MUBT: An Effective Deep Learning Method for Nidus Segmentation of Brain Tumor MRI Scans
2023 2nd International Conference on Artificial Intelligence and Computer Information Technology (AICIT)(2023)
摘要
Magnetic Resonance imaging (MRI) serves as an effective tool for detecting intracranial tumors. Traditionally, when brain tumor scans undergo manual clinical nidus segmentation, the daunting task costs a clinician excessive amount of time and energy, often resulting in poor consistency. This paper exploits and modifies a deep learning model to automate this process. Our novel Modified U-Net for Brain Tumor Segmentation (MUBT) is an effective convolutional neural network that segments nidus areas from brain tumor MRI images. We apply our model to the Multimodal Brain Tumor Image Segmentation Benchmark (BRATS) 2020, which is well established for this type of benchmarking. Our measures of performance include val-loss, dice-loss, and dice score. From our validation experiments, MUBT achieves a dice score of 93.1%, far surpassing existing models. Moreover, we demonstrate the optimizing techniques and training process with significantly more detailed information than other relevant research, making it possible to further optimize the model upon reproduction.
更多查看译文
关键词
Brain Tumor,Deep Learning,Image Segmentation
AI 理解论文
溯源树
样例
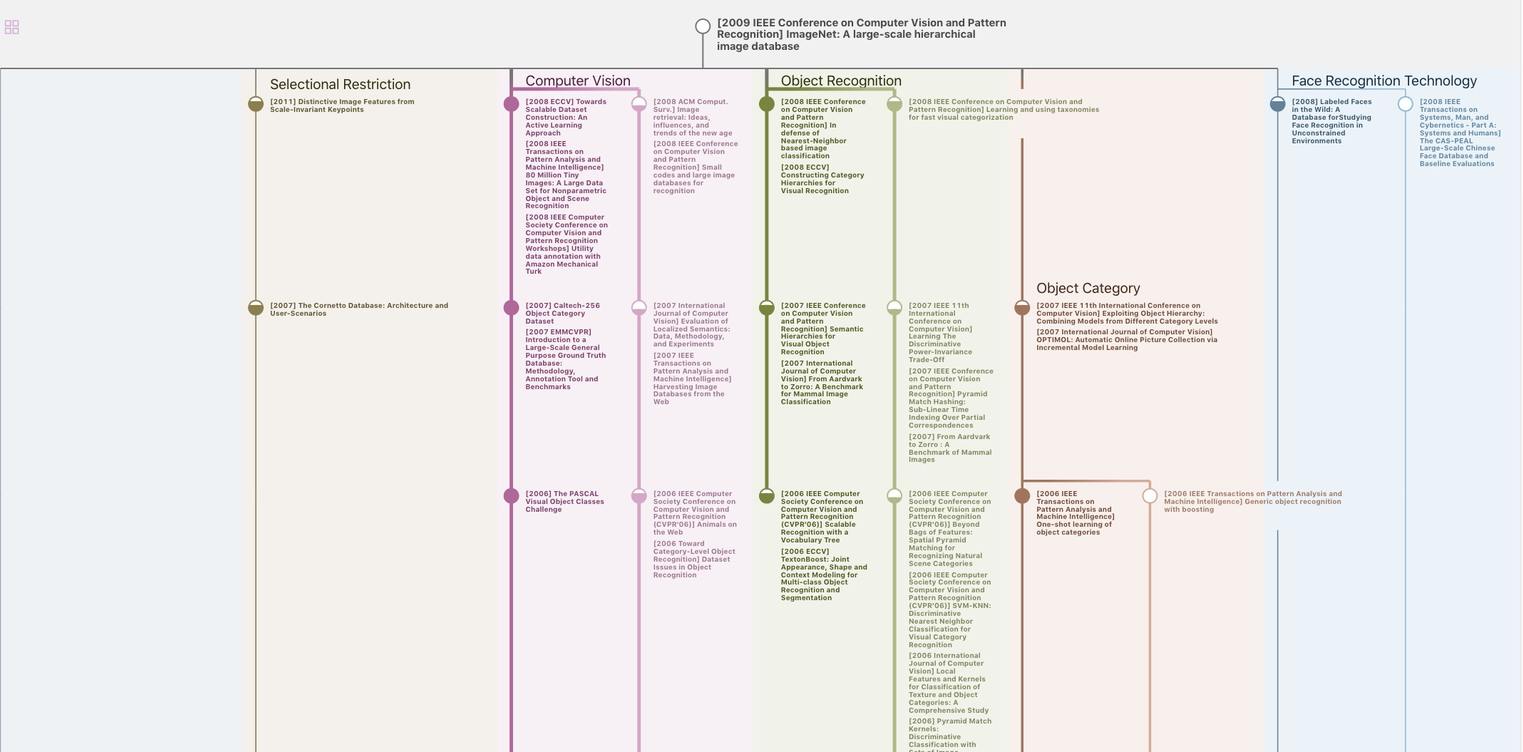
生成溯源树,研究论文发展脉络
Chat Paper
正在生成论文摘要