ABLAL: Adaptive Background Latent Space Adversarial Learning Algorithm for Hyperspectral Target Detection
IEEE JOURNAL OF SELECTED TOPICS IN APPLIED EARTH OBSERVATIONS AND REMOTE SENSING(2024)
摘要
Hyperspectral images (HSIs) are challenging for hyperspectral object detection (HTD) due to their complex background information and the limited prior knowledge of the target. This article proposes an adaptive background latent space adversarial learning algorithm for hyperspectral target detection (ABLAL). We begin by using a coarse screening method to select pseudobackground and pseudotarget sample sets, addressing the issues caused by insufficient prior target information and complicated background information, which result in low detection accuracy. Next, we utilize an Adversarial Autoencoder (AAE) based backbone network to extract the background latent spatial information of the HSI. It should be noted that we adaptively constrain the accuracy of the extracted information through the pseudotarget dataset, accounting for the impact of potential targets in the pseudobackground dataset. Furthermore, we fully utilize the information extracted by AAE and employ a strategy combining multiple output results of AAE. Specifically, we use the distance between the target latent space vector and the background latent space vector, and the HSI reconstruction difference to suppress the background. Finally, extensive experiments are conducted on real datasets to demonstrate the effectiveness of the proposed method.
更多查看译文
关键词
Adversarial learning,hyperspectral image (HSI),latent space,target detection,weak supervised learning
AI 理解论文
溯源树
样例
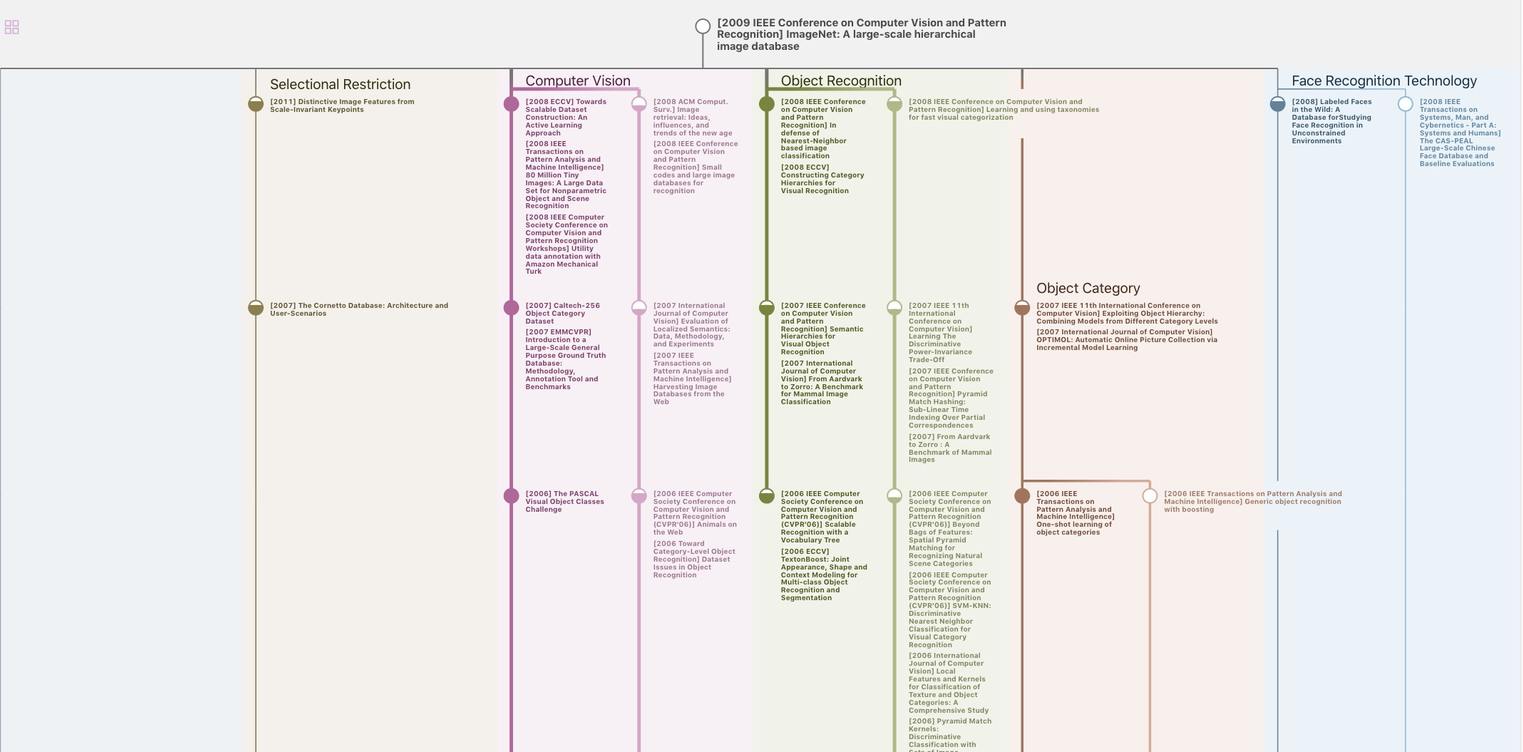
生成溯源树,研究论文发展脉络
Chat Paper
正在生成论文摘要