An LSTM-based Anomaly Classification Framework for Power Electronics Dominated Grids
2023 IEEE Power and Energy Conference at Illinois (PECI)(2023)
摘要
The power electronics dominated grid (PEDG) is a paradigm shift which will see an increase of distributed energy resources (DERs) for power generation. As the PEDG becomes prevalent, grid anomalies must be detected and mitigated. Detection of these systems must be adaptive to changing grid topologies, and thus present an opportunity for data-driven solutions run by local and supervisory controllers in the PEDG. This work presents a line-to-line fault detection scheme built upon the long short-term memory (LSTM) neural network to determine quickly when line-to-line faults occur. The training process and data collection of the proposed line-to-line LSTM based fault detection can be extended to a multi-class classifier for detection of other anomalies in PEDG. The performance of the proposed LSTM based anomaly detection is validated via several case studies. The results demonstrate promising performance for real-time and effective anomaly detection in less than 20 ms to prevent catastrophic event in PEDG.
更多查看译文
关键词
long short-term memory,artificial neural network,corrective action,line-line fault,power electronic dominated grid,distributed energy resources,resiliency,stability
AI 理解论文
溯源树
样例
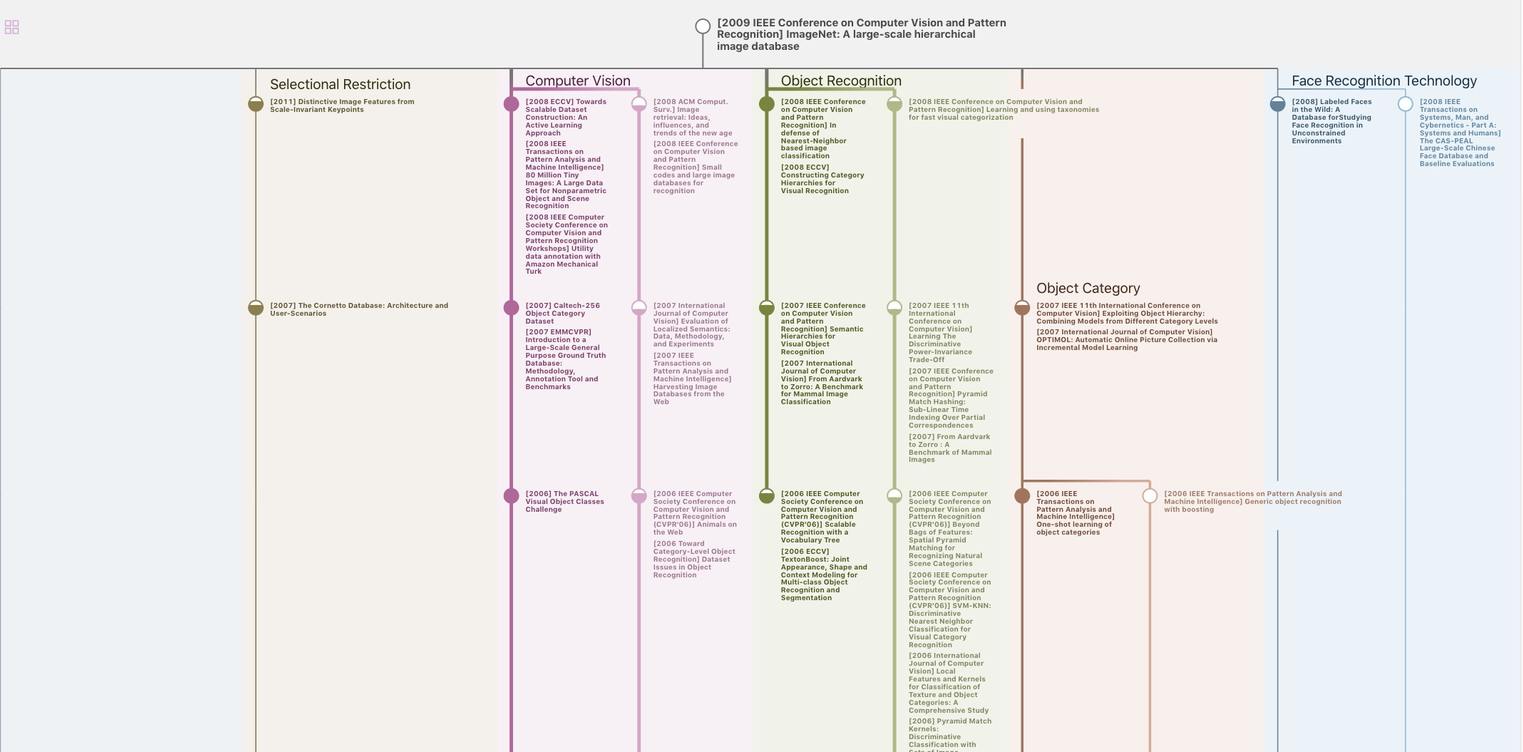
生成溯源树,研究论文发展脉络
Chat Paper
正在生成论文摘要