Home Load Disaggregation using Deep Learning and Bayesian Optimization: A Case Study in Arctic Climate in Northern Norway
2023 IEEE International Conference on Environment and Electrical Engineering and 2023 IEEE Industrial and Commercial Power Systems Europe (EEEIC / I&CPS Europe)(2023)
摘要
Load monitoring is an essential task in energy management systems. In this paper, an approach that relies on a long short-term memory (LSTM) model and a discrete wavelet transform (DWT) filter is presented to estimate the energy usage of flexible appliances. In the preprocessing stage, the main features of the aggregated power signal are extracted using DWT. Deep learning methods are very sensitive to hyperparameters, and choosing optimal values can significantly improve the accuracy of the model. To optimize the performance of the LSTM model, a Bayesian optimization algorithm is used to find the optimal set of hyperparameters. The performance of the proposed approach is evaluated using real-world data collected from a residential building in northern Norway. The results show that the proposed methodology can accurately disaggregate the power consumption of different appliances, with higher accuracy compared to existing methods.
更多查看译文
关键词
Energy disaggregation,Bayesian optimization,deep learning,Non-intrusive load monitoring,Signal processing
AI 理解论文
溯源树
样例
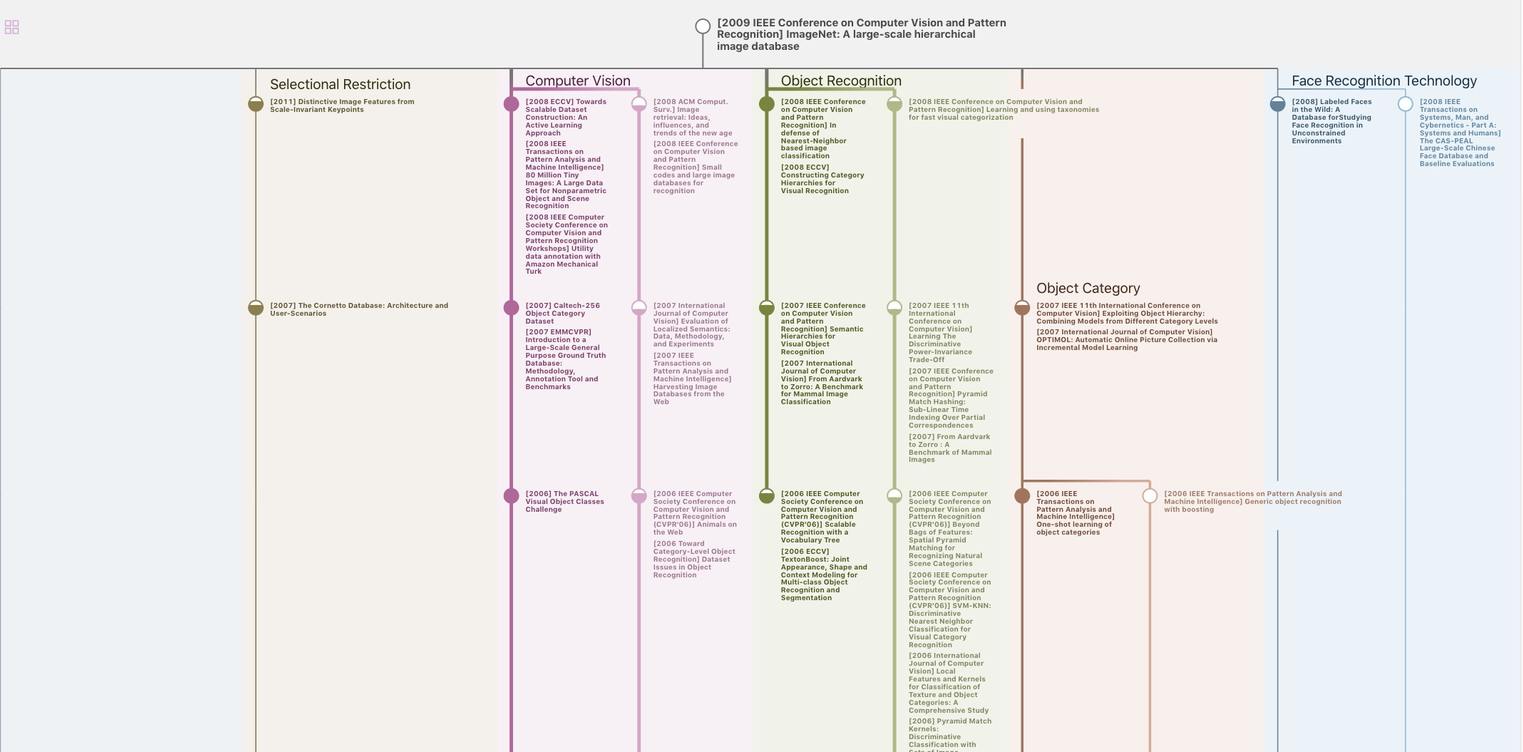
生成溯源树,研究论文发展脉络
Chat Paper
正在生成论文摘要