Privacy-Preserving Federated Primal-Dual Learning for Non-Convex Problems With Non-Smooth Regularization
2023 IEEE 33rd International Workshop on Machine Learning for Signal Processing (MLSP)(2023)
摘要
Recently, the federated learning (FL) has been a machine learning paradigm for the preservation of data privacy, though high communication cost and privacy protection are still the main concerns of FL. However, in many practical applications, the trained model needs certain nature or characteristics, such as sparseness in classification, otherwise learning performance loss is inevitable. In order to upgrade the learning performance, a suitable non-smooth regularizer (e.g., $\ell_{1}$-norm for the model sparseness) can be added to the loss function (often non-convex) in the considered optimization problem. This paper proposes a novel primal-dual learning algorithm to handle such non-smooth regularization aided non-convex FL problems, that yields much superior learning performance over some state-of-the-art FL algorithms under privacy guarantee by means of differential privacy. Finally, some experimental results are provided to demonstrate the efficacy of the proposed algorithm.
更多查看译文
关键词
Federated learning,primal-dual method,non-convex and non-smooth optimization,differential privacy
AI 理解论文
溯源树
样例
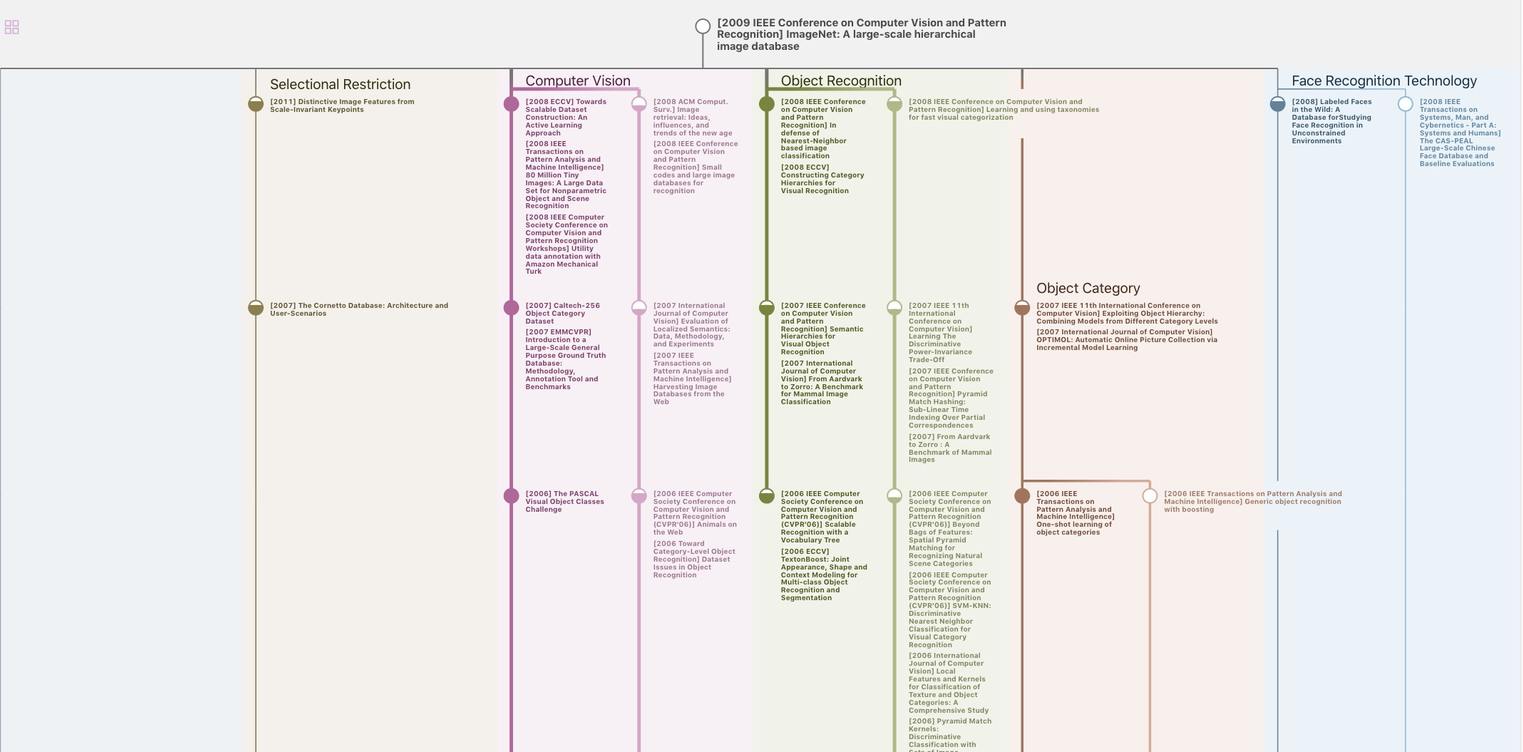
生成溯源树,研究论文发展脉络
Chat Paper
正在生成论文摘要