Trainable Policy For The On-Demand Offloading On Edge-Cloud Collaborative System
2023 IEEE 33rd International Workshop on Machine Learning for Signal Processing (MLSP)(2023)
摘要
An on-demand offloading framework is a practical solution for resource-limited Internet of Things scenarios. However, an ineffective offloading policy can lead to wasteful transmission costs. Prior works have designed policies based solely on edge information, neglecting the role of the cloud and potentially degrading overall performance. In this paper, we propose two methods to address this issue. First, we modify the training process to incorporate information from both the edge and the cloud, achieving joint edge-cloud optimization in our trainable offloading policy. Second, we leverage structured feature representations to enhance our policy’s efficiency and reduce the cost of ineffective offloading. Our experimental results show that our methods outperform existing approaches on ResNet152 and VGG16, reducing the offloading ratio by 17.74%/23.57% and increasing the offloading efficiency by 4.47%/5.52%, respectively.
更多查看译文
关键词
Collaborative intelligence,model confidence,computation offloading,metric learning,deep learning.
AI 理解论文
溯源树
样例
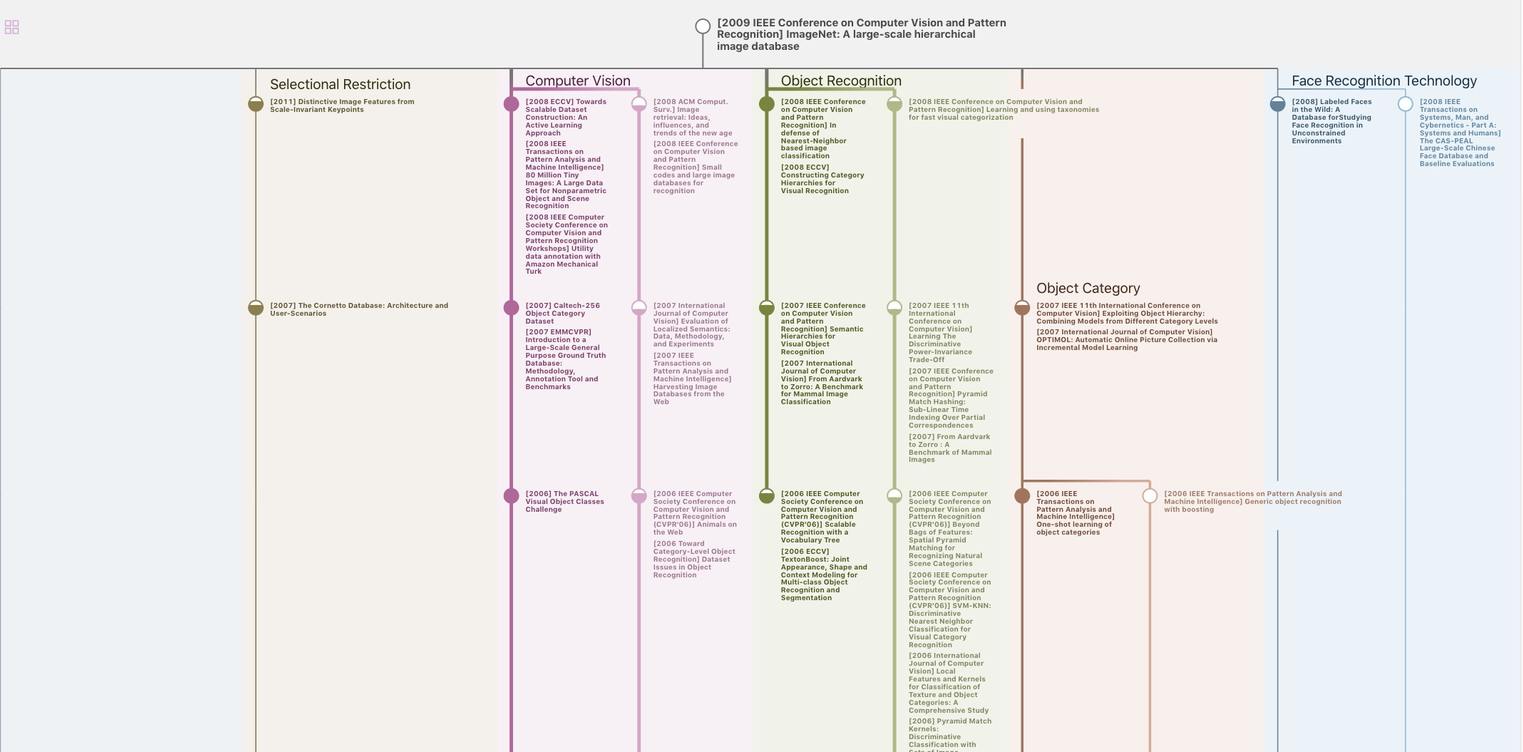
生成溯源树,研究论文发展脉络
Chat Paper
正在生成论文摘要