Generating adversarial examples with collaborative generative models
International Journal of Information Security(2024)
摘要
Deep learning has made remarkable progress, and deep learning models have been successfully deployed in many practical applications. However, recent studies indicate that deep learning models are vulnerable to adversarial examples generated by adding an imperceptible perturbation. The study of adversarial attacks and defense has attracted substantial interest from researchers due to its high application value. In this paper, a method named AdvAE-GAN is proposed for generating adversarial examples. The proposed method combines (1) explicit perturbation generated by adversarial autoencoder and (2) implicit perturbation generated by generative adversarial network. A more suitable similarity measurement criteria is incorporated into the model to ensure that the generated examples are imperceptible. The proposed model not only is suitable for white-box attacks, but also can be adapted to black-box attacks. Extensive experiments and comparisons with six state-of-the-art methods (FGSM, SDM-FGSM, PGD, MIM, AdvGAN, and AdvGAN++) demonstrate that the adversarial examples generated by AdvAE-GAN result in high attack success rate with good transferability and are more realistic-looking and natural. Our code is available at https://github.com/xzforeverlove/Generating-Adversarial-Examples .
更多查看译文
关键词
Adversarial attack,Adversarial defense,Adversarial examples,Perturbations,Collaborative learning
AI 理解论文
溯源树
样例
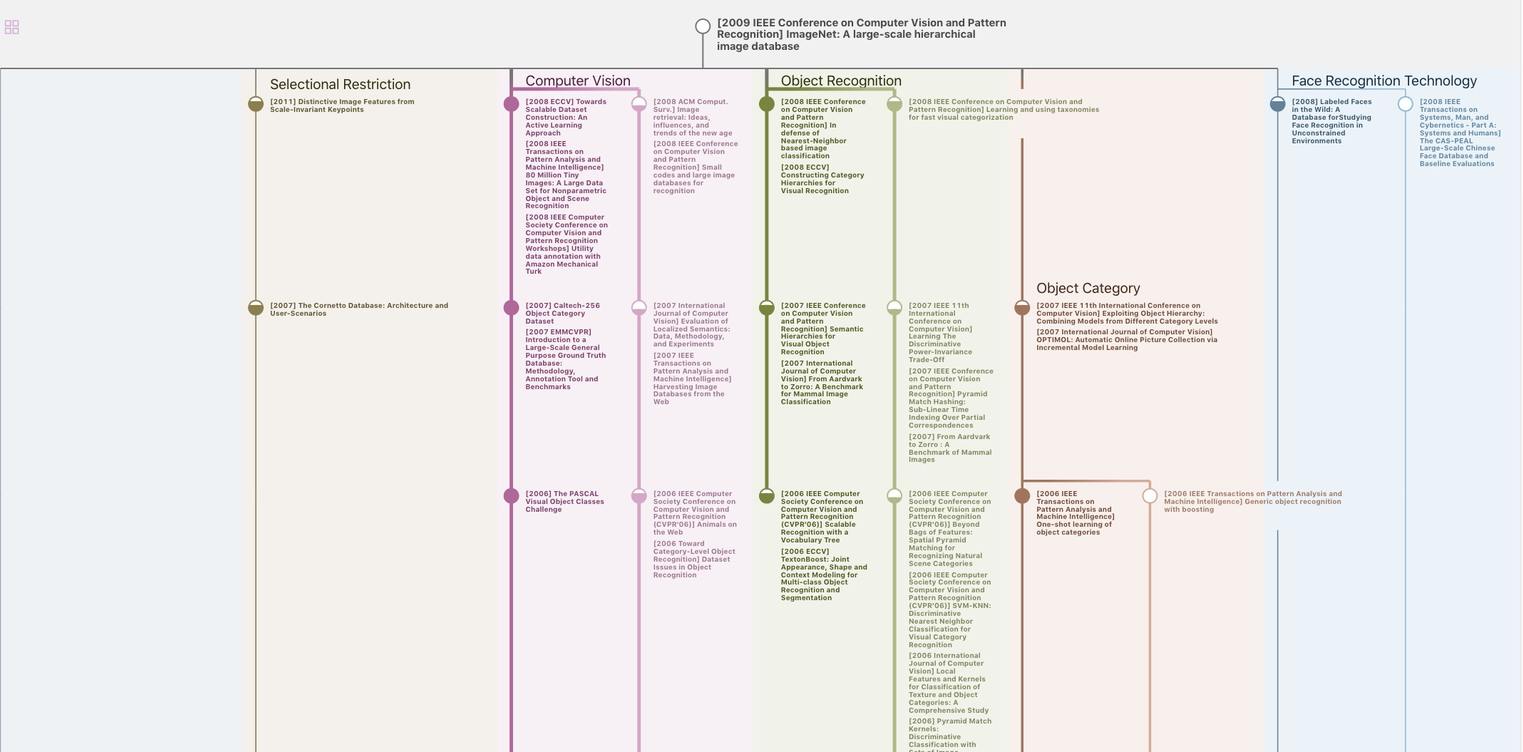
生成溯源树,研究论文发展脉络
Chat Paper
正在生成论文摘要