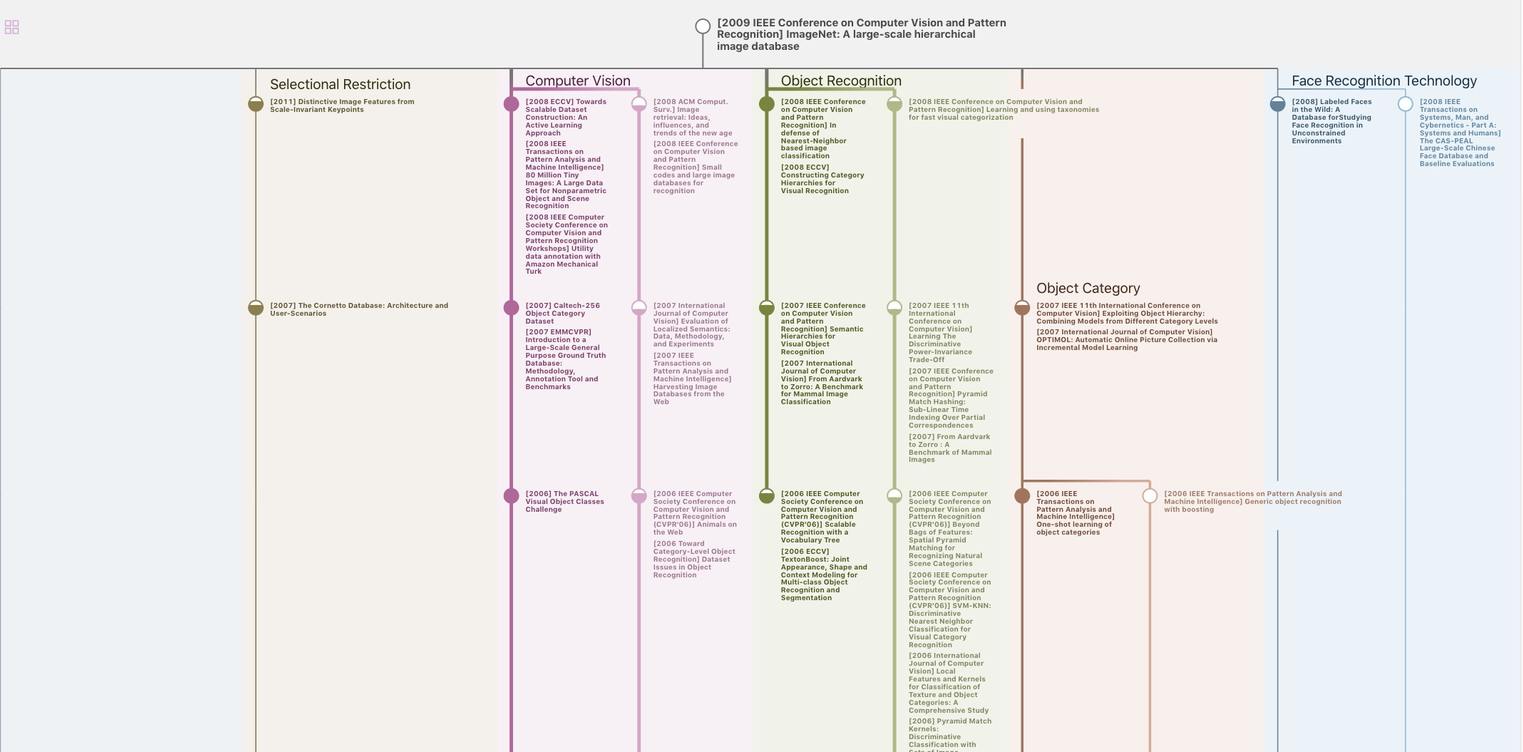
生成溯源树,研究论文发展脉络
Chat Paper
正在生成论文摘要