ECG Signals Analysis based on Compressed Sensing and Learning Techniques for Heart Disease Recognition
2023 7th International Multi-Topic ICT Conference (IMTIC)(2023)
摘要
Advancements in the acquisition and processing of physiological signals have facilitated deeper analysis aimed at disease identification and diagnosis. However, with the significant rise in data size and power consumption, it is necessary to use effective data compression, signal acquisition, transmission, and processing techniques, especially in tele-health and mobile-Health applications. A new area of research involves integrating Compressed Sensing (CS) and Compressed Learning (CL) with an electrocardiogram (ECG) signals to overcome such issues. This research paper investigates the use of CS and CL techniques on ECG signals from three distinct groups of individuals: those with normal sinus rhythms (NSR), congestive heart failure (CHF), and cardiac arrhythmia (ARR). The study employs three measurement matrices, Random Gaussian Matrix (RGM), Random Bernoulli Matrix (RBM), and Structured Fourier Matrix (SFM) with the Block Sparse Bayesian Learning reconstruction algorithm. The features extracted from the compressed signal and reconstructed signals using Long Short-Term Memory (LSTM) and Support Vector Machine (SVM) algorithms in both compressed sensing and compressed learning. The results show that the LSTM and SVM algorithms achieve high accuracy in both training and testing phases, with LSTM performing better in CL with SFM, while both SVM and LSTM perform better in SC with RGM and RBM. This study demonstrates the potential of CS and CL techniques in extracting relevant features from ECG signals, which could have implications for diagnosing and treating cardiac conditions.
更多查看译文
关键词
Compressed Sensing,Compressed Learning,Physiological Signals,ECG,Features extraction,Healthcare,Big Data,Wearable Devices
AI 理解论文
溯源树
样例
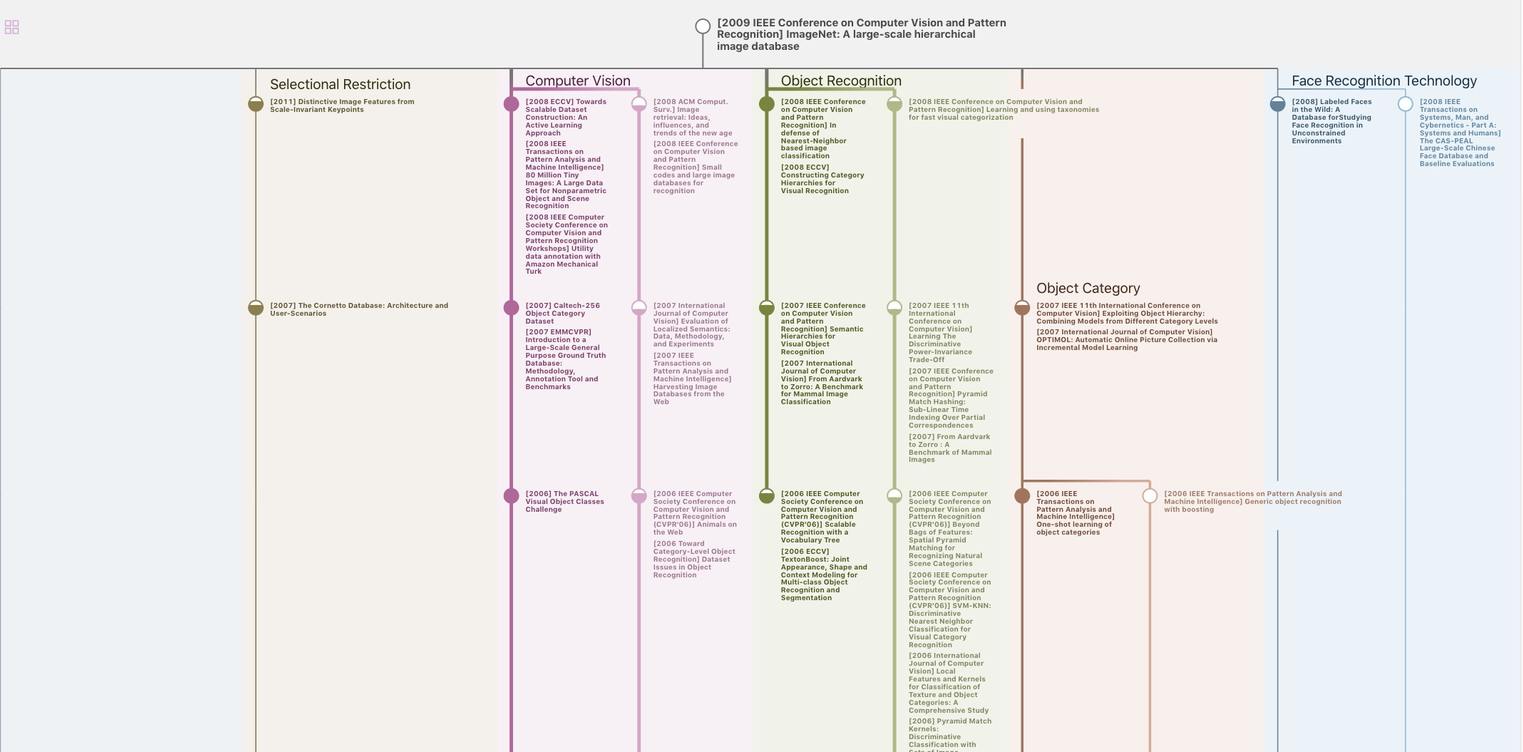
生成溯源树,研究论文发展脉络
Chat Paper
正在生成论文摘要