Self-Attention blocks in UNet and FCN for accurate semantic segmentation of difficult object classes in autonomous driving
2023 IEEE CANADIAN CONFERENCE ON ELECTRICAL AND COMPUTER ENGINEERING, CCECE(2023)
摘要
Deep learning has been widely used in computer vision applications and one of the recent breakthroughs in this field is the use of attention modules. Present models, to the best of our knowledge, are not accurate enough in terms of distinguishing difficult object classes like pedestrians and bicycles in street scenes. In this paper, we proposed the use of self-attention blocks in the encoder section of UNet and FCN with the aim of improving the performance of the models in segmenting difficult object classes. The proposed SA-UNet and SA-FCN models excel in detecting critical object classes, providing better insights into street scenes, and improving the safety of pedestrians and drivers in autonomous driving systems. We tested our proposed models on the Cityscape Dataset, and the experimental results show that our proposed models improved the IoU score by 0.1 in FCN-32 when self-attention was deployed. Similarly, in UNet, the IoU was improved by 5 percent with the attention block. Also, the visual representation of the output images demonstrates how the selfattention block in the encoder of the model can improve accuracy in detecting occluded yet important classes like Pedestrian.
更多查看译文
关键词
Semantic Segmentation,Self-Attention,UNet,Fully Convolutional Network,Accurate Pedestrian Segmentation
AI 理解论文
溯源树
样例
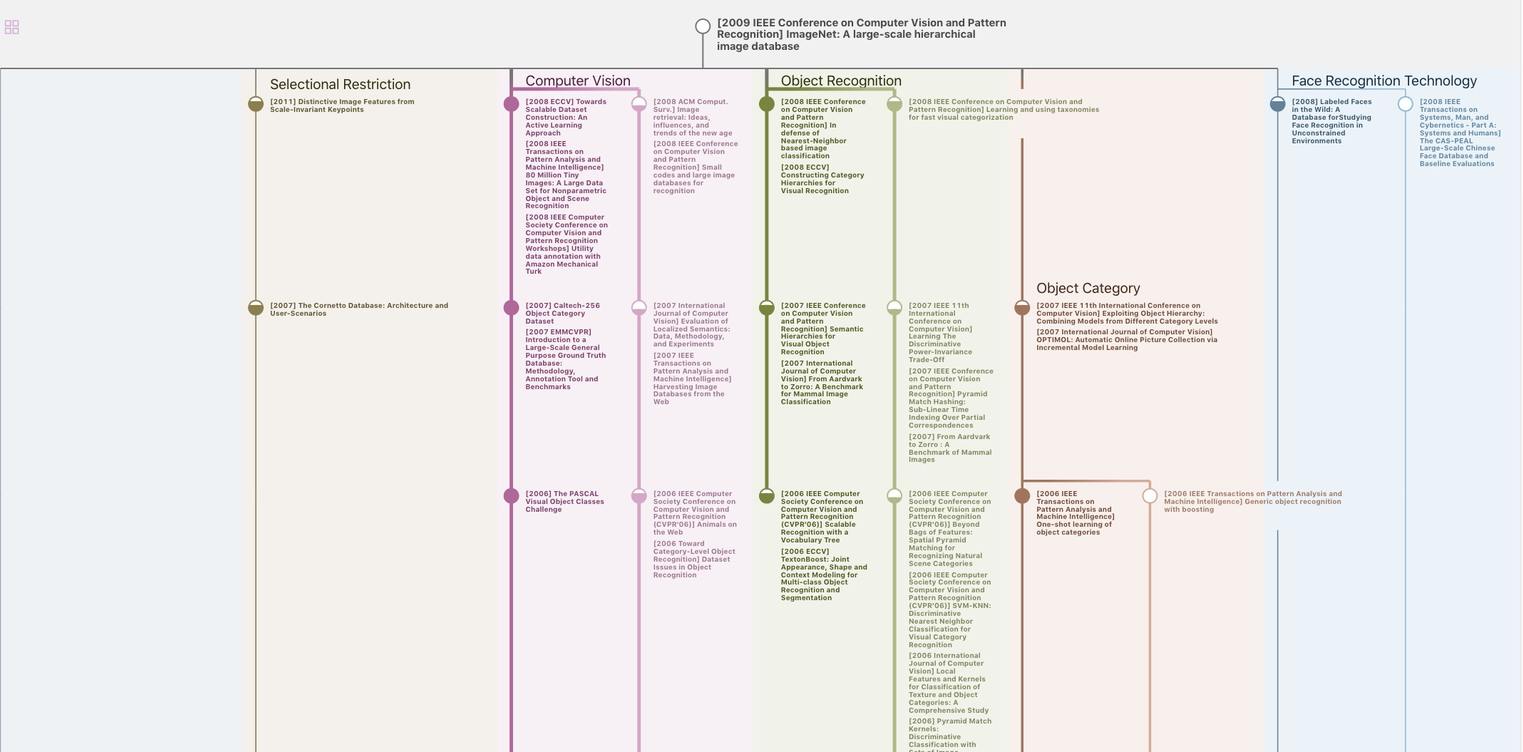
生成溯源树,研究论文发展脉络
Chat Paper
正在生成论文摘要