Multi-Agent Reinforcement Learning with Epistemic Priors
2023 9th International Conference on Control, Decision and Information Technologies (CoDIT)(2023)
摘要
It is important for autonomous multi-agent teams to coordinate actions so that collaborative goals can be achieved efficiently without conflicts. Practical issues in multi-agent, real-time systems are limited sensing and communication capabilities. A significant number of multi-agent algorithms rely on accurate state information for all agents in order to effectively coordinate. In this paper, we propose an approach called Reinforcement Learning with Epistemic Priors (MARL-EP). MARL-EP incorporates a shared mental model between agents by leveraging epistemic estimation to infer portions of the obser-vation space which are unobservable. We show that MARL-EP allows a very high level of coordination to be achieved with severely impaired sensing and zero communication between agents.
更多查看译文
关键词
accurate state information,autonomous multiagent teams,collaborative goals,communication capabilities,epistemic estimation,MARL-EP,multiagent algorithms,multiagent reinforcement learning,real-time systems,reinforcement learning with epistemic priors,shared mental model
AI 理解论文
溯源树
样例
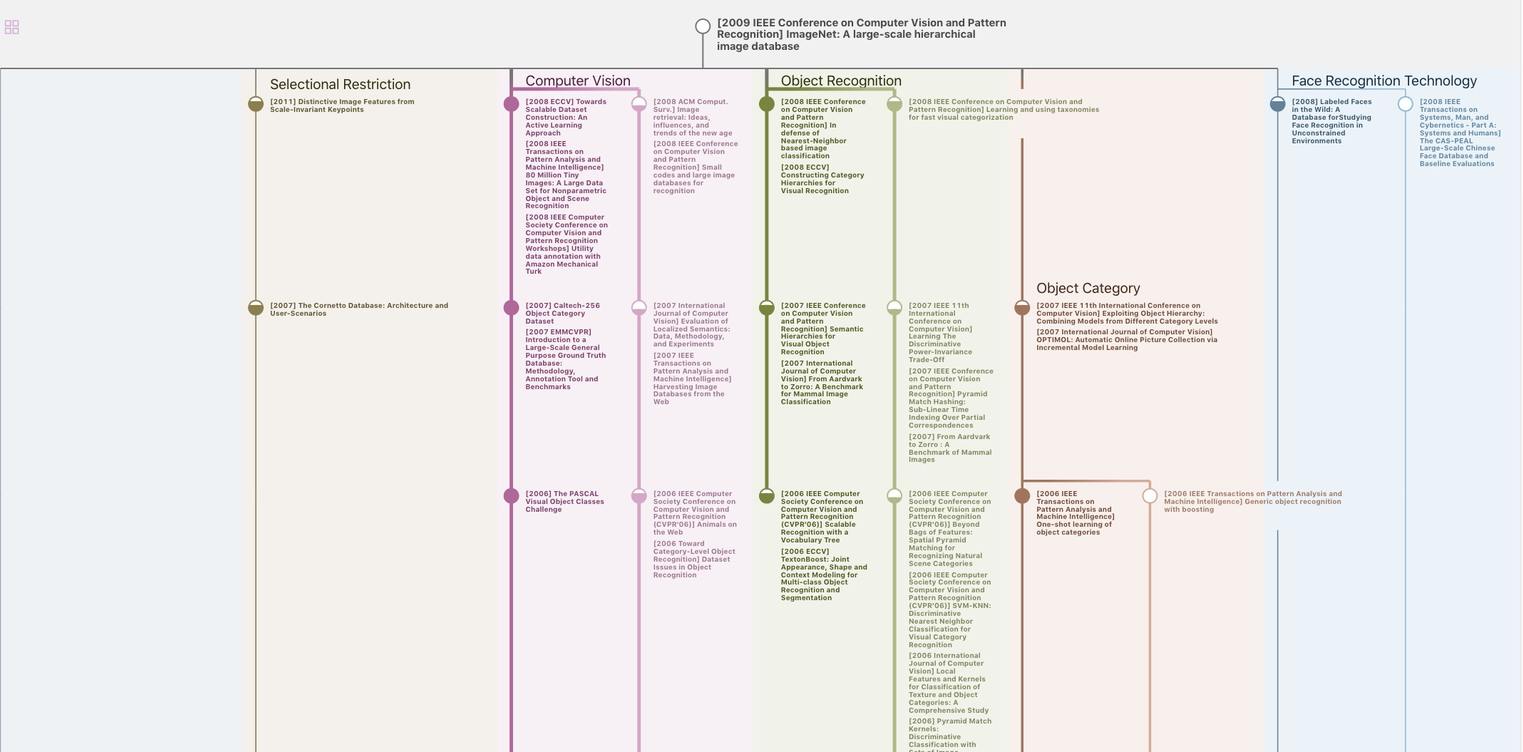
生成溯源树,研究论文发展脉络
Chat Paper
正在生成论文摘要