A U-Net Based Architecture for Automatic Music Transcription
2023 IEEE 33rd International Workshop on Machine Learning for Signal Processing (MLSP)(2023)
摘要
Automatic Music Transcription (AMT) is a complex matter involving several researchers. Recently, due to the powerful Deep Learning techniques, many effective solutions have been proposed. However, there is still room for improvement. To this purpose, in this paper, we propose an architecture based on two U-Net models exploiting Convolutional Neural Networks (CNNs) and a Bidirectional Long-Short Term Memory (BiLSTM) unit, aiming at improving the wave to MIDI transcription performance. This couple of U-Nets act as onset and offset detectors, respectively, whose information are jointly used along with the input mel spectrogram into a third model to find all the active notes in each time-frame. Some numerical results, obtained on the well known MAPS dataset, show the effectiveness of the proposed idea and the advantages over similar state-of-the-art approaches.
更多查看译文
关键词
Automatic Music Transcription (AMT),Wave to MIDI,Deep Learning,U-Net,Convolutional Neural Network (CNN)
AI 理解论文
溯源树
样例
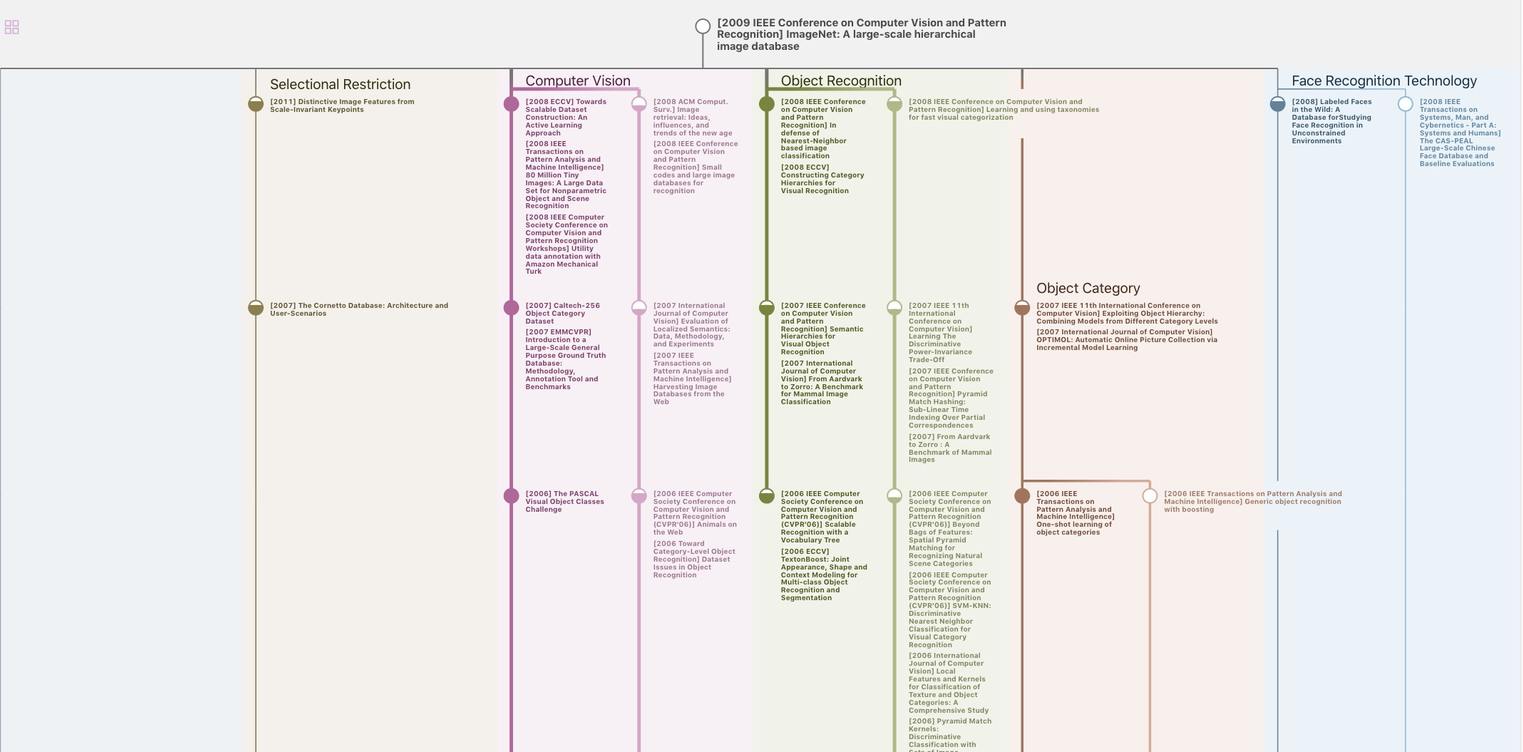
生成溯源树,研究论文发展脉络
Chat Paper
正在生成论文摘要