RacPixGAN: An Enhanced Sketch-to-Face Synthesis GAN Based on Residual modules, Multi-Head Self-Attention Mechanisms, and CLIP Loss
2023 4th International Conference on Electronic Communication and Artificial Intelligence (ICECAI)(2023)
摘要
In this paper, we present an enhanced model to overcome the drawbacks of the traditional Pix2pix GAN (Image-to-Image Translation with Conditional Adversarial Networks) in generating performance for sketch-to-face synthesis. This model integrates residual modules and multi-head self-attention mechanisms. Additionally, to enhance the model’s generative capabilities in sketch-to-face synthesis tasks, we introduce a brand-new loss function called CLIP (Contrastive Language-Image Pretraining) Loss. We begin by providing a comprehensive overview of the key theories and techniques for our model. Then, we empirically test the upgraded model and contrast it with the traditional Pix2pix GAN. The experimental outcomes demonstrate that the new model significantly outperforms the traditional Pix2pix GAN in terms of generating performance for sketch-to-face synthesis tasks, supporting the idea that adding residual modules and multi-head self-attention mechanisms can significantly improve the generator’s performance in such tasks. The addition of CLIP Loss has also been shown to improve the quality of image generation.
更多查看译文
关键词
Generative Adversarial Networks (GANs),CLIP Loss,Residual Modules,Multi-Head Self-Attention Mechanisms,Sketch-to-Face Synthesis
AI 理解论文
溯源树
样例
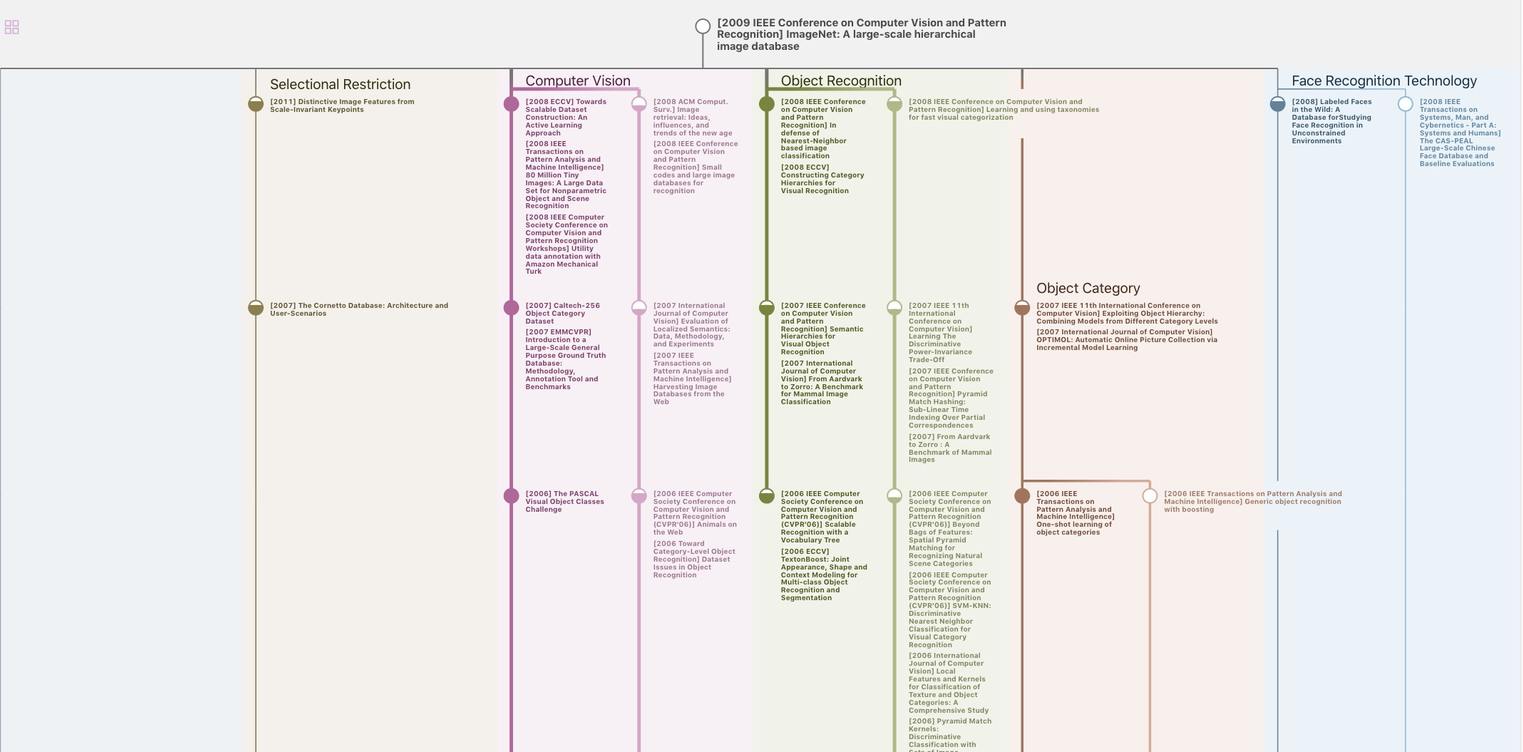
生成溯源树,研究论文发展脉络
Chat Paper
正在生成论文摘要