A temporal Human Activity Recognition Based on Stacked Auto Encoder and Extreme Learning Machine
2023 9th International Conference on Control, Decision and Information Technologies (CoDIT)(2023)
摘要
Human Activity Recognition (HAR) is one of the most important research areas in the fields of health and human-machine interaction. The creation of several artificial intelligence-based models for activity identification has resulted in poor long-term performance in the actual world since these methods are unable to extract spatial and temporal information. Though, Deep learning is starting to replace well-established hand-crafted techniques that rely on expertly built feature extraction and classification techniques in the field of HAR. Nevertheless, it is challenging to acquire an overview of the suitability of these discrete implementations of custom deep architectures for issues ranging from the recognition of manipulative gestures to the identification and segmentation of physical activities. Given these constraints, we develop an innovative Stacked Auto Encoder (SAE) and Extreme Learning Machine (ELM) architecture based on temporal feature to produce a new model for HAR.. This feature is connected to speed of movement and fed to a new neural network architecture as temporal stream. A key step in this approach is the selection of features that characterize the complexity of human actions in time. Results show that the proposed model outperforms many of the existing deep and machine learning techniques.
更多查看译文
关键词
activity identification,artificial intelligence-based models,classification techniques,custom deep architectures,deep machine learning techniques,ELM architecture,expertly built feature extraction,extreme learning machine architecture,hand-crafted techniques,HAR,human actions,human-machine interaction,innovative stacked autoencoder,machine learning techniques,neural network architecture,physical activities,SAE,segmentation,spatial information,temporal feature,temporal human activity recognition,temporal information,temporal stream
AI 理解论文
溯源树
样例
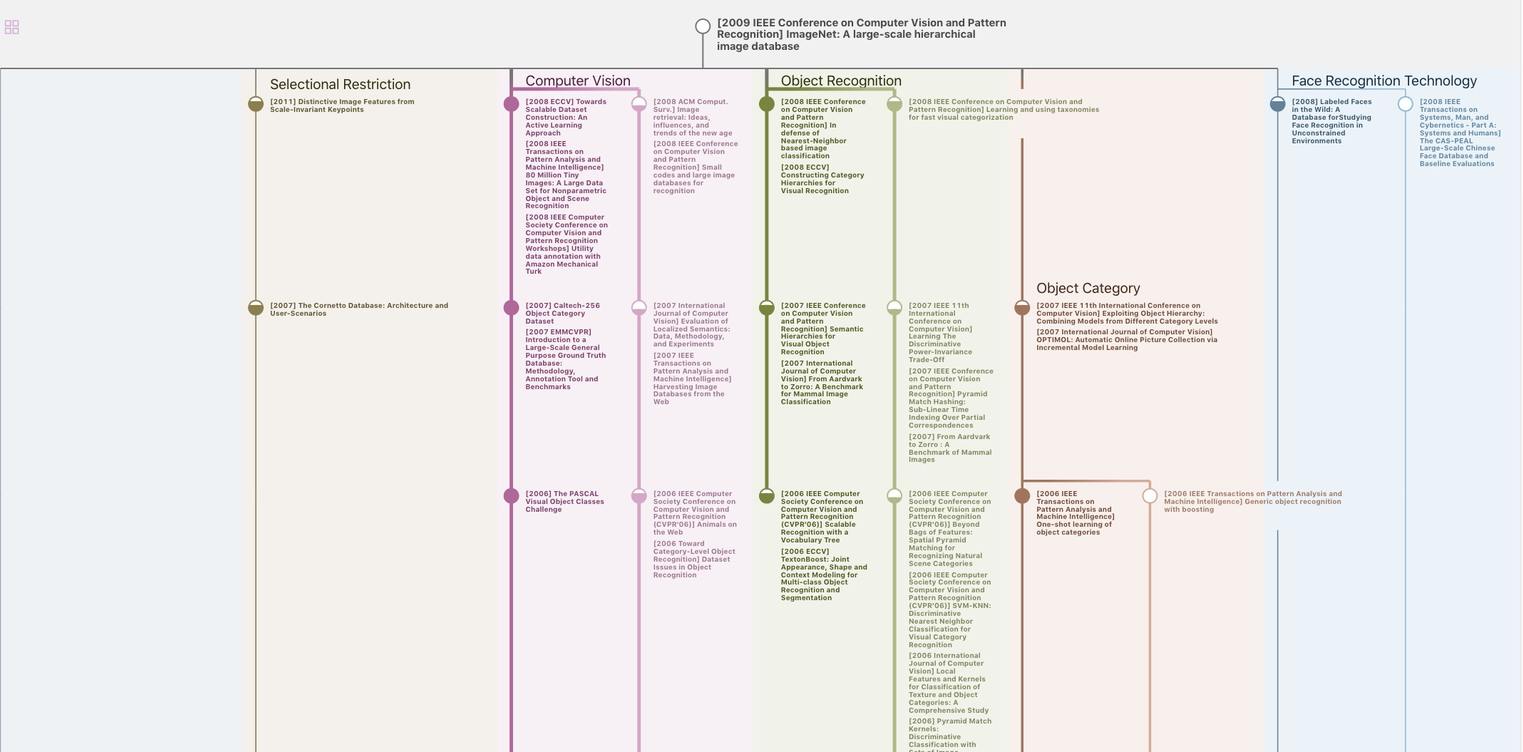
生成溯源树,研究论文发展脉络
Chat Paper
正在生成论文摘要