Preserving extremes in satellite quantitative precipitation estimates: a matter of scale
IGARSS 2023 - 2023 IEEE INTERNATIONAL GEOSCIENCE AND REMOTE SENSING SYMPOSIUM(2023)
摘要
Alternative metrics to the mean squared error (MSE) are assessed as optimization criteria for geophysical remote sensing applications. While the MSE promotes smooth solutions with compressed dynamical range, optimization criteria focusing on the preservation of the statistical properties of the target geospatial fields can be used to compensate this effect. These different optimization criteria are included as distance metrics within the objective function during the training of a deep learning algorithm for retrieval and mapping of precipitation rates from space-borne passive radiometric measurements. It is shown than imposing a constraint on the statistical distribution of the target variable at the "pixel" level only is insufficient, and that a multi-scale constraint (applied here through a discrete wavelet transform) is necessary to preserve the statistical properties of the target and the extremes across all scales.
更多查看译文
关键词
Remote sensing,deep learning,convolutional neural network,optimization,precipitation,satellite,microwave
AI 理解论文
溯源树
样例
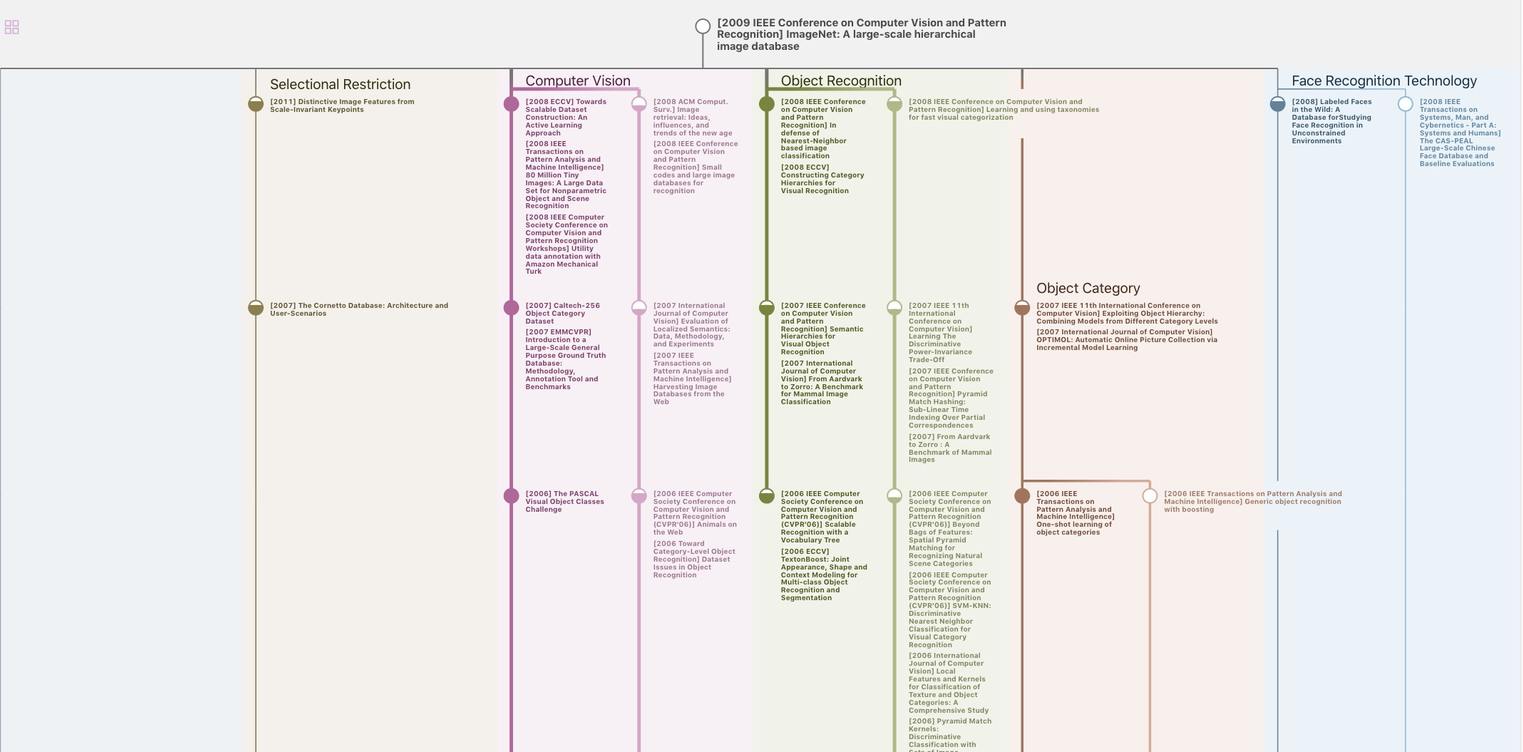
生成溯源树,研究论文发展脉络
Chat Paper
正在生成论文摘要