Enabling Streaming Analytics in Satellite Edge Computing via Timely Evaluation of Big Data Queries
IEEE TRANSACTIONS ON PARALLEL AND DISTRIBUTED SYSTEMS(2024)
摘要
Internet-of-Things (IoT) applications from many industries, such as transportation (maritime, road, rail, air) and fleet management, offshore monitoring, and farming are located in remote areas without cellular connectivity. Such IoT applications continuously generate stream data with hidden values that need to unveiled in real time. Streaming analytics is emerging as a popular type of Big Data analytics to process large volume of stream data for IoT applications in remote regions. Built upon terrestrial-satellite integrated networks, Satellite Edge Computing (SEC) equipped with computing resource in satellites has been envisioning as a key enabling technology to timely analyze stream data of IoT applications in remote regions on the Earth. Considering the dynamically-moving property of satellites in an SEC network, it is vital to optimize the responsiveness of each Big Data analytical query, such that none of such queries takes much longer time to wait for available satellites with sufficient computing resource. Furthermore, the uncertain data volumes of Big Data queries in SEC networks make the resources in satellites fragmented, particularly when the collaboration among satellites is intermittent. Therefore, directly application of existing methods may not guarantee the timelineness of streaming analytics in an SEC network. To address the afore-mentioned unique challenges of streaming analytics in SEC networks, it is urgent to design new algorithms and methods for timely Big Data processing. Specifically, we use the flow time to capture the responsiveness of streaming analytics in satellite edge computing, which is the time between the generation of the first unit of a dataset and the finish time of the data processing. We consider the flow time minimization problem for query evaluation of Big Data analytics in an SEC network with the aim of minimizing the average flow time of Big Data analytical queries, under an assumption of uncertain volumes of datasets. To this end, we first propose an approximation algorithm with a provable approximation ratio for the offline version of the flow time minimization problem. We then devise an online learning algorithm, referred to the customized Lipschitz bandit learning algorithm, with a bounded regret for the online version of the problem. We finally evaluate the performance of the proposed algorithms in a real SEC network topology. Experiment results show that the performance of the proposed algorithm outperforms its counterparts by at least 13% in terms of flow time.
更多查看译文
关键词
Satellite edge computing,Big Data analytics,query evaluation,online learning,approximation algorithms
AI 理解论文
溯源树
样例
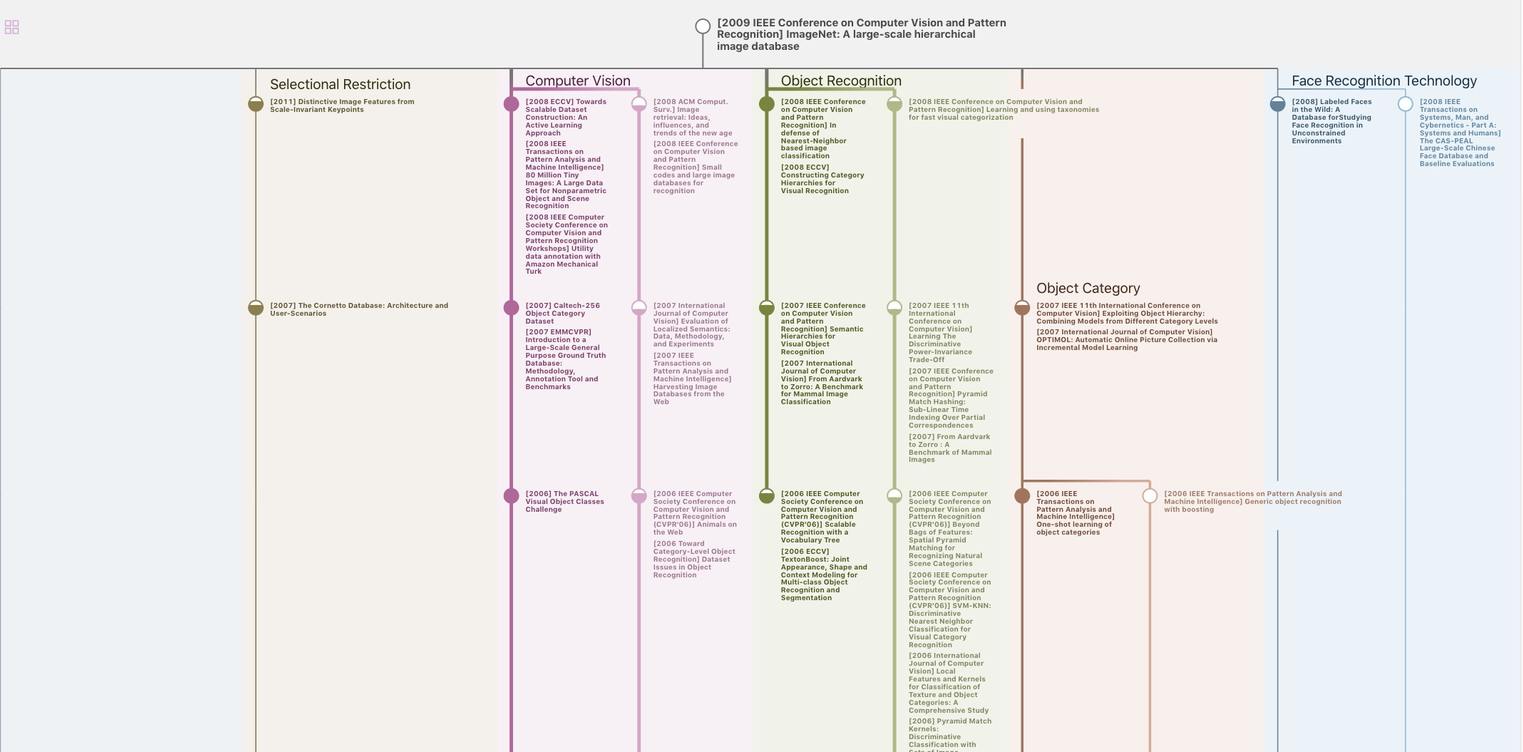
生成溯源树,研究论文发展脉络
Chat Paper
正在生成论文摘要